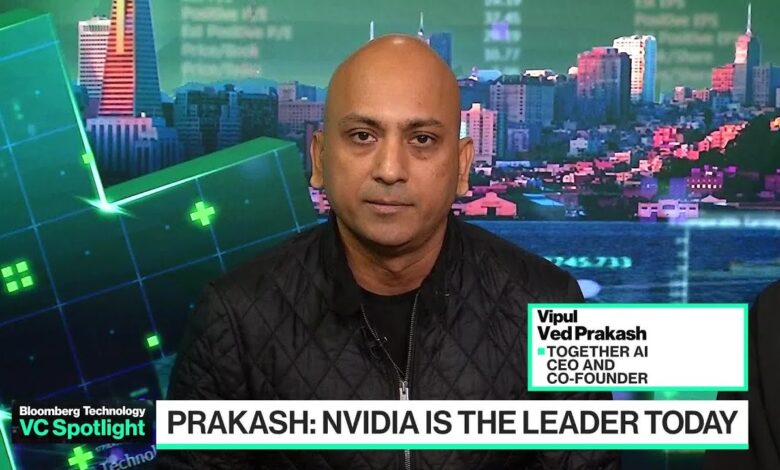
Keep the Code Behind AI Open, Say Two Entrepreneurs
Keep the code behind ai open say two entrepreneurs – Keep the code behind AI open, say two entrepreneurs – a bold statement echoing through the tech world! This isn’t just about lines of code; it’s a debate shaping the future of artificial intelligence, touching upon innovation, accessibility, and the very ethics of progress. Are the potential benefits of open-sourcing AI – faster development, broader collaboration, and democratized access – worth the risks?
Let’s dive into this fascinating discussion and explore the arguments from both sides.
The entrepreneurs championing open-source AI argue that by making the underlying code public, we unlock a wave of innovation. More minds contributing means faster progress, leading to breakthroughs we might otherwise miss. They point to successful open-source projects in other fields, like Linux, as evidence of the power of collaborative development. However, the counterargument highlights significant concerns. Unfettered access to powerful AI algorithms could lead to misuse, from creating sophisticated deepfakes to developing autonomous weapons.
The economic implications are also complex, potentially disrupting existing market structures and raising questions about the sustainability of open-source AI projects.
The Entrepreneurs’ Argument
The two entrepreneurs, having already addressed the preliminary concerns surrounding AI development, now focus their attention on the crucial benefits of embracing open-source AI. Their core argument centers on the idea that open collaboration fosters innovation and democratizes access to this powerful technology, ultimately benefiting society as a whole. They believe that the current trend toward closed-source AI models, while offering immediate profits for corporations, ultimately stifles progress and exacerbates existing inequalities.The entrepreneurs highlight several key advantages of open-source AI.
Firstly, open collaboration leads to faster innovation. Many eyes examining the codebase identify and correct errors more quickly, leading to more robust and reliable models. Secondly, open-source AI significantly lowers the barrier to entry for researchers and developers, particularly those in under-resourced communities. This accessibility promotes a more diverse and inclusive AI ecosystem, leading to innovative solutions tailored to a wider range of needs.
The debate rages on: should we keep the code behind AI open? Two entrepreneurs I spoke with strongly advocate for transparency. They argue that the potential for misuse is huge, especially considering how quickly things can unravel, like Bolivia’s economy, which, as you can see from this article, bolivias slow motion economic crisis is accelerating , highlights the importance of understanding complex systems.
Open-source AI could help us better predict and mitigate such crises before they become uncontrollable, just as open access to data and code fosters collaboration in solving problems.
Finally, open-source models promote transparency and accountability, allowing for scrutiny and mitigating the risk of biased or harmful algorithms.
Examples of Successful Open-Source Projects
The success of open-source methodologies in related fields strongly supports the entrepreneurs’ claims. The Linux operating system, for example, demonstrates the power of collaborative development, resulting in a robust and widely used system. Similarly, the Apache web server, a cornerstone of the internet’s infrastructure, showcases the benefits of open collaboration in creating secure and reliable software. These projects, developed and improved by a global community, serve as compelling evidence that open-source models can produce high-quality, impactful results.
Another notable example is TensorFlow, an open-source machine learning framework used by researchers and developers worldwide, demonstrating the power of collaborative development in advancing AI research.
Open-Source vs. Closed-Source AI: A Comparison
The entrepreneurs emphasize the importance of considering the trade-offs between open-source and closed-source AI models. The following table summarizes the key advantages and disadvantages of each approach:
Advantage/Disadvantage | Open-Source | Closed-Source | Justification |
---|---|---|---|
Innovation Speed | Faster | Slower | Collaborative development and rapid bug fixing in open-source lead to quicker advancements. Closed-source development relies on a smaller team. |
Accessibility | High | Low | Open-source models are freely available to anyone, fostering wider participation and use. Closed-source models are proprietary and restricted. |
Transparency | High | Low | Open-source code is publicly available for review and scrutiny, promoting accountability and trust. Closed-source code is opaque and subject to less scrutiny. |
Cost | Generally lower | Generally higher | Open-source models are often free to use, while closed-source models often involve licensing fees or subscription costs. |
Security | Potentially higher risk (due to public scrutiny) but also faster patching | Potentially lower risk (due to limited access) but slower patching | Open source allows for faster identification and remediation of vulnerabilities, while closed source may hide vulnerabilities but makes patching slower due to limited access to the code. |
Control | Shared | Centralized | Open-source projects are governed by a community, while closed-source projects are controlled by a single entity. |
Counterarguments and Concerns Regarding Open-Source AI: Keep The Code Behind Ai Open Say Two Entrepreneurs
The allure of open-source AI, with its promise of democratized innovation and accelerated progress, is undeniable. However, unfettered access to sophisticated AI code also presents significant risks and ethical dilemmas that demand careful consideration. While the potential benefits are substantial, the potential for misuse and unintended consequences cannot be ignored. A balanced perspective requires acknowledging both sides of this complex issue.Open-source AI introduces several potential risks, primarily stemming from the ease with which powerful algorithms can be accessed and modified by individuals with malicious intent.
The lack of control over distribution and application creates vulnerabilities that could be exploited for harmful purposes. This isn’t merely a hypothetical concern; the rapid advancement of AI technology necessitates a proactive approach to mitigating these risks.
Potential for Misuse and Malicious Applications
The accessibility inherent in open-source models makes them vulnerable to exploitation by malicious actors. Sophisticated AI algorithms, initially designed for beneficial purposes, could be adapted for creating deepfakes, crafting more convincing phishing scams, developing advanced autonomous weapons systems, or even designing more effective malware. The ease of modification allows for the circumvention of safety mechanisms built into proprietary systems, potentially leading to unforeseen and harmful outcomes.
For instance, an open-source facial recognition algorithm could be repurposed for mass surveillance or discriminatory profiling, bypassing the ethical safeguards implemented by responsible organizations.
The debate around open-source AI is heating up, with entrepreneurs advocating for transparency. It reminds me of how easily misinformation can derail important discussions, like the way Andrew McCarthy details in his article, andrew mccarthy this bogus story launched the collusion probe , where a false narrative had significant consequences. Ultimately, keeping AI code open ensures accountability and prevents similar situations from arising within the tech world.
Ethical Implications of Open-Source AI
The ethical implications of open-source AI extend beyond the potential for malicious use. Even with good intentions, the lack of centralized control and oversight can lead to unintended biases and discriminatory outcomes. If a flawed or biased algorithm is made publicly available, its impact could be far-reaching and difficult to contain. The responsibility for ethical considerations becomes diffuse, making accountability challenging.
Furthermore, the potential for the technology to fall into the wrong hands – individuals or groups lacking the ethical framework or expertise to handle it responsibly – is a significant concern.
Examples of Open-Source Technology Outcomes
The history of open-source software provides valuable lessons. The Linux operating system, a prime example of successful open-source development, has demonstrably improved the accessibility and affordability of computing. However, open-source software has also been exploited for malicious purposes, such as creating viruses and other forms of malware. The development of cryptographic tools, while offering enhanced security for many, has also been utilized by criminals to encrypt illegal activities.
This dual nature of open-source technologies – capable of both immense good and significant harm – highlights the necessity for careful consideration and proactive risk mitigation strategies in the context of AI.
Economic and Market Impacts of Open-Source AI
The rise of open-source AI presents a fascinating paradox: it simultaneously democratizes access to powerful technology while disrupting established market dynamics. This shift has profound implications for both established tech giants and the burgeoning AI industry as a whole, affecting everything from profit models to the very nature of innovation. Understanding these economic and market impacts is crucial for navigating the future of AI.The potential impact of open-source AI on the existing AI market landscape is multifaceted.
Initially, it might seem like a threat to companies relying on proprietary, closed-source models. However, a more nuanced perspective reveals a potential for both disruption and collaboration.
Profit Generation in Open-Source vs. Closed-Source AI
Profit generation in the open-source AI space differs significantly from the closed-source model. Closed-source companies typically monetize through direct sales of AI products or services, licensing fees, or cloud-based access to their models. Open-source projects, on the other hand, often rely on indirect revenue streams. These can include offering premium support, specialized training, consulting services, or developing complementary products and services that integrate with the open-source AI.
The success of companies like Red Hat, which built a substantial business around supporting open-source Linux, provides a compelling case study for this model. Open-source AI companies might also attract investment based on the potential for future growth and market share, even if their core technology is freely available.
Community Contributions to Open-Source AI
Community contributions are the lifeblood of successful open-source AI projects. The collaborative nature of these projects allows for rapid innovation and improvement, drawing on the expertise of a diverse global community of developers, researchers, and enthusiasts. This collective effort leads to faster bug fixes, the addition of new features, and the overall enhancement of the AI model’s capabilities.
The debate around open-source AI is heating up, with entrepreneurs advocating for transparency. It makes you think about the implications of secrecy in other areas, like the recent news that the us government seized over 11000 non classified documents from Trump’s home , highlighting how access to information, or lack thereof, can have huge consequences. Ultimately, keeping AI code open feels crucial for accountability and preventing potential misuse, mirroring the need for transparency in government dealings.
For example, the rapid advancements in large language models (LLMs) have been significantly driven by the open-source community’s contributions, improvements, and adaptation of existing models. This collaborative development significantly reduces the cost and time associated with research and development, accelerating the overall progress of the field.
Hypothetical Scenario: Widespread Open-Source AI Adoption
Imagine a scenario where a highly efficient, open-source AI model for medical image analysis becomes widely adopted. Hospitals and clinics, previously reliant on expensive proprietary software, could now access this superior technology at a fraction of the cost. This could lead to improved diagnostic accuracy, faster treatment times, and ultimately, better patient outcomes. While established medical imaging companies might initially face reduced sales, the increased accessibility of advanced AI could stimulate overall market growth by expanding the reach of diagnostic capabilities to underserved areas and populations.
This scenario illustrates how open-source AI can simultaneously disrupt existing markets and create new opportunities for growth and innovation. A similar effect could be seen in other sectors, such as agriculture, where open-source AI could optimize crop yields and resource management, leading to increased productivity and economic benefits for farmers, while potentially challenging the established agricultural technology providers.
Technological and Practical Considerations
The decision to keep AI code open-source presents a fascinating paradox: the collaborative potential is immense, yet the technical and logistical hurdles are significant. Successfully navigating these challenges requires a nuanced understanding of the complexities involved in maintaining, updating, and governing large-scale, collaborative AI projects. This section explores the key technological and practical considerations that must be addressed to ensure the long-term viability and success of open-source AI initiatives.Maintaining and updating open-source AI codebases is a complex undertaking.
The sheer size and complexity of modern AI models, coupled with the rapid pace of technological advancement, necessitate a robust and scalable infrastructure. Version control, testing, and deployment become exponentially more challenging as the number of contributors and the size of the codebase increase. Furthermore, ensuring compatibility across different hardware and software platforms adds another layer of complexity.
The constant need for updates to address bugs, vulnerabilities, and improve performance necessitates a well-defined process for code review, testing, and release management. This process must be transparent and inclusive to foster community participation and maintain trust.
Technical Challenges in Maintaining Open-Source AI Codebases, Keep the code behind ai open say two entrepreneurs
Managing the technical aspects of large open-source AI projects requires a multi-faceted approach. Consider the challenges associated with integrating new features, addressing bugs reported by the community, and ensuring the codebase remains secure. For example, the collaborative development of large language models (LLMs) like GPT-Neo or Stable Diffusion requires careful management of code branches, rigorous testing procedures, and a clear communication strategy to avoid conflicts and maintain code quality.
Without a structured approach, the project can quickly become unwieldy and difficult to maintain. The use of tools such as GitHub, GitLab, or similar platforms is essential, but effective use requires dedicated resources and community buy-in.
Community Governance and Collaboration in Open-Source AI
Effective community governance is crucial for the success of any open-source project, and open-source AI is no exception. Establishing clear guidelines for contribution, code review, and decision-making is paramount. This requires a robust governance model that balances the need for rapid innovation with the importance of maintaining code quality and security. A strong community culture of collaboration, respect, and inclusivity is also essential.
This includes fostering a welcoming environment for newcomers and ensuring diverse perspectives are represented in the decision-making process. Examples of successful community governance models can be found in projects like Linux and Apache, which demonstrate the importance of well-defined processes and a strong community ethos. These models often involve a combination of community voting, leadership councils, and technical steering committees.
Solutions for Managing Large Open-Source AI Projects
Addressing the challenges of managing large open-source AI projects requires a combination of technical and organizational solutions. This includes investing in robust infrastructure for version control, testing, and deployment; developing clear guidelines for code contribution and review; establishing a strong community governance model; and fostering a culture of collaboration and inclusivity. Specific tools and strategies might include:
- Implementing automated testing and continuous integration/continuous deployment (CI/CD) pipelines.
- Utilizing modular design principles to facilitate independent development and updates of different components.
- Establishing a clear contribution process with well-defined roles and responsibilities.
- Creating a dedicated team or community group responsible for code maintenance and quality assurance.
- Leveraging cloud-based infrastructure to scale resources as needed.
These solutions, when implemented effectively, can significantly reduce the risk of code fragmentation, improve the quality and reliability of the AI models, and facilitate broader adoption.
Influence of Licensing Models on Open-Source AI Adoption
The choice of licensing model significantly impacts the adoption and development of open-source AI. Different licenses impose varying restrictions on the use, modification, and distribution of the software. Permissive licenses, such as MIT or Apache 2.0, allow for greater freedom in using and modifying the code, potentially leading to wider adoption. However, they offer less protection for the original creators.
Copyleft licenses, such as GPL, require derivative works to also be open-source, promoting further collaboration but potentially limiting commercial applications. The selection of an appropriate license is a critical decision that balances the desire for broad adoption with the need to protect the intellectual property rights of the contributors. Choosing a license that aligns with the project’s goals and the community’s values is crucial for its long-term success.
The impact of different licenses is often seen in the differing levels of commercial adoption observed in projects under different licensing regimes. For example, projects under permissive licenses tend to have more commercial applications compared to projects under copyleft licenses.
Illustrative Example: Open-Source AI for Crop Disease Detection
This section details a hypothetical open-source AI project focused on improving crop yields through early disease detection. The project aims to empower farmers, particularly in developing countries, with accessible and affordable technology to combat crop losses due to disease. This is crucial as crop diseases significantly impact food security and economic stability globally.
Project Goals and Functionality
The project, named “CropAI,” aims to create a user-friendly mobile application and accompanying open-source software library. The application would allow farmers to photograph their crops, and CropAI would analyze the images using a pre-trained convolutional neural network (CNN) model to identify potential diseases. The application would then provide recommendations for treatment, preventative measures, and relevant resources. The open-source library would enable researchers and developers to improve the model, add support for new crops and diseases, and adapt it to various contexts.
This accessibility is crucial for fostering collaboration and improvement.
Technical Architecture and Key Components
CropAI’s technical architecture consists of three main components: a mobile application (frontend), a cloud-based API (backend), and the open-source AI model (model). The mobile application is developed using cross-platform frameworks like React Native or Flutter to ensure broad device compatibility. The backend API, built using Python and a framework like Flask or Django, handles image uploads, model inference, and result delivery.
The core component is the CNN model, trained on a large dataset of labeled crop images representing various diseases and healthy crops. This model is open-source, allowing for community contributions and improvements. The model’s architecture could be based on efficient and well-established architectures like MobileNet or EfficientNet, optimized for mobile deployment and low-power devices.
Development and Deployment Process
The development process would follow an iterative agile methodology, with continuous integration and continuous deployment (CI/CD) pipelines for efficient updates and bug fixes. Initial model training would involve a collaborative effort, potentially utilizing publicly available datasets and contributions from agricultural researchers. Challenges could include acquiring sufficient and high-quality labeled data, ensuring model robustness across different environmental conditions, and managing the complexities of deploying a machine learning model on resource-constrained mobile devices.
Successes would be measured by the accuracy of disease detection, the ease of use of the application, and the community’s engagement in improving the model and expanding its capabilities. The project would leverage open-source tools and platforms throughout the development lifecycle, promoting transparency and collaboration.
Societal Impact
The potential societal impact of CropAI is significant, encompassing both positive and negative aspects:
The importance of considering both positive and negative impacts is paramount for responsible technology development. A comprehensive analysis allows for proactive mitigation of potential harms and maximization of benefits.
- Positive Impacts: Increased crop yields, reduced food insecurity, improved farmer livelihoods, reduced pesticide use (through targeted treatment), increased access to agricultural information, fostered collaboration between researchers and farmers.
- Negative Impacts: Potential for misuse of the technology (e.g., for malicious purposes), reliance on technology might decrease traditional farming knowledge, data privacy concerns regarding farmer data, digital divide excluding farmers without access to smartphones or internet.
The debate surrounding open-source AI is far from settled. While the potential for accelerated innovation and democratized access is undeniable, the risks of misuse and the challenges of maintaining large, collaborative projects are significant. Ultimately, the path forward requires careful consideration of both the benefits and the dangers. Finding a balance between fostering collaboration and mitigating potential harm will be crucial in shaping a responsible and beneficial future for artificial intelligence.
The conversation continues, and it’s one we all need to be a part of.