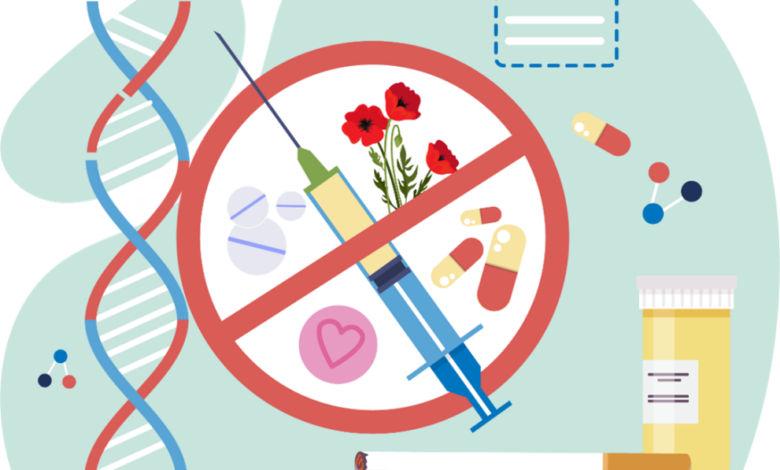
LLMs Now Write Lots of Science Good
LLMs Now Write Lots of Science Good – that’s the exciting, and slightly unsettling, reality we’re facing. Large language models are rapidly transforming how scientific research is conducted, from literature reviews to hypothesis generation. This isn’t just about automating tedious tasks; we’re talking about AI potentially reshaping the very fabric of scientific discovery and communication. But with this incredible potential comes a need for careful consideration of ethical implications and potential pitfalls.
This post dives into the current capabilities and limitations of LLMs in scientific writing, exploring their applications in various research areas, and examining the broader impact on scientific communication and dissemination. We’ll look at both the incredible opportunities and the very real challenges that lie ahead.
Applications of LLMs in Scientific Research: Llms Now Write Lots Of Science Good
Large language models (LLMs) are rapidly transforming various fields, and their potential impact on scientific research is particularly exciting. Their ability to process and understand vast amounts of text data opens up new avenues for accelerating discovery and enhancing the research process. This exploration delves into the specific applications of LLMs in scientific research, examining both their benefits and potential ethical considerations.
LLMs in Literature Reviews and Summarization
LLMs excel at processing large volumes of text. In scientific research, this translates to significantly faster and more efficient literature reviews. Researchers can input s related to their research topic, and the LLM can quickly identify and summarize relevant papers, highlighting key findings and methodologies. This allows researchers to gain a comprehensive understanding of the existing literature much more quickly than traditional manual methods.
For instance, an LLM could be used to summarize all published research on the effects of a specific drug on a particular disease, extracting key efficacy and safety data from hundreds of studies within hours. This summarized information can then be used to inform the design of new experiments or refine existing hypotheses.
LLMs in Hypothesis Generation and Experimental Design
Beyond literature review, LLMs can assist in the crucial stages of hypothesis generation and experimental design. By analyzing existing data and literature, LLMs can identify gaps in knowledge and suggest potential hypotheses to investigate. They can also aid in designing experiments by suggesting appropriate methodologies, control groups, and data collection techniques based on the research question and available resources.
It’s amazing how LLMs are now churning out scientific papers – a real game-changer! This progress makes me think about the importance of fair and accessible processes, like the one highlighted in this recent news story: appeals court allows congressional candidates to challenge Californias election laws. Ensuring equitable access to information and representation is crucial, just as the accessibility of scientific knowledge should be.
Ultimately, LLMs improving science and fair elections are both steps towards a better future.
For example, an LLM might suggest a specific statistical model to analyze data based on the experimental design, or it might propose a novel experimental setup based on the identified gaps in the literature. This collaborative approach can lead to more innovative and efficient research strategies.
LLMs in Data Analysis and Interpretation
Scientific research often involves analyzing large and complex datasets. LLMs can be instrumental in this process. While they cannot replace specialized statistical software, LLMs can assist in data cleaning, identifying patterns and anomalies, and generating initial interpretations. For example, an LLM could analyze genomic data to identify potential biomarkers associated with a disease, or it could analyze climate data to identify trends and predict future changes.
However, it’s crucial to remember that LLMs should be used as tools to assist researchers, not replace their expertise in data analysis and interpretation. The final analysis and conclusions must always be reviewed and validated by human scientists.
Ethical Implications of LLMs in Scientific Research
The use of LLMs in scientific research raises several ethical considerations, most notably authorship and plagiarism. The question of authorship becomes complex when an LLM significantly contributes to the research process. Clear guidelines are needed to determine the appropriate level of LLM contribution that warrants authorship credit. Similarly, the potential for plagiarism is a concern. Researchers must ensure that they properly cite and attribute any information generated by an LLM to avoid misrepresenting the work as their own.
It’s amazing how LLMs are now generating high-quality scientific papers, a real boon for research. However, the advancements in AI don’t negate real-world issues like the recent arrests in Michigan, as highlighted in this article: illegal immigrants arrested in michigan include thrice deported drug dealer , which reminds us that complex societal problems still require human attention.
Ultimately, both technological progress and addressing pressing social concerns are crucial for a better future.
Strict adherence to academic integrity standards is crucial to maintain the credibility and trustworthiness of scientific research. Transparency in the use of LLMs in the research process is essential to address these ethical concerns.
Hypothetical Workflow: LLM Integration in Cancer Research
Consider a research project investigating the efficacy of a novel drug against a specific type of cancer. An LLM could be integrated into the workflow as follows: First, the LLM would conduct a comprehensive literature review on the target cancer and existing treatments, summarizing key findings and identifying knowledge gaps. Second, the LLM could assist in hypothesis generation by suggesting potential mechanisms of action for the novel drug and predicting its potential efficacy based on existing data.
Third, the LLM could aid in experimental design by suggesting appropriate cell lines, animal models, and experimental protocols. Fourth, after data collection, the LLM could assist in analyzing the results, identifying trends, and generating preliminary interpretations. Finally, the researchers would critically evaluate the LLM’s contributions, validate the findings through rigorous statistical analysis, and write the research paper, clearly acknowledging the LLM’s role in the research process.
This collaborative approach ensures both efficiency and rigorous scientific integrity.
It’s amazing how LLMs are revolutionizing scientific writing, churning out papers and reports at an unprecedented rate. But this raises a fascinating question about digital legacies; what happens to all this AI-generated content after the creators are gone? It’s a question worth pondering, especially considering the ethical and practical implications, as explored in this insightful article: what should happen to a persons digital remains.
Ultimately, the impact of LLMs on science is huge, and understanding how we manage the resulting data is crucial for the future.
Impact on Scientific Communication and Dissemination
Large language models (LLMs) are poised to revolutionize how scientific research is communicated and disseminated, impacting everything from the speed of publication to the accessibility of information for a global audience. Their ability to process and generate text offers exciting possibilities, but also presents significant challenges that need careful consideration.LLMs could significantly accelerate the scientific publication process. The tedious tasks of writing abstracts, summarizing findings, and even generating initial drafts of manuscripts could be automated, freeing up researchers’ time for more creative and analytical work.
Imagine a system where an LLM could synthesize complex data sets into concise and informative summaries, allowing for quicker peer review and publication.
LLM Effects on Accessibility of Scientific Information
The democratization of scientific knowledge is a key benefit of LLMs. These models can translate scientific papers into multiple languages, making them accessible to a far wider audience than traditional methods allow. Furthermore, LLMs can simplify complex scientific concepts, making them understandable to individuals without specialized scientific training. Consider the potential for LLMs to generate easily digestible summaries of cutting-edge research for the general public, fostering a more informed and scientifically literate society.
This increased accessibility could lead to broader public engagement with scientific advancements and potentially accelerate the translation of research into practical applications.
Potential for LLMs to Improve or Hinder Scientific Communication Quality
LLMs offer the potential to improve the clarity and precision of scientific writing. They can identify grammatical errors, suggest improved sentence structure, and even flag potential inconsistencies in logic. However, the use of LLMs also raises concerns about the potential for oversimplification, the loss of nuanced arguments, and the generation of inaccurate or misleading information. Over-reliance on LLMs could lead to a homogenization of scientific writing styles, potentially diminishing the unique voices and perspectives of individual researchers.
The challenge lies in using LLMs as a tool to enhance, not replace, human expertise in scientific communication.
Challenges in Verifying the Accuracy of LLM-Generated Scientific Information, Llms now write lots of science good
One of the major hurdles in adopting LLMs for scientific communication is the difficulty in verifying the accuracy of the information they generate. LLMs are trained on vast datasets, but these datasets can contain errors, biases, or outdated information. This can lead to LLMs generating factually incorrect statements or presenting biased interpretations of data. Robust fact-checking mechanisms and rigorous validation processes are crucial to ensure the reliability of LLM-generated scientific content.
The development of methods for detecting and correcting inaccuracies produced by LLMs is a critical area of ongoing research.
Benefits and Drawbacks of Using LLMs for Scientific Communication
The potential benefits and drawbacks of using LLMs for scientific communication are substantial and require careful consideration.
Before listing the points, it’s important to remember that the successful integration of LLMs into scientific communication hinges on responsible development and implementation, emphasizing human oversight and critical evaluation.
- Benefits:
- Accelerated publication process
- Improved accessibility of scientific information
- Enhanced clarity and precision of scientific writing
- Increased efficiency in literature reviews and data synthesis
- Facilitated cross-lingual communication
- Drawbacks:
- Potential for inaccuracies and biases
- Risk of oversimplification and loss of nuance
- Challenges in verifying the accuracy of generated information
- Potential for plagiarism or unintentional duplication of content
- Ethical concerns regarding authorship and intellectual property
Future Directions and Challenges
The integration of Large Language Models (LLMs) into scientific research presents a paradigm shift, offering immense potential but also raising significant challenges. While LLMs currently excel at tasks like literature review and summarization, their limitations in nuanced understanding and critical evaluation necessitate further development to fully harness their power. Addressing these challenges is crucial to ensuring responsible and effective use of this transformative technology.LLMs currently struggle with the subtleties of scientific reasoning, often producing outputs that are factually correct but lack the depth of analysis and critical thinking expected in scientific publications.
They can also be prone to generating plausible-sounding but ultimately incorrect or misleading information, highlighting the need for robust verification methods. Moreover, ethical concerns surrounding authorship, plagiarism, and bias in algorithms demand careful consideration.
Addressing Limitations in Scientific Writing
Future advancements in LLM technology will likely focus on improving their understanding of scientific context and reasoning. This includes developing models capable of handling complex scientific terminology, integrating diverse data types (including experimental data and images), and performing sophisticated analyses beyond simple summarization. Techniques like incorporating symbolic reasoning capabilities and fine-tuning models on high-quality, curated scientific datasets will be essential.
Imagine an LLM that can not only summarize a research paper but also identify potential flaws in the methodology, suggest alternative experimental designs, and even formulate novel hypotheses based on the data presented. This level of sophistication is within reach, though significant research and development are required.
Methods for Detecting LLM-Generated Scientific Text
The ability to reliably detect LLM-generated text is paramount to maintaining the integrity of scientific publications. Current methods, often based on statistical analysis of language patterns and stylistic features, are continually evolving as LLMs become more sophisticated. Future approaches may involve analyzing the logical consistency of arguments, the presence of subtle biases, and the coherence of the text’s structure within the broader scientific context.
Developing sophisticated watermarking techniques that subtly embed information within LLM-generated text could also prove invaluable in identifying its origin. This is a critical area of research, as the potential for misuse of LLMs to generate fraudulent scientific results is a real concern. For instance, a sophisticated detection system could flag papers submitted to journals that exhibit statistically unusual patterns of language, potentially indicative of LLM authorship.
Strategies for Responsible Use of LLMs in Scientific Research
Establishing clear guidelines and ethical frameworks for the use of LLMs in scientific research is crucial. This involves defining authorship criteria, ensuring transparency in the use of LLMs in research processes, and developing mechanisms to address potential biases in LLM outputs. Educational initiatives aimed at training scientists in the responsible use of LLMs are also necessary. Institutions and journals should implement policies that clearly Artikel acceptable uses of LLMs and the necessary disclosures required when using them.
For example, a journal might require authors to explicitly state the role of an LLM in the research process, much like they currently require declarations of conflicts of interest.
Open Research Questions Related to LLM Integration
The integration of LLMs into scientific workflows raises several important questions that require further investigation:
- How can we ensure the fairness and equity of LLM-driven scientific discovery, mitigating biases present in training data?
- What are the long-term societal impacts of automating aspects of scientific research using LLMs?
- How can we effectively evaluate the reliability and validity of scientific findings generated with the assistance of LLMs?
- What are the legal and ethical implications of using LLMs to generate intellectual property?
- How can we develop LLM-based tools that facilitate effective collaboration and knowledge sharing among scientists across geographical boundaries and disciplinary lines?
Illustrative Scenario: Impact on Drug Discovery
Consider the field of drug discovery. Advanced LLMs could analyze vast datasets of molecular structures, biological pathways, and clinical trial results to identify potential drug candidates with significantly higher efficiency than current methods. Imagine an LLM capable of not only predicting the efficacy of a molecule against a specific target but also predicting potential side effects and drug-drug interactions, drastically reducing the time and cost associated with drug development.
Such a system could revolutionize the pharmaceutical industry, accelerating the development of life-saving drugs for diseases like cancer and Alzheimer’s. This hypothetical scenario demonstrates the transformative potential of advanced LLMs, but it also highlights the need for rigorous validation and ethical oversight to ensure the responsible application of this powerful technology.
The integration of LLMs into scientific workflows is still in its early stages, but the potential benefits are undeniable. From accelerating research to making scientific knowledge more accessible, LLMs could revolutionize how we approach science. However, responsible development and deployment are crucial to mitigate risks and ensure ethical use. We need robust methods for detecting AI-generated content, clear guidelines for authorship, and ongoing research to address the many open questions surrounding this rapidly evolving technology.
The future of science is being written, and LLMs are playing an increasingly significant role in the process.