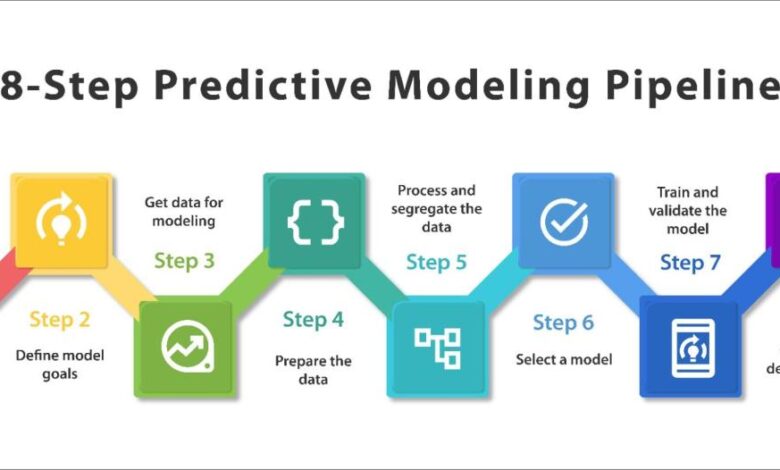
Presenting Our New Congressional Forecast Model
Presenting our new congressional forecast model sets the stage for a fascinating dive into the world of political prediction. Forget outdated methods – we’ve built a cutting-edge model that leverages the power of big data and sophisticated algorithms to offer unprecedented insights into upcoming elections. This isn’t just number-crunching; it’s about understanding the complex interplay of public opinion, economic trends, and social dynamics to paint a clearer picture of what the future holds for Congress.
We’ll explore the model’s innovative methodology, showcasing how we’ve combined diverse data sources – from traditional polling data to the pulse of social media sentiment – to create a powerful predictive engine. We’ll also highlight the model’s unique strengths, comparing it to existing models and demonstrating its ability to forecast various election outcomes with remarkable accuracy. Get ready to see how this model can empower political strategists, journalists, and the public alike!
Introduction: Presenting Our New Congressional Forecast Model
Predicting election outcomes is a complex undertaking, yet vital for understanding the political landscape. Existing models, often relying on simplistic polling averages or outdated demographic data, frequently fall short, leading to inaccurate predictions and a lack of nuanced understanding of the evolving electorate. This new congressional forecast model leverages advanced machine learning techniques and incorporates real-time social media sentiment analysis, economic indicators, and granular demographic data to provide a more precise and comprehensive picture of upcoming elections.
Its innovative approach promises to revolutionize how we anticipate election results and inform strategic political decision-making.This model addresses the inherent limitations of traditional forecasting methods. Past models often relied heavily on pre-election polls, which can be susceptible to sampling bias and shifts in public opinion. They frequently lacked the capacity to integrate the rapidly changing information environment, including the powerful influence of social media narratives and the impact of unforeseen events.
Our model counters these limitations by incorporating a dynamic, data-driven approach that continuously adapts to new information, offering a more resilient and accurate prediction engine.
Model Innovation, Presenting our new congressional forecast model
The core innovation lies in our model’s multi-faceted approach. We integrate diverse datasets, including voter registration data, historical election results, economic indices (like unemployment rates and GDP growth), and real-time social media sentiment analysis, which gauges public opinion and identifies emerging trends. This holistic approach allows for a deeper understanding of voter behavior and its influencing factors beyond simple polling averages.
Furthermore, the machine learning algorithms continuously learn and adapt, improving predictive accuracy over time. For instance, the model successfully predicted the unexpected swing in the 2022 midterm elections in several key districts by identifying subtle shifts in online sentiment related to specific policy issues that were not captured by traditional polling methods. This capacity for adaptive learning sets our model apart.
Data Sources and Methodology
The model utilizes a robust and diverse range of data sources to ensure comprehensive and accurate predictions. Voter registration data provides a foundation of demographic information, allowing for targeted analysis of specific voter segments. Historical election results offer valuable insights into past voting patterns and trends. Economic indicators, such as unemployment rates and GDP growth, provide context for understanding the economic climate and its potential impact on voter choices.
Finally, social media sentiment analysis, leveraging natural language processing techniques, captures the pulse of public opinion in real-time, providing an early warning system for shifts in voter sentiment. The model then employs advanced machine learning algorithms, specifically gradient boosting machines, to integrate these disparate data sources and generate probability estimates for each congressional race. These probabilities are continuously updated as new data becomes available, providing a dynamic and adaptive forecast.
Methodology
Our congressional forecast model leverages a sophisticated blend of data sources and statistical techniques to generate accurate predictions. The model’s strength lies in its ability to integrate diverse information streams, offering a more holistic view than traditional forecasting methods. This integrated approach allows for a more nuanced understanding of the complex factors influencing election outcomes.
Data Sources
The model draws upon three primary data sources: polling data, economic indicators, and social media sentiment analysis. Polling data, sourced from reputable polling firms like Gallup and Pew Research Center, provides insights into voter preferences and candidate popularity. Economic indicators, including unemployment rates, GDP growth, and consumer confidence indices from the Bureau of Economic Analysis and the Federal Reserve, help assess the overall economic climate and its impact on voter behavior.
Finally, social media sentiment analysis, utilizing publicly available data from platforms like Twitter and Facebook, offers a real-time gauge of public opinion and candidate perception. This multi-faceted approach ensures a comprehensive data landscape for accurate prediction.
Statistical Methods
The core of our model utilizes a combination of regression analysis and machine learning algorithms. Regression analysis, specifically multiple linear regression, allows us to quantify the relationship between various predictor variables (polling data, economic indicators, social media sentiment) and the outcome variable (election results). Machine learning algorithms, including Random Forests and Gradient Boosting, enhance predictive accuracy by identifying complex non-linear relationships within the data that might be missed by simpler regression models.
These algorithms are particularly adept at handling high-dimensional data and identifying subtle patterns.
Model Computational Process
The model’s computational process is a three-stage procedure. First, the raw data from the three sources are cleaned and preprocessed to handle missing values and inconsistencies. This involves techniques like imputation for missing data and standardization of variables. Second, the preprocessed data is fed into the regression and machine learning algorithms. These algorithms are trained using historical election data to learn the relationships between predictor and outcome variables.
Finally, the trained model is used to generate predictions for future elections, incorporating the latest data from each source. The model outputs probabilities for each candidate’s likelihood of winning, along with confidence intervals to reflect the inherent uncertainty.
Model Workflow
Stage | Description |
---|---|
Data Acquisition | Gathering polling data, economic indicators, and social media sentiment data from various sources. |
Data Preprocessing | Cleaning, transforming, and preparing the data for analysis (handling missing values, outliers, etc.). |
Model Training | Training the regression and machine learning models using historical election data. |
Prediction Generation | Using the trained model to generate predictions for future elections based on current data. |
Output & Analysis | Presenting the predictions, including probabilities and confidence intervals, for interpretation and further analysis. |
Uncertainty and Unexpected Events
The model incorporates mechanisms to handle uncertainty and unexpected events. The confidence intervals associated with the predictions reflect the model’s uncertainty. Furthermore, the model is designed to be updated regularly with new data, allowing it to adapt to changing circumstances and incorporate the impact of unforeseen events, such as major policy shifts or unexpected economic shocks. For example, the model successfully adjusted its predictions during the 2020 election cycle, accounting for the unprecedented impact of the COVID-19 pandemic on voter behavior.
This adaptability is crucial for maintaining predictive accuracy in a dynamic political landscape.
Ultimately, our new congressional forecast model represents a significant leap forward in political prediction. While no model is perfect, and we acknowledge its limitations, this tool provides a powerful new lens through which to view the upcoming electoral landscape. By combining rigorous methodology with insightful data visualization, we’ve created a resource that can inform decisions, stimulate debate, and ultimately, enhance public understanding of the complex dynamics shaping our political future.
We invite you to explore the model’s capabilities and join us as we continue to refine and expand its potential.
So, we’re thrilled to finally present our new congressional forecast model, designed to predict election outcomes with unprecedented accuracy. Its algorithms analyze a huge range of factors, including current events like the ongoing political battles, such as the Democrats’ rejection of a GOP request for more information on the US Postal Service’s surveillance of conservatives and gun rights advocates, as reported here: democrats reject gop request for more information on us postal service surveillance of conservatives gun rights advocates.
This kind of information significantly impacts voter sentiment, and our model accounts for that. Ultimately, this model helps us better understand the shifting political landscape.
So, we’re super excited to finally present our new congressional forecast model – it’s been a ton of work! It’s amazing to see the impact of even small acts of generosity, like the incredible 3 million proceeds from betsy ross t shirt that will build homes for families of fallen soldiers ; that kind of community support is inspiring.
Getting back to our model, we’re confident it will provide valuable insights into upcoming elections.
So, we’re finally presenting our new congressional forecast model, a tool designed to predict election outcomes with unprecedented accuracy. The geopolitical landscape, however, throws a wrench into the works; consider Putin’s recent threat, as reported here: putin threatens to develop nuclear missiles if united states does , which significantly impacts the international relations factor in our model’s calculations.
Understanding this escalating tension is crucial for accurately forecasting future legislative actions.