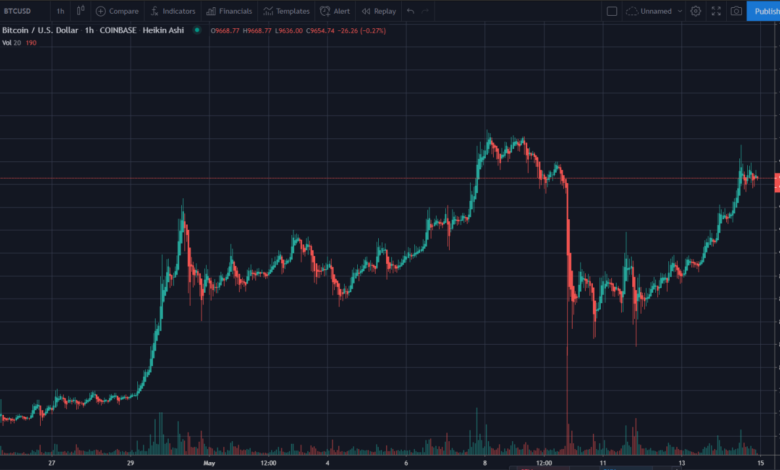
How to Predict Crypto Market Trends Using Data
How to Predict Crypto Market Trends Using Data and Analytics delves into the fascinating world of forecasting cryptocurrency price movements. From meticulous analysis of financial news and social media sentiment to the intricate application of machine learning algorithms, this comprehensive guide unveils the secrets to understanding and predicting the dynamic crypto market.
This exploration will cover various essential facets, including data sourcing, technical indicators, fundamental project evaluations, and the use of machine learning models. We’ll also delve into the crucial elements of volatility prediction, risk management, and the integration of diverse data sources for more accurate predictions. Ultimately, this guide equips you with the knowledge and tools to navigate the complexities of the crypto market with greater confidence.
Data Sources for Crypto Market Prediction
Predicting crypto market trends is a complex endeavor, demanding a multifaceted approach that leverages various data sources. The crypto market’s volatility and decentralized nature necessitate a comprehensive understanding of diverse information streams to gain meaningful insights. A crucial element in successful prediction is the ability to interpret and synthesize information from various sources, recognizing both the strengths and limitations of each.
This exploration will examine key data sources, highlighting their strengths and weaknesses, and discussing the ethical implications of their use.Understanding the market requires looking beyond simple price charts and analyzing diverse data streams. This involves a blend of financial news, social media sentiment, and on-chain activity. Each source offers a unique perspective, but no single source provides a definitive answer.
Careful analysis and integration of information from multiple sources are crucial for formulating accurate predictions.
Financial News Outlets
Financial news outlets, like Bloomberg, CoinDesk, and The Block, provide valuable insights into market sentiment, regulatory developments, and major events impacting cryptocurrencies. These sources often offer expert analysis and commentary, helping to contextualize current market trends. However, these sources can be influenced by biases and may not always reflect the nuanced sentiment of the entire market. Their timeliness is generally high, as they strive to report news as it unfolds.
The accuracy of their predictions is often tied to the accuracy of their analysis and the depth of their reporting. For example, a rapid reporting of a regulatory change can have an immediate and significant impact on the price of affected cryptocurrencies.
Social Media Sentiment Analysis Platforms
Platforms specializing in social media sentiment analysis, such as Brandwatch and Talkwalker, track and analyze public discussions about cryptocurrencies on platforms like Twitter, Reddit, and Discord. These platforms aim to gauge market sentiment by processing and categorizing social media posts. They provide a real-time view of public opinion, which can be a strong indicator of future price movements.
However, social media sentiment analysis can be susceptible to manipulation, and not all opinions reflect the actual market activity. The accuracy of these platforms is dependent on the accuracy of the algorithms used to analyze sentiment. The timeliness of social media sentiment analysis is typically high, as it reflects current public discussions in real-time. For example, a sudden surge of negative comments on a particular cryptocurrency might precede a price drop.
On-Chain Data Providers
On-chain data providers like Glassnode and Santiment offer granular insights into cryptocurrency transaction activity, network health, and market participation. These providers utilize blockchain technology to provide detailed information about transaction volumes, wallet activity, and other key metrics. The data provided by on-chain providers can offer insights into the underlying market dynamics and can be used to identify potential price movements.
However, interpreting on-chain data can be challenging, and it may not always provide a clear picture of the overall market sentiment. The timeliness of on-chain data depends on the frequency of data updates and the processing speed of the provider. For instance, high transaction volume on a specific cryptocurrency might indicate increased interest and potentially higher prices in the future.
Comparison of Data Sources
Data Source | Accuracy | Timeliness | Strengths | Weaknesses |
---|---|---|---|---|
Financial News Outlets | Moderate | High | Expert analysis, broad perspective | Potential bias, limited on-chain data |
Social Media Sentiment Analysis | Moderate | High | Real-time market sentiment | Susceptible to manipulation, limited context |
On-Chain Data Providers | High | Moderate | Granular on-chain insights, market dynamics | Complex data interpretation, potential for lagging indicators |
Ethical Considerations
The use of data sources for predicting crypto market trends raises ethical concerns. Using data to predict market movements could potentially lead to manipulation if not handled responsibly. Transparency in data usage and responsible reporting are crucial. Furthermore, ensuring fairness in access to data and avoiding bias in analysis are essential considerations. The potential for market manipulation through the misuse of prediction tools should be actively addressed.
For instance, dissemination of false or misleading information about market trends can impact investor decisions and potentially cause significant financial losses.
Technical Indicators for Trend Identification: How To Predict Crypto Market Trends Using Data And Analytics
Decoding the cryptic whispers of the crypto market requires more than just intuition. A crucial component of successful prediction lies in understanding and applying technical indicators. These tools, derived from historical price and volume data, offer valuable insights into potential future price movements. This section delves into several key indicators, their historical performance, and the potential biases inherent in their application.
Key Technical Indicators
Technical indicators are mathematical calculations derived from price and volume data. They provide a framework for identifying potential trends and patterns, allowing traders to make more informed decisions. The effectiveness of these indicators, however, is often debated, as they are not foolproof predictors.
Moving Averages
Moving averages are crucial for smoothing out price fluctuations and revealing underlying trends. Different types of moving averages, such as simple moving averages (SMA) and exponential moving averages (EMA), calculate the average price over a specific period. For example, a 20-day SMA calculates the average price over the last 20 days.
- Simple Moving Average (SMA): Calculates the average price over a specific period. It’s a straightforward calculation, but it can lag behind recent price changes, potentially missing short-term trends.
- Exponential Moving Average (EMA): Assigns more weight to recent prices, providing a more responsive measure of current trends. This responsiveness can be advantageous but might amplify short-term volatility.
Relative Strength Index (RSI)
The RSI measures the magnitude of recent price changes to evaluate overbought or oversold conditions. A reading above 70 often suggests an asset is overbought, potentially leading to a price correction. Conversely, a reading below 30 might indicate an asset is oversold, potentially setting the stage for a price rebound.
- Formula: A complex calculation based on the average gains and losses over a specified period. A common formula involves calculating the average gain and loss over the specified period, then using these to determine the RSI. Detailed calculation formulas can be found online.
- Historical Performance: RSI has demonstrated some success in identifying overbought/oversold conditions, but its effectiveness in consistently predicting price reversals is inconsistent.
Moving Average Convergence Divergence (MACD)
MACD tracks the relationship between two moving averages, helping identify momentum shifts. A positive crossover often signifies a bullish trend, while a negative crossover suggests a bearish trend.
- Formula: The calculation involves subtracting a 26-period EMA from a 12-period EMA. A signal line is typically added, which is a 9-period EMA of the MACD line. The exact formula can be found via reliable sources.
- Historical Performance: MACD has proven useful in signaling potential trend changes, but like other indicators, its predictive power is not absolute. False signals and lagging signals are common.
Volume
Volume analysis examines the trading volume associated with price movements. High volume during price increases suggests strong conviction, while low volume during price drops may signal a lack of substantial selling pressure. This data point is often overlooked, but it can be a crucial piece of the puzzle.
Table of Technical Indicators
Indicator | Formula | Interpretation |
---|---|---|
Simple Moving Average (SMA) | [(Price1 + Price2 + … + Pricen) / n] | Average price over a specified period. |
Exponential Moving Average (EMA) | (Previous EMA
|
Assigns more weight to recent prices. |
Relative Strength Index (RSI) | [Average Gain / (Average Gain + Average Loss)] – 100 | Measures magnitude of recent price changes to identify overbought/oversold conditions. |
Moving Average Convergence Divergence (MACD) | (12-period EMA – 26-period EMA) | Tracks the relationship between two moving averages to identify momentum shifts. |
Fundamental Analysis of Crypto Projects
Fundamental analysis in the crypto market goes beyond technical indicators to delve into the intrinsic value and potential of a project. It examines the project’s roadmap, team, community, and market position to assess its long-term viability and predict future performance. This approach requires a deeper understanding of the project’s goals, the team behind it, and the overall market dynamics.Understanding the project’s core tenets and the factors driving its development is crucial.
A thorough analysis of fundamental aspects can help investors differentiate promising projects from those with less potential.
Project Whitepaper Analysis
Project whitepapers serve as the foundational documents outlining the project’s goals, technology, and roadmap. A comprehensive analysis of the whitepaper involves scrutinizing the project’s technical details, intended use cases, and the mechanisms driving its functionality. Understanding the underlying technology, problem it solves, and its proposed solutions is key. Identifying the specific problem the project aims to address and how its proposed solution is unique is crucial.
Evaluating the practicality and scalability of the project’s vision is essential.
Team Expertise
The competence and experience of the project’s team significantly influence its potential success. A strong team with a proven track record in the blockchain or related fields often suggests greater project reliability and long-term potential. Assessing the team’s experience and expertise in the specific area the project addresses, such as development, marketing, or finance, is critical. Consider the team’s previous accomplishments, their experience with similar projects, and the presence of key individuals with recognized expertise in the industry.
Community Engagement
The level of community engagement and support can reflect the project’s appeal and potential adoption rate. A vibrant and active community indicates greater potential for long-term growth and success. Analyzing social media presence, forum discussions, and participation in relevant events helps assess community enthusiasm and potential for future growth. Active participation in community forums and interactions on social media are indicators of community engagement.
Market Capitalization
Market capitalization, reflecting the total value of a cryptocurrency, is a critical factor in evaluating its potential. Higher market capitalization often signifies greater investor confidence and potential for price appreciation. A thorough understanding of the market capitalization in relation to other projects within the same sector is important. Comparing market capitalization with competitor projects provides context. It’s essential to assess market capitalization alongside other factors like project utility and adoption rate.
Fundamental Analysis Method Strengths and Weaknesses
Analysis Method | Strengths | Weaknesses |
---|---|---|
Whitepaper Analysis | Provides insight into project vision and technology. | May lack concrete details and be subject to interpretation. |
Team Expertise | Indicates project reliability and potential. | Difficult to objectively measure team effectiveness. |
Community Engagement | Highlights project popularity and adoption potential. | May not always reflect actual user engagement or project viability. |
Market Capitalization | Reflects investor confidence and market perception. | Doesn’t directly indicate project value or utility. |
Interpreting Fundamental Indicators for Predicting Future Trends
Fundamental indicators, when analyzed collectively, offer a comprehensive picture of a project’s potential. A project with a strong whitepaper, experienced team, engaged community, and a competitive market capitalization is more likely to exhibit positive future market trends. Combining these indicators provides a more holistic understanding of the project’s overall viability. For example, a project with a compelling whitepaper, a team with a proven track record, and a growing community could potentially experience a surge in market capitalization.
Analyzing Metrics for Project Viability
Analyzing various metrics, including community growth, adoption rate, and the project’s market share, provides a clear picture of its viability. Consider metrics such as active users, transaction volume, and the project’s presence in relevant ecosystems to gauge its overall success. Assessing project development milestones against the project roadmap helps evaluate project progress.
Machine Learning Models for Prediction
Diving deeper into the realm of crypto market prediction, machine learning (ML) models offer a powerful toolkit for analyzing vast datasets and identifying patterns that might otherwise be missed. These models can sift through historical price fluctuations, trading volumes, social media sentiment, and news articles to potentially uncover valuable insights into future price movements. However, it’s crucial to remember that crypto markets are inherently volatile, and no model can guarantee perfect accuracy.ML algorithms are not a magic bullet, but they can be valuable tools in a broader strategy for understanding the market.
They are particularly useful for identifying potential trends and patterns that human analysts might overlook, offering a layer of automation and speed in the analysis process. Understanding the strengths and weaknesses of these models is critical for successful implementation.
Suitable Machine Learning Algorithms
Various ML algorithms are suitable for crypto market prediction. Their effectiveness depends on the specific data features and the desired outcome. A diverse selection of algorithms can be used in tandem or independently to enhance prediction accuracy.
- Regression models, such as linear regression and support vector regression, are well-suited for forecasting price movements. These models establish relationships between input variables (e.g., historical prices, trading volumes) and the target variable (cryptocurrency price). Linear regression, for example, models the price as a linear function of other variables, while support vector regression aims to find an optimal hyperplane to minimize prediction errors.
Figuring out crypto market trends using data and analytics is key, but understanding market cycles is equally important. Knowing how to spot those ups and downs, like bull and bear markets, is crucial for better investment decisions. This is exactly what Analyzing Market Cycles to Make Better Cryptocurrency Investment Decisions delves into. Ultimately, combining this knowledge with a solid data-driven approach to crypto market analysis is the most effective way to predict future trends.
- Classification models, like logistic regression and random forests, can be used to predict price direction (e.g., uptrend, downtrend). These models assign prices to specific categories based on learned patterns from historical data. For instance, logistic regression models price movements as a probability of falling into a specific category.
- Time series models, such as ARIMA (Autoregressive Integrated Moving Average) and LSTM (Long Short-Term Memory), are specifically designed for sequential data like price time series. These models leverage past data to predict future price points, considering the inherent temporal dependencies within the market.
Challenges in Training and Deployment
Building and deploying accurate ML models for crypto price prediction presents several hurdles.
- Data quality and availability. The crypto market is characterized by high volatility and a lack of standardized data. Incomplete or inaccurate data can lead to inaccurate predictions. Ensuring data quality and consistency is essential for reliable model training.
- Model complexity and interpretability. Complex models, while potentially more accurate, can be harder to understand and interpret. This can make it difficult to identify the factors driving predictions and to adjust the model based on new market insights. Therefore, finding a balance between model complexity and interpretability is crucial.
- Real-time data updates and model retraining. Crypto markets are dynamic, and models need to be constantly updated to reflect the latest trends. Efficient real-time data ingestion and model retraining mechanisms are critical to maintain predictive accuracy. This requires sophisticated infrastructure to handle the high volume of data and the rapid rate of change.
Evaluating Model Accuracy
Evaluating the accuracy of ML models for crypto price forecasting is crucial. Several metrics can be used to assess model performance.
- Mean Absolute Error (MAE) and Root Mean Squared Error (RMSE) measure the average difference between predicted and actual prices. Lower values indicate better accuracy.
- R-squared, a statistical measure, indicates how well the model fits the data. Higher R-squared values generally indicate a better fit. However, R-squared alone may not be sufficient to assess predictive power.
- Backtesting and holdout sets are critical. Models should be tested on unseen data to avoid overfitting. Dividing the dataset into training, validation, and testing sets is essential to evaluate the model’s performance on independent data.
Sentiment Analysis and Social Media Signals
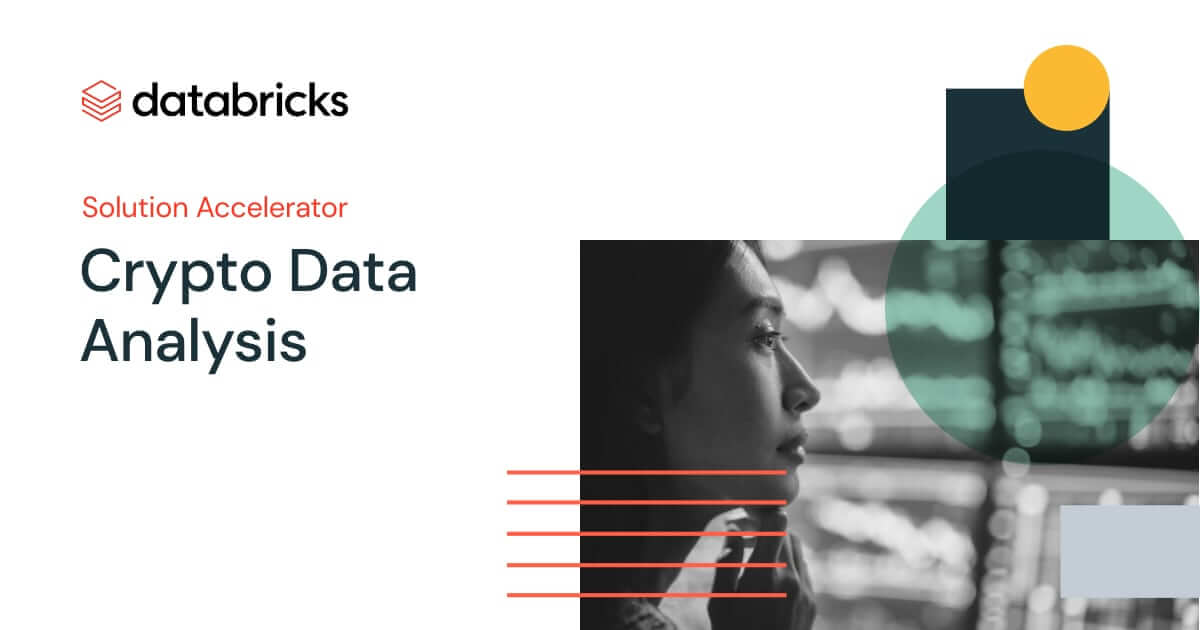
Source: databricks.com
Unveiling the hidden pulse of the crypto market, sentiment analysis offers a powerful tool for deciphering the collective mood surrounding cryptocurrencies. By monitoring social media platforms, we can glean valuable insights into investor sentiment, potentially anticipating market fluctuations. This approach complements traditional data analysis methods, providing a more nuanced understanding of market dynamics.Social media platforms teem with discussions about cryptocurrencies, offering a real-time window into investor opinions.
Analyzing this data can reveal underlying trends, enabling a deeper understanding of market sentiment. This method can prove invaluable in anticipating potential price swings and adapting investment strategies accordingly.
Gathering and Analyzing Social Media Sentiment Data
Understanding the collective sentiment surrounding cryptocurrencies requires a systematic approach to data collection and analysis. This involves scraping data from relevant social media platforms like Twitter, Reddit, and Discord, focusing on posts, comments, and discussions related to specific cryptocurrencies. Tools specifically designed for sentiment analysis can be employed to automatically categorize the sentiment of each post. These tools typically use natural language processing (NLP) algorithms to assess the emotional tone and identify positive, negative, or neutral expressions within the text.
The use of specific s and phrases relevant to the crypto market can further refine the analysis, providing more focused and accurate results.
Interpreting Social Media Sentiment for Market Prediction
A surge in positive sentiment surrounding a particular cryptocurrency can indicate a potential increase in demand, potentially leading to a price increase. Conversely, a prevailing negative sentiment might signal declining interest and potentially lower prices. Analyzing sentiment across various social media platforms offers a broader perspective on market sentiment, enabling a more comprehensive understanding of investor psychology. Monitoring the sentiment shift over time, identifying key turning points, and correlating them with price movements can yield insights into market reactions to news events or developments in the crypto space.
For instance, the announcement of a major new feature for a cryptocurrency project, often accompanied by positive social media buzz, could precede a surge in price. This is a vital tool in identifying potential market shifts and making informed investment decisions.
Categorization of Social Media Sentiment
This table categorizes social media sentiment into positive, negative, and neutral categories, providing a framework for interpreting data.
Sentiment Category | Description | Example Phrases |
---|---|---|
Positive | Expresses approval, enthusiasm, or optimism. | “Amazing project! Huge potential!” “Bullish on this coin.” |
Negative | Expresses disapproval, pessimism, or concern. | “This is a scam!” “Price is going down.” “Horrible project!” |
Neutral | Expresses neither approval nor disapproval. | “This is interesting.” “Checking out the project.” “A bit pricey.” |
Limitations of Using Social Media Data for Market Prediction
Social media data, while valuable, presents limitations in accurately predicting market trends. The sentiment expressed on social media platforms might not always reflect the actual market behavior. Furthermore, the volume of data can be overwhelming, making it difficult to filter out noise and identify meaningful trends. The presence of bots or coordinated campaigns can skew the results, distorting the overall sentiment picture.
Finally, the inherent subjectivity of sentiment analysis algorithms can introduce inaccuracies. It’s crucial to acknowledge and address these limitations when interpreting social media data to avoid misleading predictions and investment decisions.
Predicting Volatility and Risk
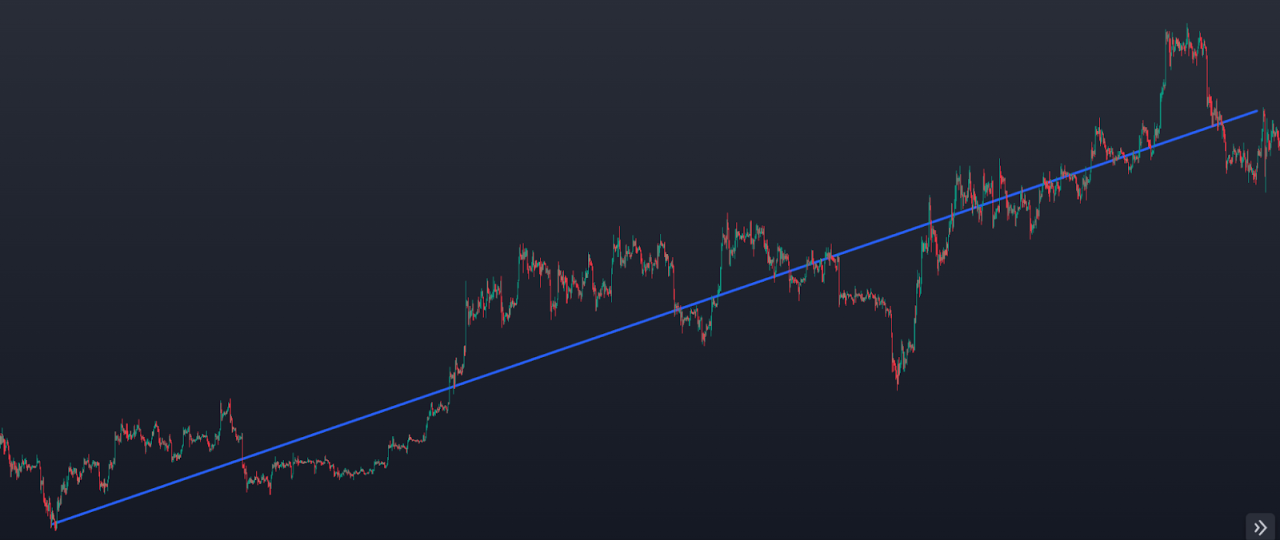
Source: googleusercontent.com
Cryptocurrency markets are notoriously volatile, making accurate prediction of price swings crucial for informed investment decisions. Understanding the factors driving this volatility and developing methods to quantify and model the associated risk are essential steps in creating a robust investment strategy. This section explores techniques for assessing and managing the risk inherent in crypto investments using data-driven approaches.
Factors Contributing to Crypto Market Volatility
Several interconnected factors contribute to the high volatility of cryptocurrency markets. These include rapid shifts in investor sentiment, regulatory uncertainty, news events impacting specific projects, and the inherent speculative nature of the asset class. Market sentiment, often influenced by social media trends and news coverage, can rapidly change, leading to sharp price movements. Regulatory developments, both positive and negative, can significantly impact investor confidence and market liquidity.
Understanding how to predict crypto market trends using data and analytics is crucial, but equally important is knowing the best practices for safe trading. After all, even the most accurate predictions won’t help if you’re not trading responsibly. For example, you should always be mindful of the essential guidelines for safe trading on cryptocurrency exchanges today, like Essential Guidelines for Safe Trading on Cryptocurrency Exchanges Today , to minimize risks.
Ultimately, combining in-depth market analysis with secure trading practices is key to navigating the volatile crypto landscape effectively.
News regarding specific projects, including security breaches, controversies, or partnerships, can cause dramatic price fluctuations. The speculative nature of cryptocurrencies, with its decentralized and often opaque structure, can magnify the impact of these factors, resulting in periods of extreme price swings.
Quantifying and Modeling Crypto Investment Risk
Quantifying risk involves assessing the potential for losses in a cryptocurrency investment. This often involves analyzing historical price data, identifying periods of high volatility, and using statistical models to estimate the probability of different outcomes. Methods include calculating volatility measures such as standard deviation and beta, which reflect the degree of price fluctuations and the asset’s sensitivity to market movements.
Furthermore, value at risk (VaR) analysis can be employed to determine the potential maximum loss over a specific time horizon with a given confidence level. These techniques help investors understand the potential downside of their investments.
Strategies for Managing Investment Risk
Effective risk management strategies are essential when using predictive models. Diversification across different cryptocurrencies and asset classes can reduce the impact of adverse movements in a single project. Stop-loss orders, which automatically sell a position if the price falls below a certain threshold, can help limit potential losses. Position sizing is crucial, allocating a portion of one’s portfolio to crypto investments to match the level of risk tolerance.
Implementing hedging strategies, using derivatives or other instruments, can help mitigate risk. Regular monitoring and re-evaluation of the investment strategy are critical to adapting to changing market conditions.
Examples of Volatility Prediction Models
Various models can be used to predict cryptocurrency volatility. One example is the GARCH (Generalized Autoregressive Conditional Heteroskedasticity) model, which accounts for time-varying volatility. It considers the conditional variance of past returns to predict future volatility. Other models, like stochastic volatility models, also incorporate time-varying volatility components. These models can be used to generate volatility forecasts and inform decisions on asset allocation, position sizing, and risk management strategies.
These models are not guaranteed to be perfectly accurate, but they provide a more informed and data-driven approach to understanding and managing risk in the crypto market.
Volatility Prediction Using Machine Learning
Machine learning models can also be employed to predict cryptocurrency volatility. Models like recurrent neural networks (RNNs) and long short-term memory (LSTM) networks can analyze historical price data, trading volume, and other relevant factors to forecast future volatility. These models can identify complex patterns and relationships that might not be apparent using traditional statistical methods. The results from these models can be integrated with other predictive techniques to create a more comprehensive approach to predicting and managing risk in the crypto market.
Combining Data Sources for Enhanced Predictions
Predicting crypto market trends is a complex task, requiring a multifaceted approach. Simply relying on one data source, like technical indicators alone, often leads to incomplete and potentially inaccurate conclusions. Combining multiple data sources, however, can significantly improve the accuracy of predictions by capturing a more comprehensive picture of the market’s dynamics. This approach leverages the strengths of each data type to mitigate the weaknesses of individual sources, ultimately resulting in more reliable forecasts.Effective predictions necessitate not just the collection of diverse data but also the rigorous processing and integration of these data streams.
This involves meticulous data cleansing, preprocessing, and transformation to ensure consistency and accuracy. The integration process aims to identify correlations, patterns, and anomalies across different data sets, ultimately leading to more informed predictions.
Methods for Integrating Data Sources
Data integration is a critical step in building robust crypto market prediction models. Combining various data sources – technical, fundamental, and sentiment – enhances the predictive power by providing a more holistic view of the market. Different methods exist for combining these diverse data sets. One approach involves weighting the contributions of each data source based on its historical performance or the specific characteristics of the cryptocurrency being analyzed.
Another method involves developing a composite indicator that synthesizes information from multiple sources. A third method is using machine learning models to learn relationships between different data types and generate predictions based on these combined insights.
Data Cleansing and Preprocessing
Accurate model building hinges on clean and well-prepared data. Data cleansing involves identifying and correcting errors, inconsistencies, and missing values within each data source. Preprocessing techniques such as normalization, standardization, and feature engineering transform data into a suitable format for machine learning models. For instance, normalization scales numerical features to a specific range, while standardization centers and scales them to have zero mean and unit variance.
These steps ensure that all data sources contribute effectively to the prediction process.
Combining Technical, Fundamental, and Sentiment Data
A crucial step in enhancing crypto market predictions is integrating technical, fundamental, and sentiment data. Technical indicators, like moving averages and RSI, provide insights into short-term price movements. Fundamental analysis assesses the underlying value of a cryptocurrency project, considering factors such as its team, technology, and market demand. Sentiment analysis, drawing on social media and news data, gauges public opinion and market mood.
By combining these data types, a more comprehensive picture of market dynamics emerges, revealing correlations that might be missed by relying on a single source.
Workflow for Merging Diverse Data Sources
A structured workflow is essential for effectively merging diverse data sources. This process begins with the collection of relevant data from various sources, including exchanges, news platforms, and social media. Next, the collected data is cleaned and preprocessed to handle missing values, inconsistencies, and outliers. Then, the different data types are transformed into a common format, allowing for seamless integration.
Finally, the preprocessed data is combined into a single dataset. Machine learning models can then be trained on this combined dataset to generate accurate predictions. This workflow ensures the data is consistent, complete, and suitable for model training.Example: Consider Bitcoin. Technical analysis might suggest an upcoming price increase. Fundamental analysis might indicate a surge in adoption and usage.
Sentiment analysis reveals a positive market mood. Combining these data points through a predictive model could lead to a more accurate and reliable prediction than using any one data source alone.
Evaluating Prediction Accuracy and Backtesting
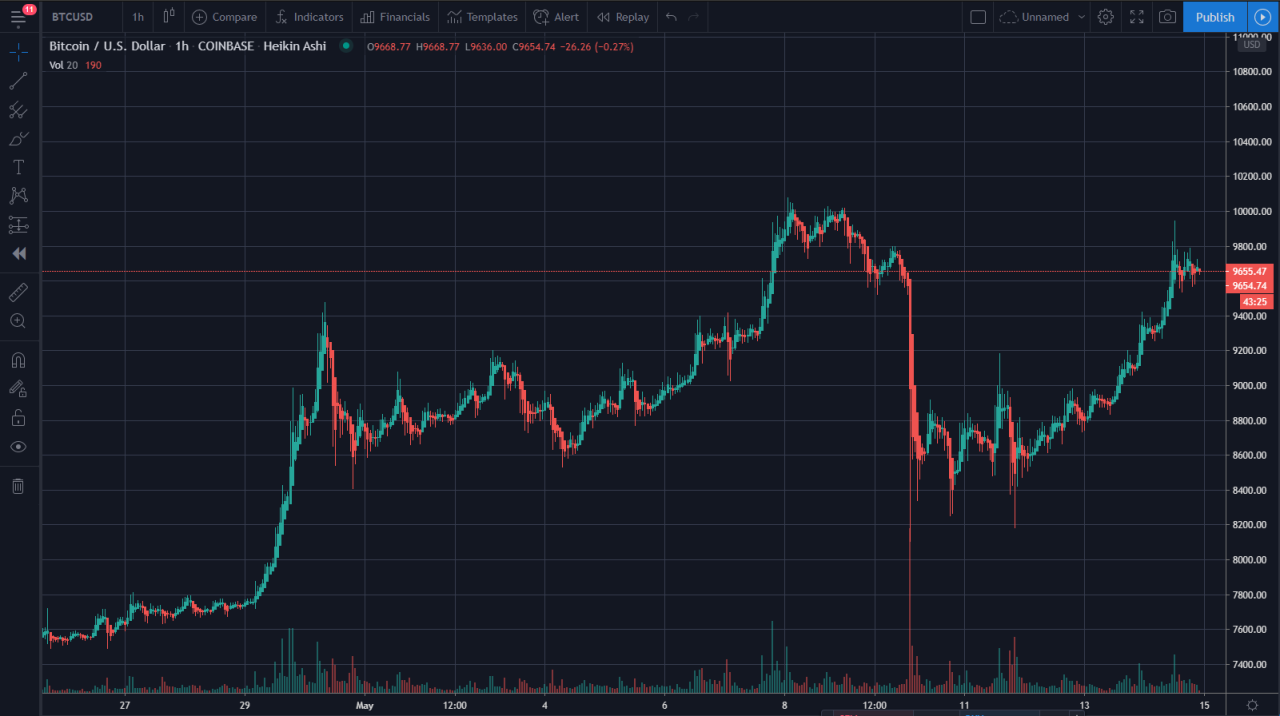
Source: cryptovoid.net
Accurately predicting crypto market trends is a complex endeavor. While data and analytics provide valuable insights, no model is perfect. A crucial step in developing reliable predictive models is rigorous evaluation of their accuracy and a thorough backtesting process. This involves understanding various metrics, testing models against historical data, and adjusting strategies based on observed performance.Evaluating the accuracy of a predictive model is fundamental to its success.
Different evaluation metrics are employed depending on the type of prediction (e.g., direction, magnitude, timing). Choosing appropriate metrics is crucial to a fair and accurate assessment.
Metrics for Evaluating Prediction Accuracy, How to Predict Crypto Market Trends Using Data and Analytics
Understanding the performance of a predictive model requires using appropriate metrics. Common metrics include accuracy, precision, recall, F1-score, and Root Mean Squared Error (RMSE). Accuracy measures the percentage of correct predictions, while precision and recall focus on the quality of positive predictions. RMSE quantifies the average difference between predicted and actual values. Selecting the right metric depends on the specific application and the desired outcome.
For example, if a model is aiming for high precision to identify only the most profitable opportunities, then precision might be a more suitable metric than overall accuracy.
Analyzing data and using analytics is key to predicting crypto market trends. However, understanding external factors like halving events is crucial too. For example, a deep dive into how halving events affect cryptocurrency economics, like those explored in Understanding the Impact of Halving Events on Cryptocurrency Economics , can significantly enhance our predictive models. Ultimately, a combination of technical analysis, fundamental analysis, and understanding of these significant events is vital for successful crypto market trend forecasting.
Backtesting Prediction Models
A critical step in validating predictive models is backtesting. This involves applying the model to historical data to assess its performance in a simulated environment. This allows for the identification of potential biases or weaknesses in the model’s logic and parameters. A systematic approach to backtesting is essential.
- Data Preparation: Carefully prepare the historical data, ensuring it’s complete, accurate, and relevant to the prediction variables. This includes cleaning the data, handling missing values, and transforming variables as necessary. For example, if a model is based on price and volume, make sure both are available for the same time periods.
- Model Application: Apply the model to the historical data, generating predictions for each data point. This step requires the model to be implemented correctly and the parameters properly tuned.
- Result Evaluation: Evaluate the predictions against the actual market outcomes. This involves using the metrics mentioned above to assess the model’s performance and identify potential trends.
Assessing Reliability and Robustness
The reliability and robustness of the results are critical. A model’s performance may be influenced by factors such as market volatility, external events, or specific data biases. Strategies for assessing reliability and robustness include:
- Varying Time Periods: Testing the model across different historical time periods to understand its performance during various market conditions. This ensures the model is not overfitting to a specific time period.
- Sensitivity Analysis: Investigating how the model’s predictions change when input parameters or data sources are altered. This helps determine how sensitive the model is to fluctuations and potential errors.
- Comparing Multiple Models: Testing multiple models and comparing their performances to determine the most effective approach. This could involve different machine learning algorithms or various data combinations.
Adjusting Models Based on Backtesting Results
Backtesting provides insights for model improvement. Identifying weaknesses or biases in the model is essential to make adjustments and refine the predictive capabilities. Adjustments can include:
- Parameter Tuning: Fine-tuning the model’s parameters to improve accuracy and reduce errors. For example, adjusting weights in a weighted average model.
- Feature Engineering: Incorporating new data features or transforming existing ones to enhance the model’s predictive power. This could involve adding indicators or creating new variables from existing ones.
- Model Selection: Replacing or modifying the chosen machine learning algorithm based on its backtesting performance.
End of Discussion
In conclusion, predicting crypto market trends requires a multifaceted approach, combining diverse data sources, technical analysis, fundamental evaluation, and advanced machine learning techniques. By understanding the strengths and weaknesses of each method, you can develop a more comprehensive and reliable predictive strategy. This guide provides a roadmap to navigate the intricacies of the crypto market and make informed decisions, ultimately maximizing your potential for success.
General Inquiries
What are some common pitfalls to avoid when using machine learning models for crypto predictions?
Overfitting to historical data, neglecting the impact of external factors, and not continuously updating models are common pitfalls. Regular backtesting and validation are essential to ensure model accuracy and prevent inaccurate predictions.
How can I ensure the reliability of the data sources I use for analysis?
Verify the credibility and reputation of news outlets, social media platforms, and on-chain data providers. Look for established track records, transparency in data collection methods, and potential biases that could affect the data.
What are the ethical considerations surrounding data usage in crypto market predictions?
Using data responsibly and ethically is crucial. Be mindful of potential biases, privacy concerns, and the potential for manipulation of market trends. Transparency and responsible data handling are paramount.
What is the best way to manage investment risk when using predictive models?
Implement a diversified investment strategy, set realistic risk tolerance levels, and regularly review your investment portfolio. Consider using stop-loss orders and position sizing strategies to mitigate potential losses.