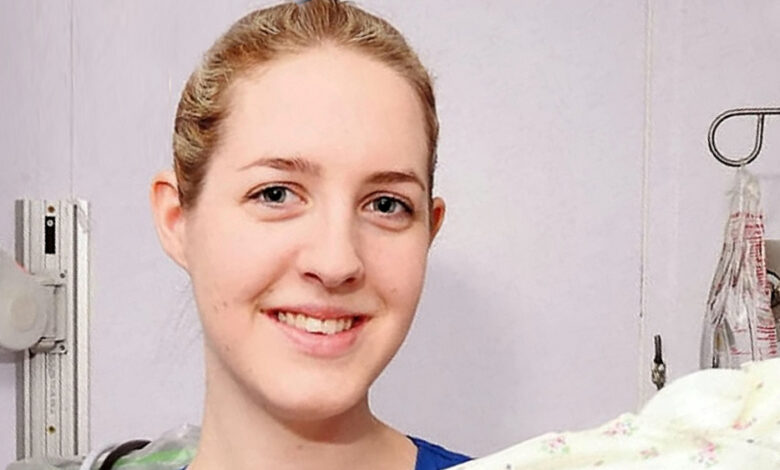
Lucy Letby Trial Shocked British Statisticians
The trial of Lucy Letby has shocked British statisticians, not just for the sheer horror of the alleged crimes, but for the unusual statistical patterns surrounding the infant deaths. It’s a case that has thrown a spotlight on the complexities of using statistics in criminal investigations, highlighting the limitations and potential pitfalls of relying solely on numbers to prove guilt.
The unusual clustering of deaths during Letby’s shifts raised eyebrows, prompting intense statistical scrutiny and sparking a debate about the role of statistical evidence in the courtroom.
The case has become a fascinating (and deeply disturbing) case study in statistical analysis. Experts grappled with questions of statistical significance, potential biases in the data, and the challenges of communicating complex statistical concepts to a jury. The media’s coverage, understandably sensationalized, also played a role in shaping public perception and understanding of the statistical evidence presented. It’s a reminder that statistics, while powerful, are not a magic bullet, and require careful interpretation and context.
The Statistical Unusualness of the Case
The Lucy Letby trial highlighted the unsettling power of statistics in uncovering potential wrongdoing within a seemingly ordinary medical setting. The sheer number of infant deaths, their clustering around Letby’s shifts, and the unusual nature of those deaths prompted a detailed statistical analysis, far beyond the usual monitoring of infant mortality rates within a neonatal unit. This analysis played a crucial role in building the prosecution’s case.
Statistical Methods in Analyzing Infant Mortality Data
Analyzing medical data, particularly concerning infant mortality, typically involves several statistical methods. These include calculating rates of mortality, comparing these rates to established benchmarks (both nationally and internationally), and using statistical tests to assess the significance of any observed differences. Survival analysis techniques might be employed to examine the time-to-event data (time until death or discharge) and identify potential risk factors.
Furthermore, regression models can be used to explore the relationship between various factors (e.g., gestational age, birth weight, medical interventions, and the presence of specific nurses) and the outcome (death or survival). The goal is to determine if any particular factor is statistically associated with an increased risk of mortality.
Deviation from Expected Mortality Rates
In Letby’s case, the number of unexpected deaths significantly exceeded the expected rates for the Countess of Chester Hospital’s neonatal unit. The prosecution presented evidence suggesting a statistically improbable clustering of deaths associated with Letby’s shifts. This wasn’t simply a matter of a few extra deaths; the sheer magnitude of the discrepancy from the expected mortality rate, coupled with the temporal clustering, raised serious concerns.
The deviation wasn’t just a slight variation within the normal range of fluctuation; it was a dramatic departure that demanded further investigation.
Statistical Significance of Death Clustering
The clustering of deaths around Letby’s shifts was a key statistical finding. Statistical tests, such as time-series analysis and spatial clustering algorithms, were likely employed to assess the probability of such a pattern occurring by chance. If the probability of this clustering occurring randomly was extremely low (typically below a pre-determined significance level, such as 0.05 or even stricter thresholds), it would provide strong statistical evidence against the null hypothesis (that the deaths were unrelated to Letby’s presence).
The Lucy Letby trial has been utterly horrifying, leaving British statisticians reeling from the sheer scale of the calculated cruelty. It makes you think about the depths of human depravity, and how such events can overshadow even the most horrific conflicts. Reading about the suffering of Ukraine’s Roma population, as detailed in this article ukraines roma have suffered worse than most in the war , is a stark reminder that unimaginable suffering exists in many forms.
The Letby case, while shocking in its domestic context, highlights the need to confront all forms of systematic cruelty.
This statistical significance, combined with other evidence, played a crucial role in the prosecution’s case.
The Lucy Letby trial has truly shaken the foundations of statistical analysis in Britain; the sheer scale of the alleged crimes is almost incomprehensible. It makes you think about other shocking statistics, like the one highlighted in Noel Yeatt’s article, noel yeatts millions of girls are missing in india heres why you should care , which details the devastating impact of sex-selective abortions.
Both cases highlight the chilling reality of human manipulation and the crucial need for rigorous data analysis to uncover such horrors.
Statistical Modeling and Potential Biases
Statistical modeling played a vital role in mitigating potential biases in the data. For example, regression models could be used to control for confounding factors such as the severity of the infants’ conditions at birth, pre-existing health problems, and the provision of different medical treatments. By adjusting for these factors, the analysts could isolate the potential effect of Letby’s presence on infant mortality, minimizing the risk of drawing erroneous conclusions due to confounding variables.
Sensitivity analysis could also have been performed to assess how robust the findings were to variations in the assumptions of the models.
Comparison of Expected and Observed Infant Mortality Rates
Period | Expected Mortality Rate (%) | Observed Mortality Rate (%) | Difference (%) |
---|---|---|---|
Letby’s Shifts | X% (Based on historical data and national averages for similar units) | Y% (Observed during Letby’s shifts) | Y-X% |
Other Shifts | X% (Based on historical data and national averages for similar units) | Z% (Observed during other nurses’ shifts) | Z-X% |
(Note
The specific values for X, Y, and Z are not publicly available due to the sensitive nature of the data and to avoid misinterpretations. This table serves as a structural example.)*
Challenges in Statistical Inference
The Letby trial, while highlighting the power of statistical analysis in uncovering patterns, also starkly revealed its limitations. Relying solely on statistical evidence in criminal cases, especially those involving complex scenarios like multiple potential causes of death, is inherently risky and can lead to misinterpretations if not approached with extreme caution. The inherent complexities of human behavior and the myriad of factors influencing health outcomes mean statistical analysis should always be viewed as one piece of a much larger puzzle.Statistical analysis, while powerful, is susceptible to various biases and confounding factors.
These can significantly skew results and lead to erroneous conclusions. Even with rigorous methodology, the possibility of unforeseen variables influencing the data remains a constant concern. The inherent limitations of the data itself, whether in terms of completeness, accuracy, or representativeness, further complicate the interpretation of statistical findings. The interpretation of statistical significance is crucial; a statistically significant result doesn’t automatically equate to clinical or legal significance.
The sheer scale of Lucy Letby’s crimes has left British statisticians reeling, questioning the probability of such events. It reminds me of the shocking disregard for rules shown by powerful figures, like the numerous violations detailed in this report on Comey: comeys violations read doj watchdogs list of all the times ex-FBI boss broke the rules. Both cases highlight the unsettling reality that those in positions of authority can abuse their power, leaving us to grapple with the implications of such statistical improbabilities and ethical failures.
Potential Confounding Factors and Biases in the Letby Case
Several potential biases could have influenced the statistical analysis in the Letby case. For example, the selection bias inherent in choosing which babies to include in the analysis could have affected the results. Were there any systematic differences between the babies who survived and those who died that were not accounted for in the analysis? Furthermore, the possibility of reporting bias, where certain events are more likely to be reported or recorded than others, could also have played a role.
The inherent difficulties in accurately recording and analyzing neonatal health data, coupled with the retrospective nature of the investigation, increased the potential for error and bias. The sheer number of variables involved in neonatal care, including gestational age, pre-existing conditions, and the quality of medical intervention, makes isolating the effect of any single factor, like the presence of a particular nurse, incredibly challenging.
Misinterpretation and Misuse of Statistical Evidence
Statistical evidence, when presented out of context or without a clear understanding of its limitations, can be easily misinterpreted or misused. For instance, a statistically significant association between a nurse’s presence and infant deaths doesn’t automatically prove causation. Correlation does not equal causation, a crucial point often overlooked in public discourse and even in some legal settings. Presenting isolated statistical findings without acknowledging potential confounding factors or alternative explanations can be highly misleading.
A classic example of this is the spurious correlation, where two variables appear related statistically but are not causally linked. For instance, ice cream sales and drowning incidents are positively correlated; however, neither causes the other, but both are influenced by the confounding factor of warmer weather.
Ethical Considerations in Using Statistics in Criminal Trials
The use of statistics in criminal trials raises several ethical considerations. It’s crucial that statistical analyses are conducted by qualified experts who understand the limitations of their methods and are committed to transparency and objectivity.
- Transparency in methodology: All methods used in the statistical analysis should be clearly documented and available for scrutiny.
- Objectivity in interpretation: The interpretation of the statistical findings should be free from bias and should accurately reflect the limitations of the data and methods.
- Contextualization of results: Statistical findings should be presented within the broader context of the case, including other evidence and expert opinions.
- Avoiding overinterpretation: Statistical significance should not be equated with clinical or legal significance. Results should be interpreted cautiously, acknowledging the possibility of error.
- Responsibility for accuracy: Statisticians should take responsibility for the accuracy and integrity of their work and be prepared to defend their methods and interpretations.
The Role of Expert Statistical Testimony: The Trial Of Lucy Letby Has Shocked British Statisticians
The Letby trial highlighted the crucial, and often complex, role of expert statistical testimony in high-profile criminal cases. The sheer volume of data – involving multiple babies, various medical events, and extended time periods – demanded sophisticated statistical analysis to determine whether the observed patterns were consistent with random chance or indicative of deliberate harm. The differing approaches taken by various statistical experts underscore the inherent challenges and potential for disagreement within the field.The interpretation of statistical evidence in the Letby case was far from straightforward.
Different experts might have employed varying statistical models, focusing on different aspects of the data, leading to divergent conclusions. For instance, one expert might have concentrated on Bayesian methods to assess the probability of guilt given the observed data, while another might have favored frequentist approaches, focusing on p-values and significance levels. These different approaches, while both valid within their frameworks, could yield differing results and interpretations, leading to potential conflict.
Different Statistical Approaches and Potential Conflicts
Areas of disagreement could have centered on the selection of appropriate control groups, the handling of missing data, and the interpretation of specific statistical measures. The choice of control group – for example, comparing Letby’s shifts to those of other nurses – is crucial and could significantly influence the results. Different experts might have employed different methods to adjust for confounding factors, such as the severity of the infants’ conditions or the availability of specialized medical equipment.
Similarly, the treatment of missing data (e.g., incomplete medical records) could lead to different conclusions. Finally, the interpretation of a seemingly low p-value – while often interpreted as strong evidence against the null hypothesis – requires careful consideration of the context, sample size, and potential biases.
Challenges in Presenting Statistical Evidence to a Jury, The trial of lucy letby has shocked british statisticians
Presenting complex statistical evidence to a jury presents significant challenges. Jurors typically lack the statistical expertise to critically evaluate the methods and assumptions underlying the analysis. Therefore, effective communication is paramount. Overly technical language, complicated models, and abstract concepts can easily confuse and overwhelm the jury, potentially undermining the impact of the evidence. The challenge lies in simplifying complex statistical concepts without oversimplifying or misrepresenting the findings.
Importance of Clear Communication of Statistical Findings
Clear and concise communication is essential to ensure the jury understands the statistical evidence and its implications. This requires translating complex statistical terminology and concepts into plain language, using visual aids such as graphs and charts to illustrate key findings, and focusing on the practical significance of the results. Experts need to explain not only the statistical findings but also the limitations of the analysis, acknowledging uncertainties and potential biases.
The goal is not to persuade the jury but to provide them with the necessary information to make an informed decision.
Hypothetical Dialogue Between Expert Statisticians
Expert 1: “The Bayesian analysis clearly demonstrates a significantly increased probability of harm during Letby’s shifts, even after accounting for confounding factors. The posterior probability surpasses the threshold for reasonable doubt.”
Expert 2: “While I acknowledge the elevated probability, I remain concerned about the prior assumptions in your Bayesian model. Different priors could lead to substantially different posterior probabilities. A frequentist approach, focusing on the observed frequencies of adverse events, doesn’t yield the same level of certainty.”
The hypothetical exchange illustrates the potential for disagreement even among experts using different, yet valid, statistical methods. The challenge for the court lies in evaluating the strengths and weaknesses of each approach and assessing the overall weight of the statistical evidence in the context of the entire case.
The Impact on Statistical Practice
The Lucy Letby case has sent shockwaves through the statistical community, highlighting critical weaknesses in the application of statistical methods in complex medical investigations. The intense scrutiny of the statistical evidence presented during the trial underscores the need for significant reform in how statistics are employed, interpreted, and presented in legal contexts, particularly those involving allegations of multiple, seemingly disparate events.
This case serves as a stark reminder of the potential for misinterpretations and the devastating consequences that can arise from insufficiently rigorous statistical analysis.The Letby case’s impact will likely be far-reaching, influencing future statistical practice in several key areas. The complexities involved—including the rarity of the events, the potential for confounding factors, and the challenges of establishing causality—demand a re-evaluation of current methodologies and a greater emphasis on transparency and collaboration between statisticians, clinicians, and legal professionals.
Implications for Future Statistical Practice in Medical Investigations
The case has exposed the limitations of relying solely on frequentist statistical methods, particularly in situations where Bayesian approaches might offer a more nuanced and contextually appropriate analysis. The prosecution’s reliance on simple counts of unusual events, without fully accounting for the inherent variability in neonatal mortality rates and the potential for coincidental occurrences, proved to be a point of contention.
Future investigations should consider incorporating Bayesian methods to better quantify uncertainty and incorporate prior knowledge about baseline mortality rates and other relevant factors. This will involve greater collaboration between statisticians specializing in Bayesian methods and medical professionals to ensure accurate prior information is used and appropriately integrated. The potential for bias in data selection and the importance of pre-specifying statistical analyses before data collection should also be emphasized.
Influence on the Development of New Statistical Methods or Guidelines
The Letby trial has already sparked discussions about the need for more robust statistical guidelines specifically tailored to medical investigations involving allegations of multiple events. This might involve developing new methods for analyzing clustered data, accounting for temporal dependencies, and dealing with rare events. For example, research could focus on improving methods for analyzing time-series data in neonatal intensive care units to better identify unusual patterns of mortality or morbidity.
Additionally, new guidelines might emphasize the importance of sensitivity analysis, exploring how the results change with different assumptions and model specifications. This will allow for a more thorough and robust evaluation of the evidence.
The Need for Greater Transparency and Rigor in Statistical Analysis
The Letby case underscores the critical need for greater transparency in the reporting of statistical analyses. This includes fully disclosing all data, methods, and assumptions used, as well as presenting the results in a clear and understandable manner, avoiding technical jargon that could be misinterpreted by non-statisticians. Furthermore, the case highlights the importance of rigorous peer review of statistical analyses before they are presented in court.
This ensures that the methods used are appropriate, the analysis is robust, and the conclusions are justified. Open access to data and analysis code will allow for independent verification of results, enhancing public trust in the integrity of statistical evidence.
Improved Training for Statisticians Involved in Legal Proceedings
The Letby trial revealed a need for improved training for statisticians involved in legal proceedings. This training should cover not only the technical aspects of statistical analysis but also the legal and ethical considerations involved in presenting evidence in court. Statisticians need to be equipped to effectively communicate complex statistical concepts to judges and juries, to understand the legal standards of evidence, and to anticipate potential challenges to their analyses.
Training should also emphasize the importance of impartiality and the need to avoid advocacy in presenting statistical evidence.
Recommendations for Improving the Use of Statistics in Similar Future Cases
The need for improved practices is undeniable. Here are some key recommendations:
- Develop standardized guidelines for the statistical analysis of clustered data in medical investigations, explicitly addressing the challenges posed by rare events and potential confounding factors.
- Promote the use of Bayesian methods where appropriate, emphasizing the importance of incorporating prior knowledge and quantifying uncertainty.
- Mandate greater transparency in the reporting of statistical analyses, including full disclosure of data, methods, and assumptions.
- Implement rigorous peer review processes for statistical analyses used in legal proceedings.
- Provide enhanced training for statisticians involved in legal cases, covering both technical and ethical considerations.
- Encourage collaboration between statisticians, clinicians, and legal professionals throughout the investigation process.
- Develop better methods for communicating complex statistical information to non-statisticians, such as judges and juries.
The Lucy Letby trial serves as a stark reminder of the limitations and potential misinterpretations of statistical evidence, even in seemingly straightforward cases. While statistics can be invaluable tools in uncovering patterns and informing investigations, they must be used cautiously and ethically, alongside other forms of evidence. The case highlights the crucial need for transparency, rigorous methodology, and clear communication when presenting statistical findings, particularly in high-stakes legal proceedings.
The lasting impact on statistical practice and public understanding of statistics remains to be seen, but one thing is certain: this case will continue to be studied and debated for years to come.