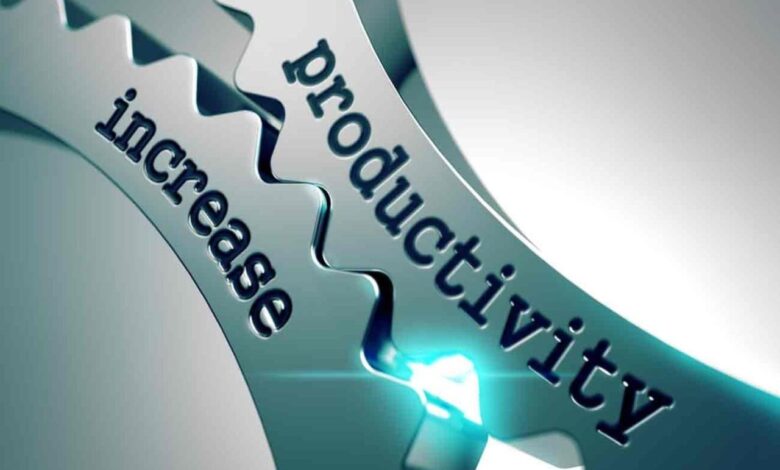
There Will Be No Immediate Productivity Boost From AI
There will be no immediate productivity boost from AI. That’s the inconvenient truth many businesses are struggling to accept. While the hype surrounding artificial intelligence promises a revolution in efficiency, the reality is far more nuanced. A significant return on investment requires careful planning, substantial upfront investment, and a realistic understanding of AI’s capabilities and limitations. This isn’t about dismissing AI’s potential; it’s about managing expectations and avoiding the pitfalls of premature adoption.
The allure of instant productivity gains from AI is strong. Marketing materials often paint a picture of effortless efficiency, where AI magically solves all problems. However, this overlooks the crucial preconditions necessary for successful AI integration, including high-quality data, robust infrastructure, and a skilled workforce capable of managing and interpreting AI’s outputs. We’ll explore the challenges, the necessary steps for successful implementation, and the long-term potential that AI truly holds.
The Myth of Immediate AI Productivity Gains
The integration of artificial intelligence into various sectors is often touted as a revolutionary productivity booster, promising immediate and substantial improvements. However, this narrative often overlooks the complex realities of AI implementation and the time required to see tangible returns on investment. The truth is, realizing significant productivity gains from AI is a journey, not a sprint.
Factors Contributing to Overestimation of Short-Term AI Benefits
Several factors contribute to the inflated expectations surrounding AI’s immediate impact on productivity. One major factor is the hype surrounding AI’s potential, often fueled by marketing and media portrayals that showcase idealized scenarios. This creates an unrealistic expectation of quick wins, neglecting the considerable time, resources, and expertise required for successful AI integration. Another factor is the difficulty in accurately measuring AI’s impact.
Let’s be real, folks, expecting an overnight productivity revolution from AI is a bit naive. The hype is real, but the reality is more nuanced. Take, for instance, the current legal battle unfolding in Arizona, where, as reported in this article arizona attorney general candidate sues over midterm election results , legal processes are still very much human-driven.
This highlights that while AI might assist, significant systemic changes take time; similarly, a true productivity boost from AI won’t happen overnight.
Quantifying productivity improvements can be challenging, particularly when dealing with complex systems and intertwined processes. Furthermore, the lack of standardized metrics across different industries makes comparing results and drawing meaningful conclusions difficult. Finally, successful AI implementation requires substantial investment in infrastructure, data acquisition, and skilled personnel – a commitment often underestimated in initial projections.
Examples of Industries with Slower-Than-Expected AI Adoption
While AI has demonstrated remarkable potential in certain niche applications, its broader adoption across many industries has been slower than initially anticipated. In the healthcare sector, for example, the implementation of AI-powered diagnostic tools has faced challenges related to data privacy, regulatory hurdles, and the need for extensive validation and testing before widespread deployment. Similarly, in the manufacturing sector, the integration of AI-driven automation systems has often been hampered by legacy infrastructure, the need for extensive retraining of personnel, and the unforeseen complexities of adapting existing workflows to accommodate new technologies.
The financial sector, while showing some progress in areas like fraud detection, still struggles with the ethical considerations and regulatory compliance associated with AI-driven decision-making.
Comparison of Anticipated vs. Actual AI Productivity Boosts, There will be no immediate productivity boost from ai
Industry | Anticipated Productivity Boost (within 1 year) | Actual Productivity Boost (within 1 year) | Reasons for Discrepancy |
---|---|---|---|
Manufacturing | 20-30% increase in efficiency | 5-10% increase in efficiency | Integration challenges, retraining needs, unforeseen downtime |
Healthcare | 15-25% reduction in diagnostic errors | 5-10% reduction in diagnostic errors | Data quality issues, regulatory hurdles, validation processes |
Finance | 10-20% reduction in operational costs | 2-5% reduction in operational costs | Data security concerns, compliance requirements, model interpretability |
Customer Service | 30-40% improvement in customer satisfaction | 10-15% improvement in customer satisfaction | Integration with existing systems, need for human oversight |
The Necessary Preconditions for AI Productivity: There Will Be No Immediate Productivity Boost From Ai
Let’s be clear: AI isn’t a magic bullet. While the potential for increased productivity is undeniable, realizing that potential requires careful planning and significant upfront investment. Ignoring the necessary preconditions will almost certainly lead to disappointment and wasted resources. Successful AI implementation hinges on a robust foundation built on several key pillars.The successful integration of AI into any organization demands a multi-faceted approach, going far beyond simply purchasing the latest software.
It’s about building a comprehensive ecosystem capable of supporting, training, and deploying AI models effectively. This ecosystem requires substantial investment in infrastructure, data management, and skilled personnel.
Data Quality and Management
High-quality data is the lifeblood of any AI system. Garbage in, garbage out remains a fundamental truth. AI models are only as good as the data they are trained on. This means investing in robust data cleaning, validation, and annotation processes. Inconsistencies, inaccuracies, and biases in the data will directly translate into flawed AI outputs, rendering the entire project ineffective.
A robust data management system, capable of handling large datasets, ensuring data security, and facilitating easy access for AI models, is crucial. This includes implementing data governance policies and establishing clear data ownership and accountability. Without this foundation, even the most sophisticated AI algorithms will struggle to deliver meaningful results.
Computational Resources
Training and deploying complex AI models requires significant computational power. This often translates to investing in powerful hardware, such as GPUs (Graphics Processing Units) and specialized AI accelerators. Cloud computing platforms can offer scalable solutions, allowing organizations to adjust their computational resources based on their needs. However, even with cloud solutions, careful planning is essential to ensure sufficient capacity to handle the computational demands of the AI workload.
Underestimating these requirements can lead to significant delays and increased costs. Choosing the right cloud provider and optimizing the AI model architecture are critical aspects of this process.
Let’s be real, folks, expecting an immediate productivity explosion from AI is a bit naive. The hype is real, but the reality is more nuanced. Consider this: the government’s decision to extend the COVID-19 health emergency until next year, as reported in this article , highlights the ongoing challenges we face. These kinds of disruptions, and the adaptations required, impact productivity far more than any immediate AI gains.
So, while AI is exciting, let’s manage our expectations.
Skilled Personnel
AI implementation isn’t just a technological challenge; it’s a human one as well. Organizations need to invest in recruiting and training personnel with the necessary skills to develop, deploy, and maintain AI systems. This includes data scientists, machine learning engineers, and AI ethicists. Furthermore, existing employees need to be trained to effectively use and interact with AI tools.
A lack of skilled personnel can hinder the entire process, leading to project delays, poor model performance, and ultimately, a failure to achieve the desired productivity gains. Investing in upskilling and reskilling programs is crucial for ensuring a smooth transition and maximizing the benefits of AI.
Let’s be realistic, folks – AI isn’t a magic wand waving away our productivity woes. It’ll take time and integration. Think about the struggles faced by businesses like those in North Carolina, who are still fighting for compensation after pandemic closures, as reported here: north carolina business owners request compensation for pandemic closures. Their recovery shows how significant change requires sustained effort, and the same applies to seeing real results from AI implementation.
We need patience and strategic planning, not quick fixes.
Examples of Successful AI Integration
Several companies have successfully navigated these preconditions and reaped substantial productivity gains. For example, Netflix uses AI for personalized content recommendations, significantly improving user engagement and retention. This success relies on their vast data infrastructure, skilled data scientists, and robust algorithms. Similarly, Amazon leverages AI extensively in its logistics and supply chain management, optimizing delivery routes and warehouse operations.
Their success is a direct result of a massive investment in data infrastructure, sophisticated AI models, and a highly skilled workforce.
Steps to Prepare for AI Implementation
Preparing for successful AI implementation requires a structured approach. Organizations should consider the following steps:
- Assess current data infrastructure and identify gaps: Evaluate the quality, accessibility, and security of existing data. Determine what improvements are needed to support AI initiatives.
- Develop a clear AI strategy aligned with business goals: Define specific, measurable, achievable, relevant, and time-bound (SMART) goals for AI implementation. This ensures that AI projects deliver tangible value.
- Invest in necessary infrastructure and personnel: Acquire or upgrade hardware, software, and cloud resources. Recruit and train personnel with the necessary AI skills.
- Pilot AI projects to test and refine processes: Begin with smaller, focused projects to gain experience and identify potential challenges before scaling up.
- Establish robust data governance and security protocols: Implement measures to ensure data quality, security, and compliance with relevant regulations.
- Continuously monitor and evaluate AI performance: Track key metrics to assess the effectiveness of AI systems and make necessary adjustments.
The Role of Human-AI Collaboration in Productivity
The integration of AI into the workplace isn’t about replacing humans; it’s about augmenting human capabilities. While AI can automate repetitive tasks and analyze vast datasets, true productivity gains stem from a synergistic partnership between human ingenuity and artificial intelligence. Successfully navigating this collaboration requires careful consideration of existing workflows, a clear understanding of AI’s limitations, and a strategic approach to integrating AI tools effectively.The successful implementation of AI tools requires careful planning and execution.
Simply introducing AI software without considering its integration into established workflows is a recipe for failure. Existing processes may need to be redesigned to leverage AI’s strengths while mitigating its weaknesses. Furthermore, human oversight remains crucial to ensure accuracy, ethical considerations are addressed, and unexpected outcomes are managed. Without this critical human element, the potential for errors, biases, and unintended consequences increases significantly.
Challenges of Integrating AI into Existing Workflows
Integrating AI into existing workflows presents several significant challenges. One key obstacle is the need for data compatibility. AI models often require specific data formats and structures, which may necessitate substantial data cleaning, transformation, and integration efforts. Another challenge lies in the potential for disruption to established processes. Introducing AI tools can require retraining employees, altering communication channels, and adjusting team dynamics.
Furthermore, ensuring data security and privacy in the context of AI implementation is paramount. This requires careful consideration of data governance policies and compliance with relevant regulations. Finally, managing the expectations surrounding AI’s capabilities is vital. Overselling AI’s potential can lead to disappointment and hinder its successful adoption.
Models of Human-AI Collaboration
Several models of human-AI collaboration exist, each with its own strengths and weaknesses. One model is the human-in-the-loop approach, where humans actively participate in the AI’s decision-making process, providing feedback and oversight. This model is particularly suitable for tasks requiring judgment, critical thinking, or ethical considerations. A contrasting model is the AI-assisted approach, where AI tools automate routine tasks, freeing up human workers to focus on more complex and creative endeavors.
This model is well-suited for repetitive or data-heavy tasks. A third model, the collaborative approach, involves humans and AI working in tandem, leveraging each other’s strengths to achieve a common goal. This approach often involves AI performing preliminary analysis, while humans provide the final interpretation and decision-making. The optimal model depends on the specific task and the organizational context.
Tasks Where Human Expertise Remains Irreplaceable
Even with the advancements in AI, several tasks remain firmly within the realm of human expertise. These include tasks requiring complex problem-solving, critical thinking, creativity, emotional intelligence, and nuanced judgment. For example, a surgeon performing a delicate operation, a lawyer arguing a complex case, or a teacher mentoring a student all require a level of human intuition and adaptability that current AI systems cannot replicate.
Similarly, tasks requiring ethical decision-making, such as judging the fairness of a loan application or determining the appropriate sentence for a criminal, often necessitate human judgment and understanding of social context.
Job Role Adaptation in the Age of AI
Job Role | Current Tasks | AI-Augmented Tasks | Future Evolution |
---|---|---|---|
Data Analyst | Data cleaning, analysis, reporting | AI-powered data exploration, anomaly detection, predictive modeling | Focus on strategic insights, model interpretation, and data governance |
Customer Service Representative | Handling customer inquiries, resolving issues | AI-powered chatbots for initial support, automated responses | Focus on complex issue resolution, personalized interactions, and relationship building |
Marketing Manager | Campaign planning, execution, analysis | AI-powered audience segmentation, ad targeting, campaign optimization | Focus on creative strategy, brand building, and customer experience |
Software Engineer | Coding, testing, debugging | AI-assisted code generation, automated testing, bug detection | Focus on complex system design, architecture, and AI model development |
The Long-Term Potential of AI for Productivity Enhancement
While the immediate impact of AI on productivity might be less dramatic than initially hyped, the long-term potential is undeniably transformative. We’re at the foothills of a technological revolution, and the coming decades will see AI reshape industries and redefine how we work, leading to unprecedented productivity gains. This isn’t just about incremental improvements; it’s about a fundamental shift in how we approach tasks and solve problems.AI’s power lies in its ability to handle complex processes beyond human capabilities, automating tasks that are repetitive, time-consuming, or require immense data processing.
This automation frees up human workers, allowing them to focus on higher-level strategic thinking, creative problem-solving, and building stronger relationships with clients and colleagues – tasks that require uniquely human skills and emotional intelligence. The result is a more efficient, effective, and ultimately, more fulfilling work environment.
AI-Driven Automation of Complex Processes
The automation potential of AI extends far beyond simple, repetitive tasks. Consider the legal profession, where AI is being used to analyze vast amounts of legal documents, identifying relevant precedents and clauses with far greater speed and accuracy than human lawyers. This frees up lawyers to focus on legal strategy, client interaction, and the creative aspects of their work.
Similarly, in manufacturing, AI-powered robots are not only performing repetitive assembly tasks but also performing predictive maintenance, optimizing production lines in real-time, and identifying potential defects before they occur – leading to significant reductions in downtime and waste. This increased efficiency translates directly to higher productivity and lower costs.
Examples of Emerging AI Technologies Revolutionizing Industries
Several emerging AI technologies promise to revolutionize various sectors. Generative AI, for instance, is already impacting creative industries, helping designers generate novel designs, writers produce compelling content, and musicians compose unique melodies. This technology accelerates the creative process and allows human artists to explore new possibilities. In healthcare, AI-powered diagnostic tools are improving the accuracy and speed of disease detection, leading to earlier interventions and better patient outcomes.
The application of AI in personalized medicine is also rapidly advancing, allowing for the development of tailored treatments based on an individual’s genetic makeup and medical history. In finance, AI algorithms are used for fraud detection, risk assessment, and algorithmic trading, improving efficiency and reducing financial losses.
A Timeline of AI Adoption and its Impact on Productivity
Predicting the exact timeline is challenging, but we can envision a phased adoption of AI across different sectors.
Phase | Time Horizon | Impact on Productivity | Examples |
---|---|---|---|
Early Adoption (Pilot Projects) | 2024-2027 | Incremental improvements in specific tasks | AI-powered chatbots for customer service, automated data entry in finance |
Widespread Adoption (Industry-Specific Solutions) | 2028-2032 | Significant productivity gains in specific sectors | AI-driven automation in manufacturing, personalized medicine in healthcare |
Transformative Adoption (Cross-Industry Integration) | 2033-2040 | Fundamental shift in workflows and business models | Generative AI impacting creative industries, autonomous vehicles transforming logistics |
It’s important to note that this timeline is an estimation, and the actual pace of adoption will vary depending on technological advancements, regulatory frameworks, and societal acceptance. However, the long-term potential for AI to dramatically enhance productivity across all sectors is undeniable. The key will be in effectively integrating AI into existing workflows, fostering human-AI collaboration, and addressing ethical considerations to ensure a positive and equitable impact.
Addressing the Challenges of AI Integration
Successfully integrating AI into a business isn’t a simple switch-flip. It requires careful planning, substantial investment, and a proactive approach to addressing the inevitable hurdles. Ignoring these challenges can lead to wasted resources, failed implementations, and even damage to company reputation. Understanding and mitigating these obstacles is crucial for reaping the long-term benefits of AI.The path to successful AI integration is often paved with challenges.
These range from cultural resistance within the organization to significant skill gaps and complex ethical considerations. Overcoming these requires a multi-faceted strategy encompassing change management, upskilling initiatives, and a robust ethical framework. Companies that succeed prioritize clear communication, employee training, and a commitment to responsible AI development and deployment.
Resistance to Change and Cultural Adaptation
Successfully implementing AI requires overcoming inherent resistance to change. Employees may fear job displacement, struggle to adapt to new technologies, or simply be uncomfortable with the unfamiliar. Addressing this requires open and honest communication. Transparency about the goals of AI integration, how it will impact roles, and the opportunities it presents can alleviate fears. Furthermore, providing comprehensive training and support ensures employees feel equipped to work alongside AI, fostering a culture of collaboration rather than competition.
Companies like Google, for example, have invested heavily in reskilling programs to prepare their workforce for the AI-driven future. They emphasize continuous learning and adaptation, making the transition smoother and less threatening for employees.
Skill Gaps and Talent Acquisition
AI integration necessitates a workforce with the necessary skills to develop, implement, and maintain AI systems. This often translates into a significant skill gap, requiring organizations to invest in training existing employees and recruiting new talent. Strategies include partnerships with educational institutions, online learning platforms, and specialized training programs focused on AI and machine learning. Companies like IBM have successfully bridged this gap by creating internal AI academies and fostering a culture of continuous learning, equipping their employees with the necessary expertise.
This proactive approach not only addresses immediate skill gaps but also ensures the organization remains at the forefront of AI innovation.
Ethical Considerations and Responsible AI
The ethical implications of AI are increasingly important. Bias in algorithms, data privacy concerns, and the potential for job displacement require careful consideration. Companies must establish clear ethical guidelines for AI development and deployment, ensuring fairness, transparency, and accountability. This involves rigorous testing for bias, implementing robust data security measures, and establishing mechanisms for addressing ethical concerns.
Companies like Microsoft have made significant strides in promoting responsible AI, emphasizing transparency, fairness, and inclusivity in their AI systems. They have actively invested in research and development to mitigate potential biases and ensure the ethical use of their technology.
A Flowchart for Addressing AI Integration Challenges
The following flowchart Artikels the key steps involved in successfully addressing the challenges of AI integration:[Imagine a flowchart here. It would begin with “Identify Challenges” branching to “Resistance to Change,” “Skill Gaps,” and “Ethical Concerns.” Each branch would then lead to a series of actions: For “Resistance to Change,” actions could include “Open Communication,” “Training Programs,” and “Change Management Strategies.” For “Skill Gaps,” actions could include “Upskilling Initiatives,” “Talent Acquisition,” and “Partnerships with Educational Institutions.” For “Ethical Concerns,” actions could include “Establish Ethical Guidelines,” “Bias Mitigation,” and “Data Privacy Measures.” All branches would ultimately converge at “Successful AI Integration.”]
The Importance of Realistic Expectations
The hype surrounding AI’s potential to revolutionize productivity is undeniable. However, unrealistic expectations can lead to significant disappointment and hinder the successful integration of AI into workflows. Managing expectations effectively is crucial for a smooth and beneficial AI adoption process. Understanding the gradual nature of AI-driven improvements and communicating this clearly to stakeholders is paramount.Overpromising AI’s capabilities can lead to several pitfalls.
Companies might invest heavily in AI solutions expecting immediate and dramatic returns, only to be met with underwhelming results. This can lead to disillusionment, budget cuts, and ultimately, the abandonment of promising AI initiatives. Furthermore, unrealistic expectations can create internal friction, with employees becoming frustrated by the perceived lack of immediate productivity gains and potentially resisting the adoption of new technologies.
The pressure to demonstrate quick wins can also incentivize the deployment of AI solutions before they are fully mature or appropriately integrated, leading to suboptimal performance and wasted resources.
Managing Expectations Around AI Adoption
Effective communication strategies are key to setting realistic expectations. Transparency is crucial. Stakeholders need to understand that AI is a tool that requires careful integration, training, and adaptation, not a magic bullet. Clear communication should Artikel the potential benefits of AI, but also emphasize the time and effort required for successful implementation. This includes detailing the necessary training for employees, the potential for initial inefficiencies during the transition, and the iterative nature of AI improvement.
For example, a company introducing an AI-powered customer service chatbot might communicate that initial customer satisfaction might even dip slightly as the system learns and adapts, before steadily improving over time. They could also highlight metrics like reduced agent workload or faster response times as key indicators of success, rather than focusing solely on immediate increases in overall productivity.
Visualizing Incremental AI Productivity Gains
Imagine a graph. The X-axis represents time, and the Y-axis represents productivity. The line starts at a baseline representing current productivity levels. Instead of a sharp, vertical increase representing immediate gains, the line shows a gradual, upward slope. The slope is not consistently steep; there are periods of slower progress, plateaus, and even slight dips, representing the challenges of implementation and adaptation.
However, the overall trend is clearly upward, demonstrating the cumulative and incremental nature of AI-driven productivity improvements over time. This visual representation effectively communicates that AI’s benefits are not instantaneous but rather unfold progressively as the technology matures and integrates seamlessly into the workflow. The graph also subtly acknowledges the challenges and setbacks that are part of the process, preventing unrealistic expectations.
So, while the promise of AI-driven productivity is undeniably exciting, let’s temper our enthusiasm with a dose of realism. The path to reaping the benefits of AI isn’t a sprint; it’s a marathon. It demands strategic planning, substantial investment, and a clear understanding of the limitations, as well as the incredible potential, of this transformative technology. By focusing on realistic expectations and addressing the challenges head-on, businesses can pave the way for significant long-term productivity gains, avoiding the disappointment of expecting immediate results.