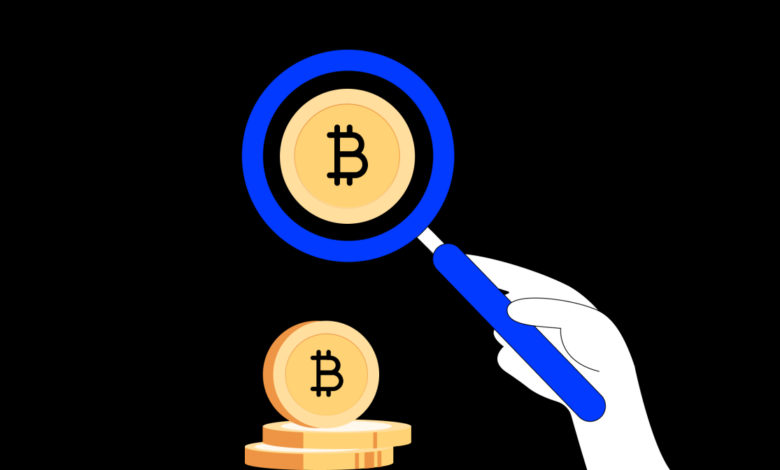
Crypto Trading Success Understanding Sentiment Analysis
Understanding Market Sentiment Analysis in Cryptocurrency Trading Success is key to navigating the volatile crypto market. This exploration delves into how analyzing public sentiment, from social media buzz to news articles, can provide valuable insights into potential price movements. We’ll uncover various methods, tools, and techniques to effectively interpret this data and potentially enhance your trading strategies.
From defining market sentiment and exploring different analysis approaches to integrating sentiment into your trading strategies, we’ll cover the entire spectrum of this fascinating field. This comprehensive guide will equip you with the knowledge to make more informed decisions in the ever-evolving world of cryptocurrency.
Defining Market Sentiment
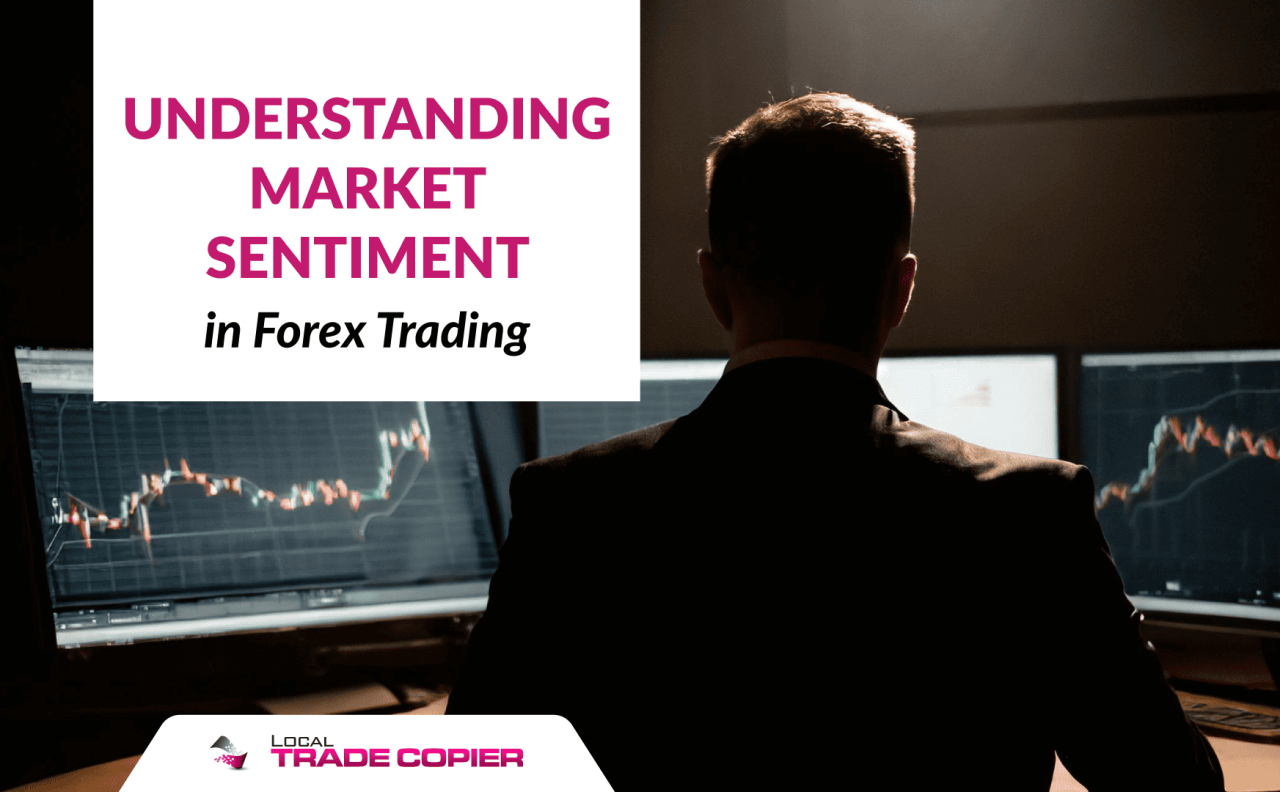
Source: mt4copier.com
Market sentiment in cryptocurrency trading reflects the collective emotional tone and overall outlook of investors toward a specific cryptocurrency or the market as a whole. It’s a crucial factor in price movements, often acting as a leading indicator of potential future price action. Understanding and interpreting market sentiment can help traders make more informed decisions and potentially capitalize on market trends.Understanding market sentiment involves more than just looking at price charts; it delves into the underlying feelings and opinions of market participants.
This sentiment can be positive, negative, or neutral, each carrying different implications for price action.
Defining Sentiment Types
Market sentiment can be categorized into three primary types: positive, negative, and neutral. Positive sentiment suggests optimism and enthusiasm, potentially driving price increases. Negative sentiment indicates pessimism and concern, which often leads to price declines. Neutral sentiment suggests a lack of strong opinions, and price movements are often less dramatic. In the cryptocurrency market, these sentiment types can be heavily influenced by news, social media trends, and on-chain data.
Factors Influencing Crypto Market Sentiment
Several factors contribute to the fluctuating sentiment within the cryptocurrency market. News events, such as regulatory announcements or major technological advancements, are key drivers. Social media buzz, particularly on platforms like Twitter and Reddit, often reflects collective investor sentiment in real-time. Furthermore, on-chain data, like transaction volume and address activity, can offer insights into market participation and investor behavior.
Sentiment Indicators Comparison
Sentiment Indicator | Description | Strengths | Weaknesses |
---|---|---|---|
Social Media Buzz | Tracking mentions, hashtags, and comments related to a specific cryptocurrency on platforms like Twitter, Reddit, and Discord. | Provides real-time insights into public perception, often reflecting immediate reactions to news or events. | Can be easily manipulated through coordinated campaigns or bots, and may not always accurately reflect the sentiment of the wider market. Requires careful analysis to filter out noise and identify genuine trends. |
News Articles | Analyzing articles and news reports about cryptocurrencies to determine the overall tone and sentiment. | Offers a broader perspective on the market, providing context and background information. | News can be biased, or late-breaking information may not be fully reflected in the analysis. Requires an understanding of the different perspectives represented in the media. |
On-Chain Data | Examining data related to cryptocurrency transactions, including transaction volume, address activity, and network metrics. | Provides objective insights into market participation and investor behavior. | Can be complex to interpret and requires technical expertise to derive meaningful conclusions from the data. Often requires specialized tools for analysis. |
Examples of Positive Sentiment Driving Price
Positive sentiment can significantly impact cryptocurrency prices. For example, a positive regulatory announcement, like a favorable ruling from a government agency, can lead to a surge in investor confidence and a subsequent price increase. Similarly, successful product launches or major technological breakthroughs can spark enthusiasm, generating positive sentiment and potentially driving significant price gains. In the past, announcements of successful partnerships or listings on major exchanges have also been instrumental in driving price appreciation, indicating a strong belief in the project’s future.
Understanding market sentiment is key to cryptocurrency trading success, but sometimes it’s hard to stay focused. Think about how moringa leaves, a superfood packed with nutrients, can boost your overall health, as detailed in this great article Why Moringa Leaves Are a Superfood for a Healthier Lifestyle. Similarly, understanding investor sentiment and market trends is vital for making informed decisions in the crypto market.
By staying grounded and informed, you’ll be better equipped to navigate the unpredictable cryptocurrency landscape.
These are examples of how positive sentiment can translate into real price increases.
Methods of Sentiment Analysis
Unveiling the pulse of the cryptocurrency market requires understanding how investors feel about the assets. Sentiment analysis, a powerful tool, delves into text data to gauge the prevailing mood, from bullish enthusiasm to bearish apprehension. This helps predict market movements and inform trading strategies. By analyzing public opinions expressed in social media, news articles, and forums, traders can gain valuable insights into market sentiment, potentially identifying trends before they manifest in price fluctuations.Sentiment analysis in crypto markets is not a crystal ball, but a valuable compass.
It provides probabilities and tendencies rather than definitive predictions. By understanding the methods and limitations, traders can effectively incorporate this tool into their strategies. Critically evaluating the sources and methodologies is essential for making informed decisions.
Natural Language Processing (NLP) in Sentiment Extraction
Natural language processing (NLP) forms the backbone of sentiment analysis. NLP techniques are crucial for extracting sentiment from unstructured text data. This involves breaking down the text into individual words or phrases (tokens), understanding the context within which these words appear, and then assigning a sentiment score (positive, negative, or neutral) to each piece of text. Sophisticated NLP models are trained on massive datasets of text and associated sentiments to identify patterns and nuances in language.
For example, a model might learn that words like “bullish,” “positive,” and “growth” often indicate positive sentiment, while words like “bearish,” “negative,” and “crash” signify negative sentiment.
Machine Learning Algorithms for Sentiment Analysis
Machine learning algorithms are instrumental in automating and refining the sentiment analysis process. These algorithms learn from labeled datasets of text and sentiment, allowing them to classify new text data with increasing accuracy. Common machine learning algorithms used in sentiment analysis include Support Vector Machines (SVMs), Naive Bayes classifiers, and Recurrent Neural Networks (RNNs). SVMs excel at finding optimal decision boundaries in sentiment classification, while Naive Bayes classifiers offer simplicity and speed.
RNNs, particularly LSTMs and GRUs, excel at handling sequential data like text, enabling more nuanced sentiment analysis.
Figuring out market sentiment is key to crypto trading success. But a balanced approach, like the one discussed in Creating a Balanced Investment Strategy Between Traditional Markets and Crypto , is crucial too. Understanding how investor emotions affect prices in both traditional and crypto markets is essential for informed decisions. Ultimately, mastering market sentiment analysis remains vital for profitable crypto trading.
Data Sources for Sentiment Analysis
Several data sources are available for extracting sentiment in cryptocurrency markets. These include:
- Social Media Platforms (Twitter, Reddit): Social media posts provide real-time insights into public opinion. These platforms offer a wealth of information regarding trader sentiment. Twitter’s hashtags and trending topics, along with Reddit’s subreddit discussions, are often indicative of current market sentiment.
- News Articles and Financial Websites: News articles and financial websites offer a broader perspective on market sentiment, encompassing expert opinions and market analysis. This provides context and depth to the sentiment extracted from social media data. Reliable news outlets, financial journals, and market analysis sites contribute to the overall picture of market sentiment.
- Cryptocurrency Forums and Discussion Boards: These platforms provide a direct channel for community sentiment, offering insights from active traders and investors. The insights from these specialized platforms can provide specific nuances and perspectives that other data sources may miss.
Sentiment Analysis Tools Comparison
Different sentiment analysis tools offer varying levels of accuracy and functionality. A comparison table below illustrates the pros and cons of some popular tools.
Tool | Pros | Cons |
---|---|---|
Tool A | High accuracy, advanced NLP models | Expensive, requires significant computational resources |
Tool B | User-friendly interface, affordable | Lower accuracy compared to more advanced tools |
Tool C | Real-time data analysis, readily available API | Limited customization options, potentially biased results |
Sentiment Analysis Tools and Techniques
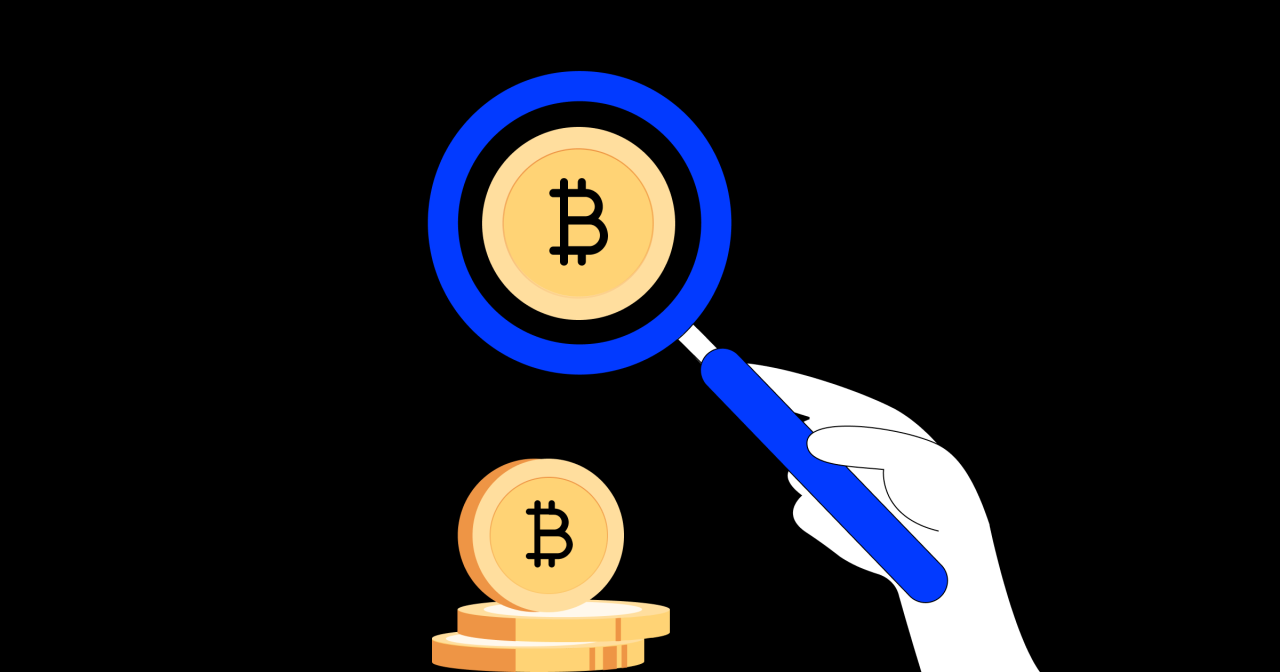
Source: mudrex.com
Unveiling the intricate world of market sentiment requires sophisticated tools and techniques to accurately gauge public opinion. Sentiment analysis, when properly implemented, can provide valuable insights into the prevailing market mood, helping traders make more informed decisions. Different tools and methods offer varying degrees of accuracy and complexity, each with its own strengths and limitations. Understanding these nuances is crucial for navigating the complexities of cryptocurrency trading.Sentiment analysis tools range from simple -based approaches to complex machine learning models.
The choice of tool depends heavily on the specific needs of the analysis, the volume of data being processed, and the desired level of sophistication. These tools can range from readily available APIs to custom-built solutions, allowing for varying degrees of customization.
Sentiment Analysis Tools
Various tools and technologies are available for performing sentiment analysis. These tools range from basic -based tools to advanced machine learning algorithms. The selection of a particular tool depends on the desired level of sophistication, the volume of data to be processed, and the required accuracy.
- Natural Language Processing (NLP) Libraries: Libraries like NLTK (Natural Language Toolkit) and spaCy offer pre-built functionalities for tokenization, stemming, and lemmatization. These libraries can be integrated into custom-built sentiment analysis pipelines, allowing for a high degree of customization. However, the setup and maintenance of such pipelines can be complex, requiring significant expertise.
- Cloud-Based Sentiment Analysis APIs: Cloud providers like AWS, Google Cloud, and Azure offer APIs specifically designed for sentiment analysis. These APIs often leverage pre-trained machine learning models, providing readily accessible and accurate sentiment analysis capabilities. Their strength lies in ease of use and scalability, but the level of customization might be limited.
- Third-Party Sentiment Analysis Platforms: Specialized platforms provide dedicated sentiment analysis tools, often with user-friendly interfaces and pre-configured models. These platforms typically focus on specific domains or markets, and may offer tailored features for particular use cases, such as cryptocurrency trading. The accuracy and reliability of these platforms depend heavily on the quality and training data of the underlying models.
Accuracy and Reliability of Sentiment Analysis Techniques
The accuracy of sentiment analysis hinges on several factors. The quality and size of the training data significantly impact the model’s performance. Also, the choice of algorithms and the specific language used in the analyzed text plays a crucial role. Techniques like lexicon-based methods, while simpler, often lack the nuanced understanding of context that more advanced methods possess.
- Lexicon-Based Methods: These methods rely on predefined dictionaries or lists of words associated with positive, negative, and neutral sentiments. While simple to implement, they can struggle with nuanced language, sarcasm, or context-dependent meanings.
- Machine Learning Methods: Machine learning algorithms, such as Support Vector Machines (SVM) and Recurrent Neural Networks (RNNs), are more sophisticated and can better understand context. These methods generally produce more accurate results but require significant computational resources and labeled training data.
Evaluating Sentiment Analysis Model Accuracy
Evaluating the accuracy of a sentiment analysis model is a crucial step in ensuring its reliability. A variety of metrics are used, including precision, recall, F1-score, and accuracy. These metrics measure the model’s ability to correctly classify positive, negative, and neutral sentiments.
A model with high precision correctly identifies many positive sentiments, but might miss some. A model with high recall identifies many positive sentiments, but might also incorrectly label some negative sentiments as positive.
A comprehensive evaluation should consider various aspects, including the dataset’s characteristics and the model’s performance on different sentiment categories. A well-structured evaluation protocol, using techniques like cross-validation, helps minimize bias and provide a more robust assessment of the model’s performance.
Sentiment Analysis Platform Capabilities, Understanding Market Sentiment Analysis in Cryptocurrency Trading Success
Platform | Capabilities | Strengths | Limitations |
---|---|---|---|
AWS Comprehend | Sentiment analysis for various languages, including English. | Scalability, wide language support, integration with other AWS services. | Might not be ideal for highly specialized use cases or very specific market analysis. |
Google Cloud Natural Language | Offers a range of NLP tasks, including sentiment analysis, across various languages. | Robust API, integration with other Google Cloud services. | Might require significant setup for custom model training. |
NLTK | Open-source library allowing custom model creation. | Flexibility and control over model building. | Requires programming expertise and resources for development. |
Integrating Sentiment Analysis into Trading Strategies: Understanding Market Sentiment Analysis In Cryptocurrency Trading Success
Sentiment analysis, when effectively integrated into a cryptocurrency trading strategy, can provide valuable insights into market dynamics beyond price charts. It allows traders to identify potential turning points and adjust their positions proactively, potentially improving returns. This approach complements traditional technical analysis by considering the collective emotional response of market participants.By understanding the prevailing sentiment, traders can anticipate shifts in market behavior and make informed decisions.
This approach aims to identify trends before they fully manifest in price movements, giving traders a potential edge in the market.
Examples of Incorporating Sentiment Analysis into a Trading Strategy
Sentiment analysis can be integrated into various stages of a trading strategy. For instance, a trader might use social media sentiment to gauge the overall mood surrounding a particular cryptocurrency. Positive sentiment could suggest a potential upward trend, while negative sentiment might signal a downward movement. Furthermore, news sentiment analysis can be used to determine if recent news articles are bullish or bearish, and how this impacts market sentiment.
Identifying Potential Market Trends Using Sentiment Data
Sentiment analysis can be a powerful tool for identifying potential market trends. A significant shift from positive to negative sentiment on a specific cryptocurrency, coupled with declining trading volume, could signal an impending price drop. Conversely, a surge in positive sentiment and increasing trading volume might suggest a forthcoming price increase. Careful monitoring of these indicators can help traders anticipate market movements.
Adjusting Trading Positions Based on Sentiment Data
Sentiment analysis data can be used to adjust trading positions dynamically. For example, if a trader notices a strong bearish sentiment emerging on a particular cryptocurrency, they might choose to reduce their holdings or even take a short position. Conversely, a significant uptick in positive sentiment could trigger an increase in their holdings or a long position. By using sentiment data, traders can adapt their strategies in real-time to market conditions.
Correlation of Sentiment Levels and Price Movements
Sentiment Level | Description | Likely Price Movement | Trading Strategy Recommendation |
---|---|---|---|
Extremely Bullish | High levels of positive sentiment, significant increase in trading volume. | Potential for price increase. | Increase long positions, consider leveraged trading (with caution). |
Bullish | Positive sentiment, steady trading volume. | Continued upward trend or consolidation. | Maintain or increase long positions, monitor for potential pullbacks. |
Neutral | Balanced sentiment, moderate trading volume. | Possible consolidation or sideways movement. | Hold current positions, wait for clear signals. |
Bearish | Negative sentiment, decreasing trading volume. | Potential for price decrease. | Reduce or close long positions, consider short positions. |
Extremely Bearish | High levels of negative sentiment, significant decrease in trading volume. | Potential for substantial price drop. | Close all positions, avoid further trading until sentiment reverses. |
This table provides a general guideline; the actual correlation between sentiment and price movement can vary significantly depending on specific circumstances.
Backtesting Trading Strategies Using Sentiment Data
Backtesting trading strategies incorporating sentiment analysis involves applying the strategy to historical data, including sentiment data. This process allows traders to evaluate the effectiveness of the strategy in different market conditions. Tools for sentiment analysis can be used to tag historical news or social media posts, creating a dataset for testing purposes. Results from the backtest can then be analyzed to determine the strategy’s profitability and risk characteristics.
Significant factors to consider include the time frame of the backtest and the accuracy of the sentiment data used. A well-designed backtest can help traders refine their strategies and make informed decisions about implementing them in live trading.
Challenges and Limitations of Sentiment Analysis
Sentiment analysis, while a powerful tool, faces significant hurdles when applied to volatile cryptocurrency markets. The inherent unpredictability and rapid fluctuations of these markets make accurate sentiment measurement challenging. Furthermore, the complex interplay of various factors, including news, social media chatter, and technical indicators, often obfuscates the true sentiment behind market movements.Understanding these limitations is crucial for effectively integrating sentiment analysis into trading strategies.
Simply relying on sentiment alone can lead to flawed decisions and significant losses. A balanced approach that considers sentiment alongside other market indicators is vital for informed trading.
Challenges in Accurately Measuring Sentiment
Cryptocurrency markets are notoriously difficult to analyze due to their decentralized nature and the ever-evolving landscape of social media and news. The sheer volume of data, often contradictory and rapidly changing, poses a significant obstacle for sentiment analysis tools. Moreover, the nuanced language used in social media posts and news articles, replete with slang, jargon, and sarcasm, can easily be misinterpreted, leading to inaccurate sentiment classifications.
Limitations of Sentiment Analysis for Predicting Market Outcomes
Sentiment analysis, while helpful in identifying prevailing market sentiment, is not a reliable predictor of future price movements. Past sentiment data, while potentially informative, does not guarantee future price trends. The dynamic nature of the cryptocurrency market, driven by unforeseen events and speculative bubbles, renders predictions based solely on sentiment analysis inherently unreliable. For instance, a sudden surge in positive sentiment may not always translate to a price increase, and conversely, negative sentiment might not necessarily lead to a downturn.
Potential for Bias and Errors in Sentiment Analysis
Sentiment analysis tools are susceptible to biases inherent in the data they process. These biases can stem from the specific algorithms used, the source of the data, or the inherent subjectivity of language itself. For example, a tool trained primarily on English-language data might struggle to accurately interpret sentiment expressed in other languages, leading to misinterpretations. Furthermore, the subjective nature of sentiment makes it difficult to achieve perfect objectivity in analysis.
Different people may interpret the same message with varying degrees of intensity and bias, affecting the accuracy of sentiment analysis.
Examples of Misleading Sentiment Analysis
A significant surge in positive sentiment about a particular cryptocurrency might be triggered by a coordinated pump-and-dump scheme, where artificial demand is artificially created to manipulate the price. Sentiment analysis, focusing solely on the positive feedback, might fail to identify the underlying manipulation, leading to erroneous conclusions. Similarly, a sudden influx of negative news or social media chatter about a cryptocurrency, even if legitimate, may be amplified and misrepresented by automated sentiment analysis tools, leading to an overreaction and a market downturn.
Summary of Potential Sources of Error in Cryptocurrency Sentiment Analysis
Source of Error | Description | Impact |
---|---|---|
Data Bias | Sentiment analysis tools trained on skewed data sets might produce inaccurate results. | Misleading interpretations of prevailing sentiment. |
Language Nuance | Specialized jargon, sarcasm, and slang in social media posts can be misinterpreted. | Inaccurate sentiment classification. |
Data Volume and Velocity | The massive and rapid influx of data can overwhelm analysis tools. | Overlooked signals, delayed responses, and reduced accuracy. |
Market Manipulation | Coordinated efforts to artificially inflate or deflate sentiment can mislead analysis. | False signals, unreliable predictions. |
Algorithmic Limitations | Inherent limitations of algorithms used in sentiment analysis can lead to biases. | Inaccurate conclusions, flawed analysis. |
Real-World Case Studies
Sentiment analysis, when applied correctly, can offer valuable insights into market trends, providing a potential edge in cryptocurrency trading. However, its effectiveness depends heavily on the quality of the data, the sophistication of the analysis, and the accuracy of interpretation within a specific context. Understanding how sentiment has influenced past price movements can help traders refine their strategies and mitigate risks.Analyzing past instances where sentiment analysis played a role in cryptocurrency price fluctuations can offer valuable insights for future trading decisions.
By examining historical patterns, traders can develop more informed strategies that incorporate emotional market factors. This understanding can lead to a more nuanced approach to market prediction and decision-making.
Specific Instances of Sentiment Impact on Cryptocurrency Prices
Sentiment analysis can be a crucial tool in understanding market behavior. News articles, social media posts, and forum discussions often reflect prevailing sentiment, which can influence price action. For example, a surge of positive sentiment surrounding a particular cryptocurrency might lead to increased buying pressure, driving up the price. Conversely, negative sentiment can trigger selling pressure and cause prices to fall.
Results of Sentiment Analysis in Predicting Price Changes
The accuracy of sentiment analysis in predicting price changes varies greatly depending on factors like the specific cryptocurrency, the volume and nature of sentiment data used, and the quality of the analytical model. While sentiment analysis alone is not a guaranteed predictor of price movements, it can be a valuable complementary tool to other market indicators.
Knowing how people feel about cryptocurrencies is key to trading success. Understanding market sentiment analysis is crucial, but it’s not the whole picture. You also need to consider the underlying market psychology, like fear and greed, to make better investment choices. This means recognizing how investor emotions influence prices, which is covered in detail in this helpful guide on Understanding Market Psychology to Make Better Cryptocurrency Investment Choices.
Ultimately, successful crypto trading combines a keen eye for sentiment analysis with a grasp of market psychology for informed decisions.
Successful Trading Strategies Leveraging Sentiment Data
Successful trading strategies often integrate sentiment analysis with technical indicators and fundamental analysis. A trader might combine sentiment data with volume analysis to assess the strength of a price movement. For example, if positive sentiment coincides with high trading volume, it could signal a potentially strong uptrend. Conversely, low volume with negative sentiment could indicate a weakening market trend.
Strategies need to consider the overall market context and not rely solely on sentiment data.
Comparison of Different Case Studies
Case Study | Cryptocurrency | Sentiment Source | Analysis Method | Predicted Price Change | Actual Price Change | Success/Failure | Contextual Factors |
---|---|---|---|---|---|---|---|
Bitcoin 2021 Bull Run | Bitcoin | News articles, social media | Natural Language Processing (NLP) on news sentiment | Upward trend | Upward trend | Success | Strong institutional adoption, positive regulatory outlook |
Ethereum’s Merge | Ethereum | Crypto forums, technical analysis sites | Sentiment analysis of community discussion | Slight price correction | Significant price correction | Failure | Uncertainty surrounding the Merge, technical issues |
Dogecoin’s Meme-Driven Rally | Dogecoin | Social media posts, Reddit threads | Sentiment analysis of social media comments | Upward trend | Upward trend (followed by a crash) | Limited Success | High speculative trading, lack of underlying value |
Importance of Context in Interpreting Sentiment Data
It is crucial to consider the context surrounding the sentiment data when interpreting its implications for price movements. For instance, positive sentiment about a cryptocurrency’s development might be irrelevant if the broader market is experiencing a downturn. Similarly, negative sentiment about a project might be overblown if there are strong fundamental reasons supporting its long-term value. Contextual awareness is essential to avoid drawing inaccurate conclusions based solely on sentiment.
Future Trends and Developments
The cryptocurrency market is dynamic, and sentiment analysis, while valuable today, is poised for significant evolution. As the market matures and trading volumes increase, the need for more sophisticated and accurate sentiment analysis tools becomes paramount. This evolution is driven by advancements in AI, the sheer volume of data generated, and the need for more nuanced interpretations of market chatter.
Emerging Trends in Sentiment Analysis
Sentiment analysis for crypto markets is moving beyond basic positive/negative classifications. Sophisticated algorithms are being developed to identify nuanced sentiment, such as excitement, fear, or uncertainty. This is crucial because these subtle distinctions can predict market movements more accurately than simple polarity. For instance, a surge in “excited” tweets about a new cryptocurrency launch could signal an upcoming price surge, while a large number of “fear” posts about a market correction could be a precursor to a significant sell-off.
Advanced AI Techniques
Incorporating more advanced AI techniques, like natural language processing (NLP) and machine learning (ML), is a key trend. NLP enables computers to understand and interpret human language with greater precision. ML algorithms can learn from vast datasets of market sentiment and historical price movements, allowing for the development of more accurate prediction models. This approach can identify patterns and correlations in sentiment that might be missed by traditional methods.
For example, a machine learning model trained on Twitter data, news articles, and forum discussions can identify specific phrases or s associated with price fluctuations.
Evolution of Sentiment Analysis Tools and Technologies
Sentiment analysis tools are becoming more sophisticated, capable of handling more complex data sources and incorporating real-time analysis. Integration with social media feeds, news aggregators, and cryptocurrency forums allows for comprehensive and dynamic sentiment capture. Furthermore, the ability to analyze sentiment across multiple languages is crucial for global markets. This is exemplified by the increasing use of multilingual NLP models that can interpret sentiment expressed in different languages, enabling a broader view of global market sentiment.
Future of Sentiment Analysis
The future of sentiment analysis in crypto trading promises to be one of greater precision and real-time insights. By incorporating advanced AI techniques and expanding data sources, analysts will be able to predict market movements with higher accuracy. This will translate to better trading strategies and risk management, ultimately benefiting both investors and traders. Tools will likely become more user-friendly, allowing for easier integration into existing trading platforms.
Predicted Advancements
Category | Predicted Advancement | Impact |
---|---|---|
Data Sources | Inclusion of blockchain data, on-chain metrics, and decentralized social media platforms. | Enhanced accuracy by considering a wider range of data points. |
Analysis Techniques | Implementation of deep learning models for more complex sentiment extraction and predictive modeling. | Improved accuracy and the ability to identify nuanced sentiment patterns. |
Real-time Analysis | Development of real-time sentiment analysis dashboards and alerts. | Enables quicker response to market shifts and potential trading opportunities. |
Accessibility | User-friendly interfaces and integration into existing trading platforms. | Increased adoption and wider use of sentiment analysis in the crypto community. |
Last Point
In conclusion, understanding market sentiment analysis is crucial for success in cryptocurrency trading. While not a guaranteed predictor of future price action, it offers valuable context and potential insights. By carefully evaluating different sentiment indicators, employing robust analysis techniques, and integrating the insights into well-defined trading strategies, you can potentially gain a competitive edge. Remember, however, that sentiment analysis is just one piece of the puzzle; a balanced approach combining various market indicators is always recommended.
Questions Often Asked
What are some common data sources for sentiment analysis in crypto?
Common data sources include social media platforms like Twitter and Reddit, news articles from various financial publications, and on-chain data reflecting transactions and activity within the blockchain.
How can I evaluate the accuracy of a sentiment analysis model?
Accuracy evaluation involves comparing the model’s predictions against known sentiment labels or price movements. Metrics like precision, recall, and F1-score can help assess its reliability.
What are the limitations of using sentiment analysis for predicting market outcomes?
Sentiment analysis can be influenced by noise, biases, and a lack of context. It’s crucial to remember that sentiment is just one piece of the puzzle, and other factors like market fundamentals, technical indicators, and regulatory changes also significantly impact cryptocurrency prices.
How can I avoid potential biases in sentiment analysis?
To mitigate bias, you should carefully consider the source of the data, the methodology of sentiment extraction, and the potential for human interpretation errors. Using diverse data sources and employing multiple sentiment analysis techniques can help to reduce bias.