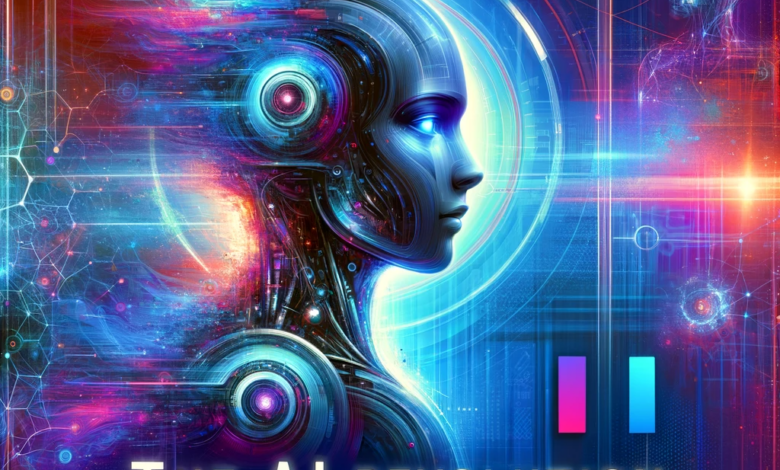
What Happened to the AI Revolution?
What happened to the artificial intelligence revolution? We were promised flying cars and robot butlers, weren’t we? The reality, however, has been a bit… less spectacular. This post dives into the hype, the hurdles, and the surprisingly human side of AI’s journey so far, exploring why the initial projections haven’t quite materialized and where we might be headed next.
From the initial explosion of excitement surrounding self-driving cars and AI-powered medical diagnoses to the current, more nuanced understanding of AI’s capabilities and limitations, the path has been filled with both remarkable progress and unexpected setbacks. We’ll examine the technological roadblocks, the ethical dilemmas, and the shifting priorities in AI research that have shaped the narrative.
Hype vs. Reality
The AI revolution, as depicted in popular culture and initially touted by tech giants, promised a future brimming with self-driving cars, personalized medicine powered by AI diagnostics, and robots handling all mundane tasks. This vision painted a picture of unprecedented efficiency, convenience, and societal advancement, fueled by rapid breakthroughs in machine learning and artificial intelligence. However, the reality has been far more nuanced, revealing a significant gap between the hype and the actual progress made.
Areas Where AI Fell Short of Expectations
Many predicted a swift and seamless integration of AI into all aspects of life. While AI has made significant strides in specific domains, its widespread transformative impact has been slower than initially anticipated. For example, fully autonomous vehicles, despite substantial investment, remain largely confined to controlled environments and haven’t achieved the level of safety and reliability necessary for widespread public adoption.
Similarly, while AI is increasingly used in healthcare, it’s often employed as a supplementary tool rather than a complete replacement for human expertise in diagnosis and treatment. The expectation of AI handling all mundane tasks has also been largely unmet; while AI automates certain processes, it has yet to achieve the level of general intelligence needed to tackle the diverse and unpredictable nature of many human tasks.
Comparison of Projected and Actual AI Advancements
Initial projections often suggested a much faster pace of AI development. Some experts predicted widespread adoption of sophisticated AI systems within a decade, whereas the reality shows a more gradual and incremental progress. The challenge lies in the complexity of building truly intelligent systems capable of reasoning, adapting, and learning in the same way humans do. The development of general-purpose AI, capable of handling a wide range of tasks, remains a distant goal, far beyond the initial projected timelines.
For instance, the development of truly sentient AI, a staple of science fiction, is still largely theoretical and faces numerous unsolved technical and ethical challenges.
The Role of Media Hype in Shaping Public Perception
The media played a significant role in amplifying the initial excitement surrounding AI. Sensationalized headlines and futuristic portrayals in movies and television shows created an inflated perception of AI’s capabilities. This hype cycle, while beneficial in attracting investment and talent to the field, also created unrealistic expectations among the public, leading to disappointment when the promised advancements failed to materialize on the projected timeline.
The portrayal of AI as either a utopian savior or a dystopian threat often overshadowed the more nuanced and complex reality of AI development and its limitations.
Promised AI Applications vs. Current Reality
The following table illustrates the discrepancy between the promised capabilities of various AI applications and their current state:
Application | Promised Capability | Current Reality | Discrepancy |
---|---|---|---|
Self-Driving Cars | Fully autonomous driving in all conditions | Limited autonomous features in specific environments; human oversight still required | Significant; full autonomy remains a challenge |
AI-powered Medical Diagnosis | Completely automated and accurate diagnosis of diseases | Assists human doctors; improves accuracy but doesn’t replace human expertise | Moderate; AI serves as a valuable tool but not a complete replacement |
Personalized Education | AI tutors adapting to individual learning styles and needs | Personalized learning platforms exist but are limited in scope and adaptive capabilities | Moderate; progress is being made but widespread adoption with full personalization is still some time away |
Robot Domestic Assistants | Robots performing all household chores autonomously | Limited automation of specific tasks; robots struggle with complex, unpredictable tasks | Significant; general-purpose household robots are far from reality |
Technological Hurdles and Limitations: What Happened To The Artificial Intelligence Revolution
The hype surrounding the AI revolution often overshadows the significant technological hurdles that continue to impede its progress. While impressive advancements have been made, particularly in narrow AI applications, the path to truly intelligent, general-purpose AI remains fraught with challenges. These challenges stem from both the inherent limitations of current algorithms and the sheer computational demands of creating truly sophisticated systems.The development of truly advanced AI faces a complex interplay of factors, ranging from the limitations of current machine learning algorithms to the sheer scale of computational resources required.
This section will delve into these key technological bottlenecks.
Computational Challenges
Creating powerful AI systems requires immense computational resources. Training large language models, for instance, can consume massive amounts of energy and time, often requiring clusters of high-performance GPUs operating for weeks or even months. This presents a significant barrier to entry, limiting the research and development capabilities of many institutions and researchers. The sheer scale of data required to train these models also poses a problem, necessitating vast data storage and processing capabilities that are not readily available to everyone.
For example, training a large language model like GPT-3 required an estimated 175 billion parameters and a significant investment in computing power, making it inaccessible to most researchers. This imbalance in access to computational resources creates a disparity in the field, potentially hindering innovation.
Limitations of Current Machine Learning Algorithms
Current machine learning algorithms, while powerful, are fundamentally limited. Many rely on supervised learning, requiring massive labeled datasets which are expensive and time-consuming to create. Furthermore, these algorithms often struggle with generalization – performing well on the data they were trained on but failing to adapt to new, unseen data. This lack of robustness is a major obstacle in creating truly reliable and versatile AI systems.
For example, a facial recognition system trained on images of people with lighter skin tones may perform poorly when presented with images of people with darker skin tones, highlighting the limitations of generalization in current algorithms. Similarly, current algorithms often struggle with causal reasoning and common sense, areas where human intelligence excels.
Difficulties in Achieving General-Purpose AI
A major goal of AI research is to create general-purpose AI (AGI) – systems capable of performing any intellectual task that a human being can. However, achieving AGI remains a significant challenge. Current AI systems excel at specific tasks, but they lack the flexibility, adaptability, and common sense reasoning abilities of humans. Building systems that can learn and adapt in diverse, unpredictable environments, and exhibit human-level intelligence across a wide range of domains, is a far more complex undertaking than current techniques allow.
The development of AGI requires significant breakthroughs in areas such as knowledge representation, reasoning, and learning.
Specific Technological Bottlenecks, What happened to the artificial intelligence revolution
Several specific technological bottlenecks have slowed progress in AI development. These include the limitations of current hardware, the difficulty in designing algorithms that can handle noisy or incomplete data, and the challenges of creating truly robust and explainable AI systems. The lack of standardized benchmarks and evaluation metrics also hinders progress, making it difficult to compare the performance of different AI systems and track advancements objectively.
The development of more efficient and powerful hardware, such as neuromorphic chips inspired by the human brain, is crucial for overcoming computational limitations.
Unsolved Problems Limiting AI’s Advancement
The path to more advanced AI is blocked by several crucial unsolved problems.
- Robustness and Generalization: Creating AI systems that can reliably perform in unpredictable and unfamiliar environments.
- Explainability and Interpretability: Understanding how complex AI systems arrive at their decisions.
- Common Sense Reasoning: Equipping AI systems with the basic understanding of the world that humans possess.
- Causal Inference: Enabling AI systems to understand cause-and-effect relationships.
- Data Efficiency: Developing algorithms that can learn effectively from limited data.
- Bias and Fairness: Mitigating biases in training data and ensuring fair and equitable outcomes.
- Security and Safety: Developing secure and reliable AI systems that cannot be easily manipulated or misused.
So, what’s the verdict on the AI revolution? It’s not dead, but it’s definitely not what many initially envisioned. The journey has been a fascinating blend of breakthroughs and limitations, highlighting the complex interplay between technology, ethics, and societal expectations. While the transformative potential of AI remains immense, the path forward requires careful consideration, responsible development, and a clear understanding of both its capabilities and its inherent risks.
The future of AI is still being written, and it’s a story worth following.
So, the AI revolution? It feels like we’re in a bit of a lull, right? Maybe the hype outpaced reality. But thinking about unexpected price hikes got me wondering about the interconnectedness of things – check out this article on why orange juice has never been more expensive – it shows how seemingly unrelated factors can impact our daily lives, just like the slow burn of AI integration might be affecting us in subtle, unexpected ways.
The point is, complex systems are hard to predict, whether it’s AI or the global orange juice market.
So, where’d the AI revolution go? It feels like we were promised flying cars, but instead got… slightly better spam filters. The hype cycle, as usual, overshot the reality. But geopolitical shifts are impacting tech development too; check out this article on how Taiwan is trying to learn from the wars in Gaza and Ukraine , which shows how real-world conflicts can dramatically alter technological priorities and funding.
This shift in focus is probably a major reason why the AI revolution seems to have stalled, at least for now.
So, the AI revolution everyone hyped? It seems to have plateaued a bit, right? We’re still seeing advancements, but the explosive growth predicted hasn’t quite materialized. This makes me think about fostering diverse fields of innovation, which is why a new art gallery in Bangalore is so important, as discussed in this insightful article: why a new art gallery in bangalore is important for indian science.
Supporting creativity alongside tech might be the key to unlocking the next phase of the AI revolution, a more holistic and human-centered one.