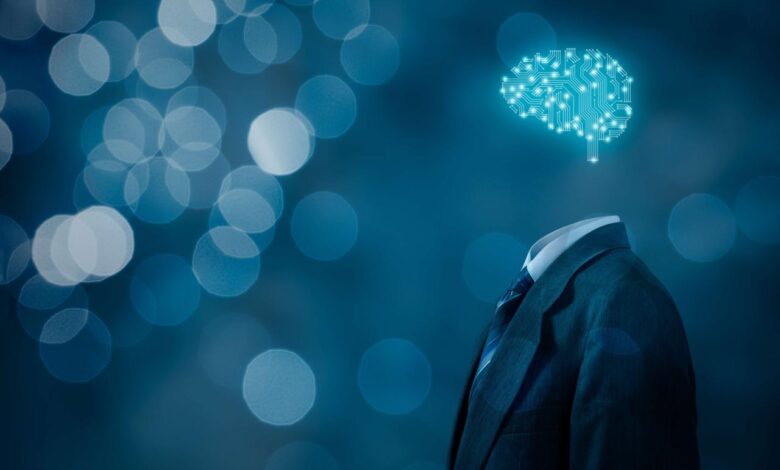
Why Companies Struggle to Onboard AI
Why companies are struggling to onboard AI is a complex issue, far beyond a simple lack of budget. It’s a multifaceted challenge encompassing technical hurdles, cultural resistance, and a surprising shortage of the right expertise. This isn’t just about buying software; it’s about fundamentally transforming how a business operates, and that transformation requires careful planning, significant investment, and a clear understanding of the potential pitfalls.
From the difficulties of integrating AI into existing systems to the ethical considerations surrounding its use, the path to successful AI implementation is paved with obstacles. This post delves into the key reasons why so many companies are finding AI adoption more challenging than anticipated, exploring the technical, financial, and human factors that play a crucial role.
Data-Related Issues: Why Companies Are Struggling To Onboard Ai
The successful implementation of AI heavily relies on the quality and suitability of the data used to train and operate the models. Poor data quality is a significant hurdle for many companies attempting AI adoption, leading to inaccurate predictions, biased outcomes, and ultimately, project failure. Addressing these data-related challenges is crucial for achieving meaningful results from AI initiatives.Data quality problems frequently derail AI projects.
These problems significantly impact the reliability and effectiveness of AI models. Overcoming these challenges requires a strategic approach that prioritizes data cleaning, preprocessing, and validation throughout the entire AI lifecycle.
Common Data Quality Problems, Why companies are struggling to onboard ai
Inconsistent data formats, missing values, and inaccurate entries are just a few of the common problems. Imagine trying to train a model to predict customer churn with inconsistent date formats – some using MM/DD/YYYY, others DD/MM/YYYY, and still others using YYYY-MM-DD. The model would struggle to interpret the data correctly, leading to unreliable predictions. Similarly, missing values for key variables like customer purchase history or engagement levels will significantly reduce the model’s accuracy.
Inaccurate entries, perhaps due to human error during data entry, further compound these issues. These inconsistencies and inaccuracies directly impact the model’s ability to learn and generalize effectively.
The Importance of Data Cleaning, Preprocessing, and Validation
Before any AI model can be trained, the data must undergo rigorous cleaning, preprocessing, and validation. Data cleaning involves identifying and correcting or removing inaccurate, incomplete, irrelevant, duplicated, or improperly formatted data. Preprocessing transforms the raw data into a format suitable for the AI model, which might include normalization, standardization, feature scaling, or feature engineering. Validation ensures the data accurately reflects the intended purpose and meets the quality standards required for the AI application.
Without these steps, the AI model is likely to produce unreliable and potentially harmful results. For instance, a model trained on improperly scaled data might produce wildly inaccurate predictions, rendering it useless for practical application.
Examples of Data Biases and Their Negative Impact
Data biases, often unintentional, can significantly impact AI performance and lead to unfair or discriminatory outcomes. For example, a facial recognition system trained primarily on images of light-skinned individuals may perform poorly when identifying individuals with darker skin tones, leading to misidentification and potential safety concerns. Similarly, a loan application AI trained on historical data reflecting existing societal biases might unfairly deny loans to applicants from certain demographic groups.
These biases, embedded within the data, are amplified by the AI model, perpetuating and even exacerbating existing inequalities. It’s crucial to identify and mitigate these biases through careful data selection, preprocessing techniques, and ongoing monitoring of the model’s performance across different demographic groups.
Designing a Data Governance Strategy
A robust data governance strategy is essential for addressing data quality issues. This strategy should define roles, responsibilities, and processes for data collection, storage, processing, and use. It should include clear guidelines for data quality standards, data validation procedures, and mechanisms for identifying and addressing data biases. Regular data audits, data quality monitoring, and feedback loops are crucial components of an effective data governance strategy.
For example, a company might establish a data quality team responsible for overseeing data collection processes, implementing data cleaning procedures, and regularly auditing data for accuracy and completeness. This proactive approach helps ensure that the data used to train and operate AI models is reliable, unbiased, and suitable for its intended purpose.
Successfully onboarding AI requires a holistic approach. It’s not enough to simply acquire the technology; companies must also invest in the necessary skills, address data quality issues, and cultivate a culture of acceptance and collaboration. Overcoming these challenges will unlock the transformative potential of AI, but only with a clear strategy, careful planning, and a commitment to long-term investment.
The journey may be complex, but the rewards for those who navigate it successfully are immense.
So many companies are struggling with AI integration, it’s crazy! The sheer complexity, coupled with a lack of skilled personnel, is a major hurdle. It reminds me of how unpredictable things can be – like finding a massive drug bust, as reported in this article about border patrol agents finding $43,000 worth of cocaine washed up on a Florida coastline.
You just never know what’s going to pop up, and similarly, unexpected issues constantly arise when implementing AI. Ultimately, successful AI onboarding requires careful planning and a dedicated team.
So many companies are struggling with AI adoption, it’s crazy! It’s not just about the tech itself, but also about geopolitical factors impacting the supply chain. For example, the news that trump does not want to do business with China’s Huawei highlights how international relations can severely disrupt access to crucial components and expertise needed for successful AI integration.
This kind of uncertainty makes long-term AI strategy planning a real headache for businesses.
Companies are struggling with AI onboarding because it’s not just about the tech; it’s about integrating it effectively into existing workflows and company culture. Think about it like this: successfully deploying AI requires a similar commitment and strategic planning as, say, a major military operation, which makes revisiting President Ronald Reagan’s Veterans Day remarks revisiting president ronald reagans veterans day remarks a surprisingly relevant exercise.
The lack of a clear, well-defined strategy, much like a poorly planned campaign, is a major reason why AI initiatives often fall short of expectations.