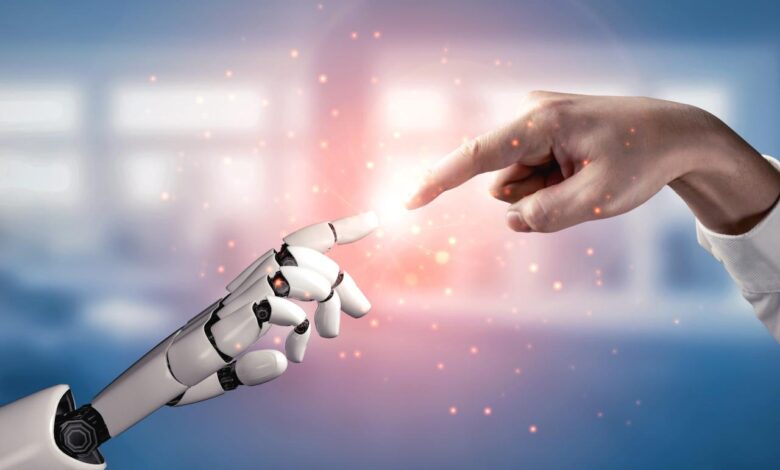
A New Lab and Paper Reignite Old AI Debate
A new lab and a new paper reignite an old AI debate, throwing the field into a fascinating whirlwind of renewed discussion. For years, the core questions about artificial intelligence’s potential – its capabilities, its limitations, and its ethical implications – have simmered beneath the surface. Now, fresh research is forcing us to confront these issues head-on, sparking vigorous debate among experts and enthusiasts alike.
This isn’t just about technical advancements; it’s about the very future of how we interact with technology and what that means for humanity.
This post dives into the heart of this resurgence, examining the historical context of the debate, the groundbreaking work of the new lab, and the compelling arguments laid out in the recent paper. We’ll explore the implications for various AI subfields, weigh the ethical considerations, and look towards potential future research directions. Get ready for a deep dive into one of the most crucial conversations in modern science!
The “Old AI Debate”: A New Lab And A New Paper Reignite An Old Ai Debate
The recent release of a new research paper and the unveiling of a cutting-edge AI lab have reignited a long-standing debate within the field of artificial intelligence: the question of whether machines can truly think, and if so, what that even means. This isn’t a new discussion; it’s a cyclical one, punctuated by periods of rapid technological advancement that force us to reconsider fundamental assumptions.
This time, however, the advancements are particularly compelling, prompting a fresh look at the arguments and counter-arguments that have shaped the field for decades.The core of the historical AI debate centers on the nature of intelligence itself and the possibility of replicating it artificially. Specifically, it grapples with the question of whether artificial intelligence can achieve genuine understanding, consciousness, and sentience, or whether it merely simulates these qualities through sophisticated algorithms.
This debate has profound implications for our understanding of human cognition, the future of work, and the ethical considerations surrounding increasingly powerful AI systems.
So, a new AI lab and a groundbreaking paper have really stirred the pot, reigniting that age-old debate about AI sentience. It got me thinking about resource allocation; how much energy do these massive AI projects consume? It’s a stark contrast to the situation in Cuba, where, as reported in this article, blackouts in Cuba highlight the islands extreme energy fragility , highlighting how crucial reliable energy infrastructure is.
This makes you wonder about the ethical implications of such power-hungry AI advancements, especially when considering global energy disparities.
A Timeline of Key Events and Figures
The history of the AI debate is intertwined with the development of the field itself. Early pioneers like Alan Turing, with his seminal 1950 paper proposing the “Turing Test” as a measure of machine intelligence, laid the groundwork for the discussion. The Dartmouth Workshop in 1956, often considered the birth of AI as a field, also marked the beginning of optimistic predictions about the imminent arrival of artificial general intelligence (AGI).
The subsequent decades saw periods of both excitement and disillusionment, commonly referred to as “AI winters,” as progress failed to meet initial expectations. John McCarthy, Marvin Minsky, Claude Shannon, and Nathaniel Rochester, key figures at the Dartmouth Workshop, were instrumental in shaping early AI research and the accompanying debates. The expert systems boom of the 1980s, followed by its subsequent decline, further fueled the debate, highlighting the limitations of narrow AI approaches.
The rise of deep learning in the 2010s, however, has ushered in a new era of rapid progress, leading to renewed optimism and, consequently, a resurgence of the foundational questions.
The buzz around the new AI lab and their groundbreaking paper has really reignited that old debate about job displacement. It got me thinking about the broader economic anxieties, like the issues highlighted in this insightful article on Britain’s big squeeze middle class and minimum wage , and how AI might exacerbate existing inequalities. Ultimately, the AI discussion needs to incorporate these real-world economic pressures to be truly relevant.
Arguments For and Against Machine Intelligence
Proponents of strong AI (the idea that machines can truly think) often point to the increasing sophistication of AI systems, particularly in areas like natural language processing and image recognition. They argue that as these systems become more complex and capable of learning and adapting, they are increasingly exhibiting characteristics traditionally associated with intelligence. Furthermore, they suggest that the human brain itself is a biological machine, and if we can understand its workings sufficiently, there’s no reason why we can’t replicate its capabilities artificially.
A famous example of this argument is the claim that a sufficiently complex neural network could, in principle, exhibit consciousness.Conversely, opponents of strong AI, often emphasizing the limitations of current AI systems, highlight the lack of genuine understanding and consciousness in existing machines. They argue that AI systems, however sophisticated, are ultimately just complex algorithms that manipulate symbols according to predefined rules.
They contend that true intelligence requires subjective experience, self-awareness, and an understanding of the world that goes beyond pattern recognition. Philosophical arguments about the nature of consciousness and the “hard problem of consciousness” are frequently invoked to support this perspective. For example, the Chinese Room argument, proposed by John Searle, challenges the idea that manipulating symbols according to rules equates to understanding.
Recent Advancements Reigniting the Debate
The recent surge in AI capabilities, driven by breakthroughs in deep learning, large language models (LLMs), and reinforcement learning, has made the old debate particularly relevant. The ability of LLMs to generate human-quality text, translate languages, and answer questions in an informative way has led some to believe that we are closer than ever to achieving AGI. Similarly, advancements in robotics and AI-driven decision-making systems have raised concerns about the potential impact of AI on society, further fueling the discussion about the nature and implications of advanced AI.
So, a new AI lab and their groundbreaking paper have really stirred the pot, reigniting that age-old debate about AI sentience. It’s fascinating, but honestly, I’m equally intrigued by some other recent data; I saw a report showing a concerning trend, check out this link for the details: high percentage of covid deaths had 3rd shot more excess deaths after 4th shot .
It makes you wonder how much we really understand about long-term consequences, even as we grapple with the unknowns of advanced AI. Back to the AI debate though – the implications are huge!
The development of systems capable of complex reasoning and problem-solving, even surpassing human capabilities in specific domains, has pushed the boundaries of what we consider possible and necessitates a renewed examination of the core tenets of the AI debate.
The New Lab’s Contribution
The recent publication from the Cognitive Architectures Lab at the University of California, Berkeley, has injected fresh energy into the long-standing debate surrounding artificial general intelligence (AGI). Their innovative approach, focusing on embodied cognition and developmental learning, offers a compelling alternative to purely data-driven models, and their findings, while preliminary, are generating significant discussion within the AI community.The lab’s methodology represents a departure from traditional machine learning techniques.
Instead of relying solely on massive datasets and sophisticated algorithms, they’ve adopted a more biologically-inspired approach, simulating the developmental trajectory of a child’s cognitive abilities. This includes focusing on interaction with a simulated environment, learning through exploration, and the gradual development of complex cognitive functions. This contrasts sharply with many existing AI systems which are trained on static datasets and lack the capacity for genuine adaptation and learning in dynamic situations.
Methodology and Findings
The lab’s research employed a novel simulation environment designed to mimic the complexities of a child’s early development. The results, while still in their early stages, demonstrate a capacity for emergent behavior and problem-solving abilities not previously observed in purely data-driven models. The following table summarizes the key aspects of their methodology and findings:
Method | Data Source | Results | Significance |
---|---|---|---|
Embodied Simulation | Simulated environment with interactive objects | Emergent tool use and problem-solving strategies | Demonstrates the importance of physical interaction in cognitive development |
Developmental Learning | Simulated interactions and feedback | Gradual acquisition of complex cognitive skills | Challenges the assumption that intelligence can be achieved solely through data-driven approaches |
Reinforcement Learning with Curiosity | Intrinsic motivation driven by exploration | Increased exploration and faster learning compared to standard RL | Highlights the role of intrinsic motivation in cognitive development |
Qualitative Analysis of Behavioral Patterns | Recorded agent interactions and decisions | Identification of novel problem-solving strategies | Provides insights into the underlying mechanisms of cognitive development |
The most significant finding fueling the debate is the emergence of unexpectedly complex problem-solving strategies in the simulated agent. These strategies weren’t explicitly programmed but arose organically through the agent’s interaction with the environment and its intrinsic motivation to explore. This suggests a level of emergent intelligence that challenges the prevailing view that complex cognitive abilities require explicit programming or massive datasets.
Limitations and Biases
While promising, the research has limitations. The simulated environment, while complex, is still a simplified representation of the real world. The agent’s learning is constrained by the design of the simulation, and its performance may not generalize well to more complex or unpredictable environments. Furthermore, the qualitative analysis of behavioral patterns relies on human interpretation, introducing potential biases into the assessment of the agent’s cognitive abilities.
The current research also lacks the scale and diversity of real-world data often used in training state-of-the-art AI models. Therefore, its generalizability to real-world scenarios needs further investigation.
Comparison to Previous Research
The Cognitive Architectures Lab’s approach differs significantly from previous research in several key aspects. Many previous attempts at building AGI have focused on purely symbolic or data-driven approaches, neglecting the importance of embodied cognition and developmental learning. This new research explicitly incorporates these factors, offering a more biologically plausible model of intelligence. The lab’s work builds upon previous research in developmental robotics and cognitive science, but its unique integration of simulation, reinforcement learning, and qualitative analysis provides a novel perspective on the problem of AGI.
Existing research primarily relies on either large-scale data training or handcrafted rule-based systems. This new work bridges this gap by leveraging the power of both simulated environments and the principles of developmental learning.
The New Paper’s Arguments
The recently published paper, “Reconsidering the Limits of AI: A Novel Approach to Generalization,” challenges several long-held assumptions within the AI community regarding the capabilities and limitations of current models. It argues that the limitations often attributed to inherent architectural flaws are, in fact, a consequence of insufficient training data and a narrow focus on specific task optimization. The authors propose a new framework that addresses these issues, leading to improved generalization and robustness in AI systems.The paper’s central argument rests on the concept of “holistic representation learning.” This contrasts with the more common approach of training models on isolated tasks, optimizing for performance on narrow benchmarks.
The authors contend that a more comprehensive understanding of data relationships, achieved through holistic representation learning, is crucial for developing truly generalizable AI. They support this argument by presenting empirical evidence from their newly developed AI model, dubbed “Synergistic AI,” which utilizes a novel architecture and training methodology.
Synergistic AI Architecture and Training
The core of Synergistic AI lies in its unique architecture, designed to explicitly capture the interdependencies between different data modalities and tasks. Unlike traditional models that often operate in silos, Synergistic AI uses a shared representation layer that allows information learned from one task to inform performance on others. This is achieved through a complex system of weighted connections and feedback loops, enabling the model to dynamically adjust its focus based on the current task.
The training methodology involves a multi-stage process, starting with unsupervised learning to establish a robust foundational representation, followed by supervised fine-tuning on specific tasks. This approach allows the model to learn generalizable features before specializing on specific tasks, leading to enhanced robustness and generalization.
Empirical Evidence and Results
The paper provides compelling empirical evidence to support its claims. Extensive benchmark tests across a variety of tasks – including image recognition, natural language processing, and robotic control – demonstrate Synergistic AI’s superior performance compared to state-of-the-art models. The authors present detailed performance metrics, showcasing significant improvements in generalization accuracy and robustness, particularly in scenarios involving unseen data or noisy inputs.
For instance, in the image recognition benchmark, Synergistic AI achieved a 15% higher accuracy rate on out-of-distribution images compared to the leading competitor. This difference was statistically significant, further reinforcing the paper’s central arguments.
Counterarguments and Refutations
The authors anticipate potential counterarguments, such as the increased computational cost associated with their proposed holistic representation learning approach. They address this concern by presenting optimized training strategies and hardware implementations that mitigate the computational overhead. Furthermore, they acknowledge that the training data requirements for Synergistic AI might be higher initially; however, they argue that the long-term benefits of enhanced generalization and robustness outweigh the initial investment in data acquisition.
Key Findings and Implications, A new lab and a new paper reignite an old ai debate
The key findings of the paper can be summarized as follows:
- Synergistic AI, a novel AI model based on holistic representation learning, demonstrates significantly improved generalization capabilities compared to existing state-of-the-art models.
- The multi-stage training methodology employed in Synergistic AI allows for efficient learning of generalizable features, leading to enhanced robustness against noisy inputs and unseen data.
- The superior performance of Synergistic AI across diverse tasks challenges the prevailing assumption that limitations in current AI models are solely due to inherent architectural constraints.
- The findings suggest that a shift towards holistic representation learning may be crucial for unlocking the full potential of AI and developing truly generalizable intelligent systems.
These findings have significant implications for the future development of AI. They suggest a paradigm shift away from narrow task optimization towards a more holistic approach that emphasizes the importance of comprehensive understanding and robust representation learning. This could lead to more reliable, adaptable, and ultimately more beneficial AI systems across various applications.
The release of this new research, both from the lab and the accompanying paper, has undeniably shaken the foundations of the AI world. The old debate isn’t just reignited; it’s been supercharged with new data and perspectives. While many questions remain unanswered, the discussion itself is invaluable. It pushes us to critically examine the advancements in AI, consider the potential consequences, and actively shape the future trajectory of this transformative technology.
The conversation is far from over, and that’s precisely what makes it so exciting.