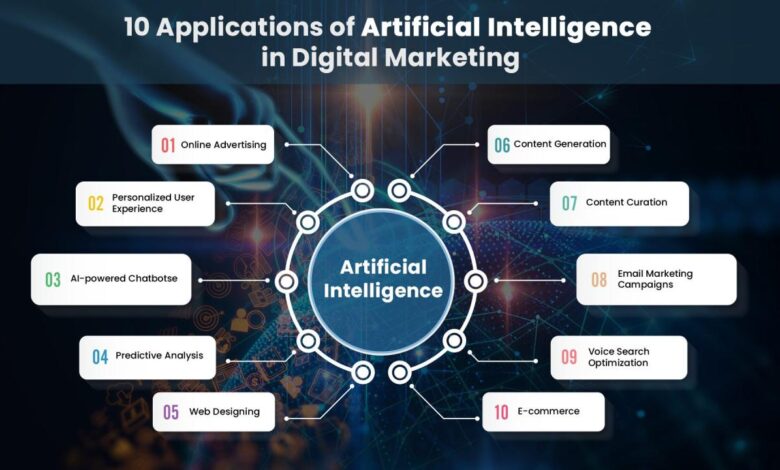
AI Can Predict Tipping Points Before They Happen
Ai can predict tipping points before they happen – AI can predict tipping points before they happen – a statement that sounds like science fiction, but is rapidly becoming reality. Imagine predicting societal shifts, environmental collapses, or even market crashes
-before* they occur, giving us precious time to prepare and mitigate potential damage. This isn’t about fortune telling; it’s about leveraging the power of artificial intelligence to analyze massive datasets and identify patterns that foreshadow critical transitions.
This post explores how AI is being used to predict these pivotal moments and the ethical considerations that arise.
We’ll delve into the different AI algorithms employed, the crucial data sources needed, and the inherent limitations of this technology. We’ll examine both successful and unsuccessful predictions, highlighting the importance of understanding biases and uncertainties. Finally, we’ll discuss the future of AI-driven tipping point prediction and the crucial research still needed to refine this groundbreaking field.
Defining Tipping Points in Various Contexts
Tipping points represent critical thresholds beyond which a system undergoes a fundamental shift, often irreversible. Understanding these points is crucial for effective intervention and mitigation across numerous disciplines. While predicting them precisely remains challenging, recognizing their characteristics and employing various methodologies enhances our ability to anticipate and manage their impact.Tipping points are characterized by a relatively small change in an input variable triggering a disproportionately large and often abrupt shift in the system’s state.
This nonlinear response is key to their definition and distinguishes them from gradual, predictable changes. The system’s trajectory beyond the tipping point can be difficult, if not impossible, to reverse, leading to significant consequences.
Tipping Points in Environmental Science
Environmental systems are particularly susceptible to tipping points. For instance, the melting of Arctic sea ice exemplifies this phenomenon. As temperatures rise, the ice melts, reducing the reflective surface (albedo) and accelerating further warming. This positive feedback loop can lead to a dramatic and potentially irreversible decline in Arctic ice cover, with far-reaching consequences for global climate patterns and ecosystems.
Another example is the Amazon rainforest. Deforestation and climate change push the rainforest towards a tipping point where it could transition to a savanna-like ecosystem, significantly impacting biodiversity and carbon sequestration. The characteristics defining these tipping points include critical thresholds in temperature, deforestation rates, and ice extent, leading to abrupt shifts in ecosystem structure and function. Identifying these thresholds often involves complex climate models and analyzing historical data on ecosystem changes.
Tipping Points in Economics, Ai can predict tipping points before they happen
Economic systems are also prone to tipping points. The 2008 global financial crisis serves as a prime example. A relatively small event, the collapse of Lehman Brothers, triggered a cascade of failures throughout the financial system, leading to a global recession. Characteristics of this tipping point included interconnectedness of financial institutions, excessive leverage, and a lack of regulatory oversight.
Methodologies for identifying potential economic tipping points often involve analyzing financial market data, identifying systemic risks, and developing stress tests to evaluate the resilience of the financial system. Early warning systems, based on indicators like credit spreads and market volatility, can help predict potential crises, though perfect prediction remains elusive.
Tipping Points in Social Trends
Social trends can also exhibit tipping points. The rapid adoption of a new technology, like the internet or smartphones, demonstrates this. Initially, adoption might be slow, but once a critical mass of users is reached, adoption accelerates dramatically, leading to a fundamental shift in social interaction and communication. The characteristics here often include network effects, social influence, and technological advancements.
Identifying potential tipping points in social trends involves analyzing social network data, tracking the spread of information and behaviors, and utilizing agent-based models to simulate social dynamics. While predicting the exact timing of these shifts remains challenging, identifying trends and key influencers can provide insights into potential future shifts.
Visualizing Tipping Point Predictions: Ai Can Predict Tipping Points Before They Happen
Visualizing the complex probabilities surrounding tipping points requires careful consideration of both the predicted likelihood of the event and the inherent uncertainty in the AI’s predictions. Effective visualizations can help us understand the risk and guide decision-making, especially in scenarios with potentially catastrophic consequences. We need to move beyond simple binary predictions (tipping point will/will not occur) and embrace representations that capture the nuanced nature of these predictions.Effective visualization of tipping point predictions involves representing the probability of a tipping point occurring over time, along with the uncertainty inherent in those predictions.
This allows for a more nuanced understanding of the risk, moving beyond a simple yes/no answer.
Probability of Tipping Point Over Time
Imagine a graph with time on the x-axis and probability on the y-axis, ranging from 0 to 1 (or 0% to 100%). A line on this graph would show the predicted probability of a tipping point occurring at any given time. For instance, if we’re modeling the melting of the Greenland ice sheet, the line might start low, gradually increase over several decades, then potentially accelerate sharply as the ice sheet reaches a critical threshold.
Initially, the line might rise slowly, reflecting a low probability of a tipping point in the near future. As time progresses and conditions worsen, the line would climb more steeply, indicating an increasing likelihood of a tipping point occurring within a specific timeframe. The line wouldn’t necessarily reach 100%, reflecting the inherent uncertainty and the possibility of mitigating factors.
The shape of this line, its slope, and its eventual plateau or peak are all crucial indicators of the predicted risk. A sudden, steep increase would represent a rapidly approaching tipping point, while a gradual increase suggests more time to react. This visual representation provides a clear and intuitive understanding of the evolving risk over time. Consider, for example, a prediction of Amazon rainforest dieback.
The graph would show a gradual increase in probability over decades, potentially accelerating due to deforestation and climate change, until it plateaus at a high probability, representing the likelihood of significant dieback occurring within a certain timeframe.
Visualizing Uncertainty in AI Predictions
The inherent uncertainty in AI-based predictions should be explicitly visualized. One effective method is to use shaded regions around the probability line described above. The width of the shaded region would represent the confidence interval – a wider region indicates higher uncertainty, while a narrower region suggests greater confidence in the prediction. For instance, in the early stages of prediction, when data is scarce, the shaded region would be wider, reflecting the greater uncertainty.
As more data becomes available and the AI model refines its predictions, the shaded region would narrow, indicating increased confidence. This visual representation clearly communicates the level of uncertainty associated with the prediction, allowing stakeholders to interpret the results with appropriate caution. Furthermore, different colors could represent different sources of uncertainty, such as uncertainty in model parameters, uncertainty in input data, or uncertainty related to future external factors.
For example, a darker shade might represent uncertainty stemming from unpredictable weather patterns, while a lighter shade could represent uncertainty due to limitations in the data used to train the AI model. This layered approach to uncertainty visualization would provide a comprehensive picture of the risks involved. The Greenland ice sheet example could show a wider confidence interval initially, reflecting uncertainty in future greenhouse gas emissions and their impact on ice melt.
As more data becomes available, and as climate models improve, this confidence interval would narrow.
The ability of AI to predict tipping points holds immense potential for shaping a more proactive and resilient future. While challenges remain, particularly regarding data biases and ethical considerations, the progress made is undeniably impressive. By understanding the strengths and limitations of AI in this context, we can harness its power responsibly, mitigating risks and potentially avoiding catastrophic outcomes.
The journey to perfecting AI-driven tipping point prediction is ongoing, but the potential rewards—a world better prepared for significant change—make it a pursuit well worth undertaking.
AI’s ability to predict tipping points is fascinating; imagine its potential in financial markets! For example, understanding why bond investors soured on France, as detailed in this insightful article how bond investors soured on france , could be significantly enhanced with AI’s predictive capabilities. Early warning systems, powered by AI, could potentially flag such shifts before they impact global markets, preventing major financial disruptions.
AI’s ability to predict tipping points is pretty mind-blowing, isn’t it? Think about how much we could prevent if we knew a societal shift was coming – like a sudden market crash or even a social upheaval. It’s fascinating to consider this in contrast to the mystery surrounding language itself; I recently read an article on plenty of words have no clear origin , which highlights how much we *don’t* know about seemingly simple things.
Perhaps AI can bring some of that same predictive power to understanding the evolution of language too, ultimately helping us anticipate and even navigate those unpredictable societal shifts.
AI’s ability to predict tipping points is pretty mind-blowing, isn’t it? Thinking about how it could help us avoid major societal shifts makes me wonder about the underlying causes of those shifts. It’s fascinating to consider that, as this article suggests, one of history’s biggest drivers is not what you might think , and that AI might be able to identify these hidden drivers before they trigger a crisis.
Ultimately, accurate prediction of these tipping points could lead to more proactive and effective interventions.