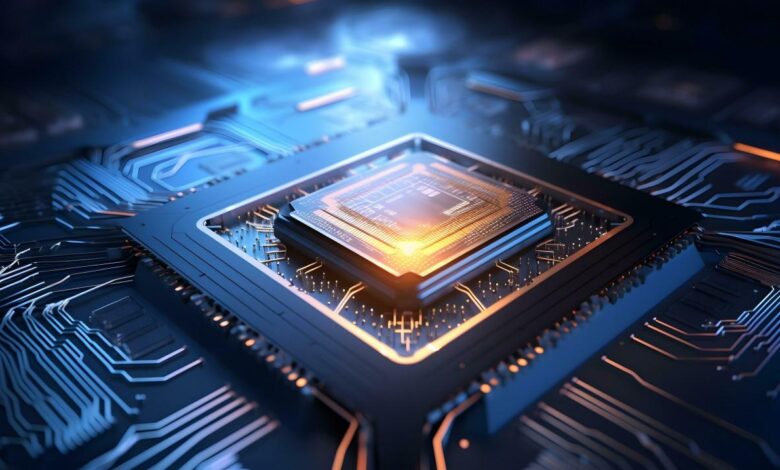
AI Has Returned Chipmaking to the Heart of Computer Technology
AI has returned chipmaking to the heart of computer technology. It’s a thrilling time for the tech world! We’re witnessing a renaissance in chip design and manufacturing, all thanks to the incredible advancements in artificial intelligence. No longer a futuristic fantasy, AI is actively shaping the chips powering our devices, from smartphones to supercomputers. This isn’t just about incremental improvements; it’s a fundamental shift in how we create the very building blocks of our digital lives.
Think faster, more efficient, and incredibly powerful chips – all powered by AI.
This resurgence isn’t entirely new; AI has dabbled in chipmaking for years. But recent breakthroughs in machine learning, particularly deep learning, have unleashed its true potential. AI algorithms are now capable of designing chips far more complex and efficient than anything humans could create alone. This translates to smaller, faster, and more energy-efficient devices, pushing the boundaries of what’s technologically possible.
We’re talking about a revolution in miniaturization and performance, and it’s all happening right now.
The resurgence of AI in chip manufacturing
For decades, chip design has relied heavily on human expertise and increasingly complex, but ultimately deterministic, algorithms. However, the exponential growth in data and computing power, coupled with advancements in machine learning, has sparked a remarkable resurgence of artificial intelligence in this crucial technological domain. This isn’t simply a return to AI’s earlier involvement; it’s a fundamental shift, leveraging the capabilities of AI to tackle previously insurmountable challenges in chip design and manufacturing.
AI’s Historical Involvement in Chipmaking
AI’s initial foray into chip manufacturing was limited. Early applications, dating back to the 1980s and 90s, primarily focused on automating specific tasks within the design process, such as circuit verification or placement and routing. These applications were rule-based systems, far from the sophisticated machine learning models we see today. The computational limitations of the time restricted AI’s role to niche applications, and its impact remained relatively modest.
The complexity of chip design, with billions of transistors, far outstripped the capabilities of the AI systems available at the time.
Recent Advancements Fueling the Resurgence
The recent surge in AI’s influence is driven by several key advancements. Deep learning, particularly convolutional neural networks (CNNs) and recurrent neural networks (RNNs), have proven remarkably effective in analyzing vast datasets of chip designs and identifying optimal configurations. Reinforcement learning algorithms are now used to train AI agents to design chips, iteratively improving their designs based on performance feedback.
Furthermore, advancements in hardware, particularly the development of specialized AI accelerators, have enabled the training and deployment of far more complex AI models than ever before. The availability of massive datasets, generated through simulations and actual manufacturing data, has also been crucial.
Comparison of AI-Driven and Traditional Chip Design
AI-driven chip design offers several advantages over traditional methods. Traditional methods, often reliant on human intuition and heuristic algorithms, struggle to optimize chips for multiple, often conflicting, objectives simultaneously (e.g., performance, power consumption, area). AI, on the other hand, can simultaneously consider numerous parameters and explore a far wider design space, leading to chips with superior performance and efficiency.
Furthermore, AI can automate many tedious and time-consuming tasks, accelerating the design process significantly. However, AI-driven design also presents challenges. The “black box” nature of some AI models can make it difficult to understand the reasoning behind their design choices, potentially hindering debugging and verification. Furthermore, the computational cost of training complex AI models can be substantial.
Timeline of AI’s Contribution to Chip Manufacturing
The following timeline highlights key milestones:
This timeline illustrates the accelerating pace of AI’s integration into chip manufacturing. Early involvement focused on limited automation, while recent years have witnessed a dramatic expansion of AI’s capabilities and impact.
AI’s resurgence in chip design is revolutionizing the tech world, pushing the boundaries of what’s possible. This technological leap forward makes me wonder about the political landscape, and how shifts in power, like the implications of Senator Sinema quitting the Democrat party, the implications of Senator Sinema quitting the Democrat party , could impact funding and regulation for this crucial sector.
Ultimately, the future of AI and chipmaking hinges on both technological innovation and stable political environments.
Year | Milestone | Description |
---|---|---|
1980s-1990s | Early AI applications | Rule-based systems for specific tasks like circuit verification. Limited impact. |
2010s | Emergence of deep learning | Deep learning techniques begin to be applied to chip design problems. Still primarily focused on specific sub-tasks. |
2015-Present | AI-driven design automation | Significant advancements in deep learning and reinforcement learning lead to AI systems capable of designing entire chips. |
2020-Present | AI for manufacturing optimization | AI is increasingly used to optimize manufacturing processes, improving yield and reducing costs. |
AI’s role in chip design and optimization
The semiconductor industry is facing unprecedented challenges in designing ever-smaller, faster, and more power-efficient chips. Traditional design methods are struggling to keep pace with the increasing complexity, leading to longer development cycles and escalating costs. Artificial intelligence (AI) is emerging as a powerful tool to overcome these hurdles, revolutionizing chip design and optimization processes. AI algorithms are proving invaluable in navigating the vast design space, identifying optimal solutions, and accelerating the entire development pipeline.AI algorithms are transforming chip design in several key ways, impacting everything from initial architecture exploration to final verification.
This isn’t simply about automation; AI provides a level of insight and optimization that was previously impossible. The ability to analyze massive datasets and identify intricate patterns allows for breakthroughs in chip efficiency and performance that are significantly beyond human capabilities.
AI-Driven Chip Design Automation
AI is automating many tedious and time-consuming aspects of chip design. For instance, machine learning algorithms can automatically generate circuit layouts, optimizing for factors like area, power consumption, and signal integrity. This automation significantly reduces the workload on human designers, allowing them to focus on higher-level design decisions and more creative problem-solving. Consider the example of floorplanning, where AI can explore millions of possible placements of components on a chip, identifying the most efficient arrangement far faster than any human team.
This translates directly into reduced design time and lower development costs.
AI’s Impact on Reducing Chip Development Time and Cost
The application of AI in chip design directly translates to substantial reductions in both time and cost. By automating repetitive tasks and optimizing designs more effectively, AI significantly shortens the design cycle. A hypothetical scenario illustrates this: Imagine a team designing a high-performance processor. Using traditional methods, the design process might take two years, involving multiple iterations and extensive manual verification.
With AI assistance, this process could be shortened to 18 months, potentially even less, as AI algorithms can quickly identify and correct design flaws, minimizing costly rework. The resulting cost savings are considerable, due to reduced engineering hours and faster time to market.
AI for Optimizing Chip Performance and Power Consumption
AI algorithms are crucial in optimizing chip performance while simultaneously minimizing power consumption. Machine learning models can be trained on vast datasets of existing chip designs and their performance characteristics to predict the impact of design choices on power and performance. This predictive capability allows designers to explore a wider range of design options, identifying optimal trade-offs between performance and power efficiency.
For example, AI can optimize the clock frequency and voltage levels of different parts of the chip, ensuring maximum performance while minimizing energy waste. This is particularly critical for mobile devices and other power-constrained applications.
Accelerated Chip Design: A Hypothetical Scenario
Let’s consider a hypothetical scenario involving the design of a new generation of AI accelerators. Traditionally, designing such a chip would involve extensive manual simulations and optimizations, a process that could easily take several years and involve hundreds of engineers. With AI, the process could be dramatically accelerated. AI algorithms could automatically generate initial chip architectures, optimize the placement and routing of components, and even predict potential performance bottlenecks.
Human designers would then focus on refining the AI-generated design, leveraging the AI’s insights to make informed decisions and address any remaining challenges. This collaborative approach would significantly reduce development time and cost, bringing the new chip to market much faster. Furthermore, the resulting chip would likely be more power-efficient and performant than one designed using traditional methods.
AI’s influence on chip fabrication processes: Ai Has Returned Chipmaking To The Heart Of Computer Technology
The incredibly complex process of chip fabrication, involving hundreds of steps and demanding extreme precision, is undergoing a revolution thanks to artificial intelligence. AI is no longer just a tool for designing chips; it’s actively improving the efficiency, yield, and quality of their manufacture. This enhanced precision translates directly into faster, more powerful, and more reliable chips for everything from smartphones to supercomputers.AI’s application in chip fabrication addresses many long-standing challenges.
Traditional methods often rely on heuristic rules and extensive manual intervention, leading to inconsistencies and difficulties in pinpointing the root causes of defects. AI, with its ability to analyze vast datasets and identify complex patterns, offers a more data-driven and proactive approach.
AI-Driven Precision in Lithography
Lithography, the process of transferring circuit patterns onto silicon wafers, is a critical and incredibly delicate step. Even minute variations in exposure time, laser intensity, or wafer alignment can lead to defects. AI algorithms can analyze real-time data from lithography machines, identifying subtle variations and automatically adjusting parameters to maintain optimal precision. For instance, an AI system might detect a slight drift in the laser alignment and autonomously correct it before it causes a significant number of defective chips.
This leads to a substantial increase in the yield – the percentage of successfully manufactured chips – which directly impacts the overall cost and profitability of production.
AI-Enhanced Defect Detection and Prevention, Ai has returned chipmaking to the heart of computer technology
Traditional defect detection relies heavily on visual inspection, a time-consuming and potentially error-prone process. AI-powered systems, however, can analyze images from various inspection stages, identifying subtle defects invisible to the human eye. These systems can leverage machine learning models trained on vast datasets of images, classifying defects with high accuracy and identifying the root cause of these defects. This predictive capability allows manufacturers to proactively adjust process parameters, preventing defects before they propagate through the manufacturing line.
For example, an AI system might identify a recurring defect pattern linked to a specific step in the etching process, prompting engineers to investigate and adjust the parameters of that step, thereby preventing future defects.
AI’s resurgence in chip design is truly remarkable; it’s pushing the boundaries of what’s possible in computing. This technological leap feels oddly parallel to the human resilience showcased by the first responders, as highlighted in this news report about the President and First Lady visiting Dayton hospital: president and first lady visit victims and thank first responders at dayton hospital.
Both situations demonstrate incredible capabilities – one in the digital realm, the other in the human spirit – both equally vital for our future. The precision of AI in chipmaking mirrors the precision needed in emergency response; both require meticulous planning and execution.
Comparison of AI-Driven and Traditional Quality Control
Aspect | Traditional Approach | AI-Driven Approach |
---|---|---|
Defect Detection | Primarily visual inspection, often slow and prone to human error. Relies on statistical sampling. | Automated image analysis using machine learning, capable of detecting subtle defects with high accuracy. Analyzes 100% of chips. |
Root Cause Analysis | Time-consuming and often relies on expert judgment, making it difficult to pinpoint the exact cause of defects. | AI algorithms analyze vast datasets to identify patterns and correlations, enabling faster and more precise root cause identification. |
Predictive Maintenance | Relies on scheduled maintenance and reactive fixes based on observed failures. | AI predicts potential equipment failures based on sensor data, allowing for proactive maintenance and preventing downtime. |
Yield Improvement | Incremental improvements through iterative process adjustments. | Significant yield improvements through real-time optimization and defect prevention. |
AI’s Role in Predicting and Preventing Defects
AI’s predictive capabilities are transforming chip fabrication by enabling proactive defect prevention. By analyzing data from various sources, including sensor data from manufacturing equipment, process parameters, and historical defect data, AI models can predict the likelihood of defects occurring. This allows manufacturers to take corrective actions before defects actually manifest, minimizing production losses and improving overall yield. For instance, an AI system might predict a high probability of defects in a particular batch of wafers due to variations in temperature and humidity, allowing manufacturers to adjust environmental controls or re-process the wafers before significant defects occur.
This proactive approach drastically reduces the costs associated with scrap and rework, leading to significant economic benefits.
The economic and societal impact of AI in chipmaking
The integration of artificial intelligence into chip manufacturing is poised to revolutionize the semiconductor industry, triggering a cascade of economic and societal effects that will reshape global markets and labor dynamics. This shift promises unprecedented advancements in chip design and production efficiency, but also presents challenges related to workforce adaptation and the potential for increased economic disparity.AI’s influence on chipmaking extends far beyond mere automation; it’s fundamentally altering the innovation landscape and the very nature of competition within the sector.
AI’s Drive on Innovation and Competition
The application of AI in chip design allows for the exploration of a vastly larger design space than previously possible. AI algorithms can optimize chip layouts for power efficiency, performance, and area, leading to the creation of more powerful and energy-efficient chips. This accelerated innovation cycle fosters a more competitive environment, pushing companies to continuously improve their AI-driven design processes to maintain a leading edge.
AI’s resurgence in chipmaking is revolutionizing the tech world, impacting everything from smartphones to supercomputers. It’s a stark contrast to the human element highlighted in the news, like the recent ice raids on Mississippi food processing plants resulting in 680 arrests , which underscores the complexities of our globalized economy. Ultimately, though, AI’s impact on chip production will likely reshape how we interact with technology for years to come.
For example, companies like Google and Nvidia are heavily investing in AI-powered chip design, leading to a rapid advancement in the capabilities of their processors. This competition ultimately benefits consumers through the availability of more advanced and affordable technology.
Implications for Global Supply Chains
AI-driven automation in chip fabrication has the potential to significantly impact global supply chains. By improving manufacturing yields and reducing production times, AI can mitigate the effects of geopolitical instability and natural disasters on chip production. However, increased reliance on AI-powered systems also introduces new vulnerabilities. Cybersecurity threats and the potential for AI-driven disruptions to manufacturing processes need to be carefully addressed.
A reliance on a few key AI algorithm providers could also create new chokepoints in the supply chain. For instance, a disruption to a major AI algorithm provider could significantly impact the production capabilities of numerous chip manufacturers.
Job Market Impacts of AI Adoption
The adoption of AI in chip manufacturing will undoubtedly transform the job market. While some jobs may be displaced by automation, new roles focused on AI development, maintenance, and oversight will emerge. The overall impact on employment will depend on the pace of AI adoption and the ability of the workforce to adapt to the changing demands of the industry.
Reskilling and upskilling initiatives will be crucial to ensure a smooth transition and minimize job displacement. For example, while jobs involving repetitive manual tasks in fabrication plants may decrease, there will be a growing need for specialists in AI-driven process optimization, data analysis, and algorithm development.
Projected Economic Benefits of AI in Chipmaking (Next Decade)
Year | Increased Productivity (%) | Reduced Manufacturing Costs (%) | Market Expansion (Billions USD) |
---|---|---|---|
2024 | 5 | 3 | 50 |
2027 | 10 | 7 | 150 |
2030 | 15 | 12 | 300 |
2033 | 20 | 18 | 500 |
Note
These figures are estimates based on current trends and expert projections and may vary depending on various factors. The actual economic benefits could be significantly higher or lower. These figures are illustrative and not intended as precise predictions. They reflect a general trend of increased productivity and market expansion due to AI adoption.
Future trends and challenges in AI-driven chip manufacturing
The integration of artificial intelligence (AI) into chip manufacturing is still in its nascent stages, yet its potential to revolutionize the industry is undeniable. The next 5-10 years promise significant advancements, pushing the boundaries of what’s possible in terms of chip design, fabrication, and overall efficiency. However, alongside these exciting prospects lie significant challenges and ethical considerations that must be carefully addressed.
The coming decade will witness a dramatic increase in the sophistication and application of AI across the entire chipmaking lifecycle. We’re moving beyond simple automation to genuinely intelligent systems capable of optimizing processes in real-time, predicting and preventing defects, and even designing entirely new chip architectures. This shift will be driven by advancements in machine learning algorithms, particularly deep learning and reinforcement learning, combined with the increasing availability of vast datasets generated during chip manufacturing.
Advancements in AI for Chipmaking
AI’s role in chip design will expand significantly. We can expect AI-powered tools to automate the tedious and complex process of designing intricate chip layouts, optimizing for performance, power consumption, and area. This will accelerate the design cycle, allowing for faster innovation and quicker time-to-market for new chips. Furthermore, AI will play a crucial role in materials science, helping to discover and optimize new materials for improved chip performance and reliability.
For example, AI algorithms could analyze vast datasets of material properties to predict the performance of novel semiconductor materials, accelerating the development of more energy-efficient and powerful chips. This is similar to how AI is already being used in drug discovery to identify promising candidate molecules.
Challenges and Limitations of AI-driven Chip Manufacturing
The heavy reliance on AI in chip manufacturing presents several challenges. Data security and intellectual property protection are paramount concerns. The massive datasets used to train and operate AI systems contain highly sensitive information about chip designs and manufacturing processes. Robust security measures are crucial to prevent data breaches and intellectual property theft. Another challenge lies in the explainability and trustworthiness of AI algorithms.
Understanding why an AI system made a particular decision is critical, especially in a high-stakes environment like chip manufacturing. If an AI system makes a mistake, it’s crucial to be able to understand the reasoning behind the error to prevent future occurrences. This “black box” nature of some AI algorithms can be a significant hurdle. Finally, the high cost of implementing and maintaining AI-driven systems could be a barrier for smaller companies, potentially exacerbating existing inequalities in the industry.
Ethical Considerations in AI-driven Chip Manufacturing
The ethical implications of using AI in chip manufacturing are substantial. Bias in training data could lead to unfair or discriminatory outcomes. For instance, if the training data predominantly reflects the experiences of a particular group of engineers, the AI system might inadvertently favor certain design choices or manufacturing processes, potentially leading to biases in chip performance or reliability.
Furthermore, the potential for job displacement due to automation needs careful consideration. Strategies for retraining and upskilling workers are crucial to mitigate the negative social impacts of AI-driven automation in the chip manufacturing industry. Transparency and accountability are essential to ensure the ethical use of AI in this critical sector. Independent audits and rigorous testing procedures are necessary to ensure fairness and prevent unintended consequences.
AI’s Revolution in Other Technology Areas
The advancements in AI-driven chip manufacturing will have a ripple effect across numerous technology sectors. More efficient and powerful chips will enable breakthroughs in areas such as artificial general intelligence, autonomous vehicles, high-performance computing, and medical imaging. For example, improvements in chip performance could significantly enhance the capabilities of medical imaging systems, allowing for earlier and more accurate diagnosis of diseases.
Similarly, faster and more energy-efficient chips will be essential for the development of truly autonomous vehicles, enabling real-time processing of vast amounts of sensor data. The advancements in chip technology driven by AI will fuel innovation across a wide range of fields, shaping the future of technology in profound ways.
Case studies of successful AI applications in chipmaking
The integration of artificial intelligence (AI) into chip manufacturing is no longer a futuristic concept; it’s a rapidly evolving reality. Several companies are leveraging AI’s power to optimize processes, improve yields, and accelerate innovation. The following case studies highlight some notable successes and the challenges overcome in the journey towards AI-driven chipmaking.
Nvidia’s AI-Powered Chip Design
Nvidia, a leader in GPU technology, has extensively integrated AI into its chip design process. They utilize machine learning algorithms, specifically deep learning models, to automate various stages of design, from initial architecture exploration to detailed circuit layout. These AI models analyze vast datasets of previous designs, identifying optimal configurations and predicting performance characteristics. The outcome is a significant reduction in design time and improved chip performance.
For instance, their AI tools have helped to optimize power consumption while simultaneously increasing processing speed. One challenge Nvidia faced was the need for extremely powerful computing resources to train and run these complex AI models, requiring significant investment in high-performance computing infrastructure.
TSMC’s AI-Driven Predictive Maintenance
Taiwan Semiconductor Manufacturing Company (TSMC), the world’s largest dedicated independent semiconductor foundry, employs AI for predictive maintenance of its sophisticated fabrication equipment. They utilize machine learning algorithms to analyze sensor data from their manufacturing equipment, identifying patterns that indicate potential failures before they occur. This proactive approach minimizes downtime, reduces maintenance costs, and improves overall production efficiency. The AI system analyzes terabytes of data, identifying subtle anomalies that might be missed by human operators.
A significant challenge TSMC faced was integrating the AI system with their existing legacy systems, requiring substantial effort in data integration and system compatibility.
Intel’s AI-Enhanced Process Control
Intel, a major player in the semiconductor industry, is leveraging AI to optimize its chip fabrication processes. They are using machine learning to fine-tune various parameters within their manufacturing facilities, such as temperature, pressure, and chemical composition. This results in improved yield, reduced defects, and enhanced product quality. For example, AI algorithms can analyze real-time data from the fabrication process and adjust parameters in real-time to compensate for variations and maintain optimal conditions.
A key challenge for Intel has been ensuring the reliability and robustness of their AI-based process control systems, requiring rigorous validation and testing to avoid unintended consequences.
Visual representation of AI’s impact
An infographic depicting AI’s role in chip manufacturing would ideally be a multi-stage flowchart, visually representing the journey of a chip from design to production. Each stage would showcase how AI algorithms are integrated to improve efficiency, reduce defects, and accelerate the overall process. The use of color-coding, icons, and clear data points would make the infographic easily understandable and impactful.The infographic would begin with the design phase, highlighting AI’s contribution to automating the design process, optimizing circuit layouts, and predicting potential performance bottlenecks.
This would be followed by the fabrication stage, where AI’s role in process control, defect detection, and yield optimization would be visually emphasized. Finally, the testing and packaging phases would show how AI helps streamline quality control, improve testing efficiency, and optimize packaging designs. Each stage would include quantifiable data, such as percentage improvements in yield, reduction in defect rates, or speed enhancements.
AI-Enhanced Chip Design
This section of the infographic would depict the chip design stage, showcasing AI’s role in automating layout generation and optimization. A visual representation could be a side-by-side comparison: one side showing the traditional, manual design process, potentially cluttered and less efficient, and the other side showing an AI-optimized design, clean, efficient, and with optimized power consumption. Data points could include: “AI reduces design time by 30%,” “AI improves power efficiency by 15%,” and “AI increases circuit density by 10%,” illustrated with bar graphs or pie charts.
The colors used could represent different aspects, for example, green for efficiency gains and blue for time savings.
AI in Chip Fabrication
This section would focus on the manufacturing process. An illustration could show a simplified schematic of a fabrication plant, with AI algorithms overlaid as nodes influencing various aspects, such as temperature control, material deposition, and lithography. Data could be presented using a combination of charts and icons: “AI reduces defect rates by 25%,” represented by a downward-trending line graph; “AI improves yield by 10%,” represented by an upward-trending bar graph; and “AI optimizes process parameters in real-time,” shown using an icon of a computer screen displaying data streams and AI-driven adjustments.
Color-coding could be used to highlight different aspects of the fabrication process, for example, yellow for lithography and red for etching.
AI in Chip Testing and Packaging
This final section would showcase AI’s role in automated testing and optimized packaging. A visual could show a flowchart of the testing process, with AI integrated at various points for automated defect detection and analysis. The packaging section could visually represent AI’s contribution to optimizing the design and placement of components, leading to improved performance and reliability. Data points could include: “AI accelerates testing time by 20%,” shown with a timer icon; “AI improves accuracy of defect detection by 15%,” illustrated with a percentage increase; and “AI optimizes packaging efficiency by 5%,” represented by a before-and-after comparison of packaging designs.
The colors could be consistent with the previous sections to maintain visual coherence.
The integration of AI into chipmaking isn’t just a technological marvel; it’s a game-changer with far-reaching implications. From accelerating innovation and boosting global competitiveness to transforming the semiconductor industry’s landscape, AI’s impact is undeniable. While challenges remain, the future looks incredibly bright. We can anticipate even more groundbreaking advancements in the years to come, leading to faster, more powerful, and more sustainable technology that will reshape our world in ways we can only begin to imagine.
The era of AI-driven chip manufacturing is upon us, and it’s only just beginning.