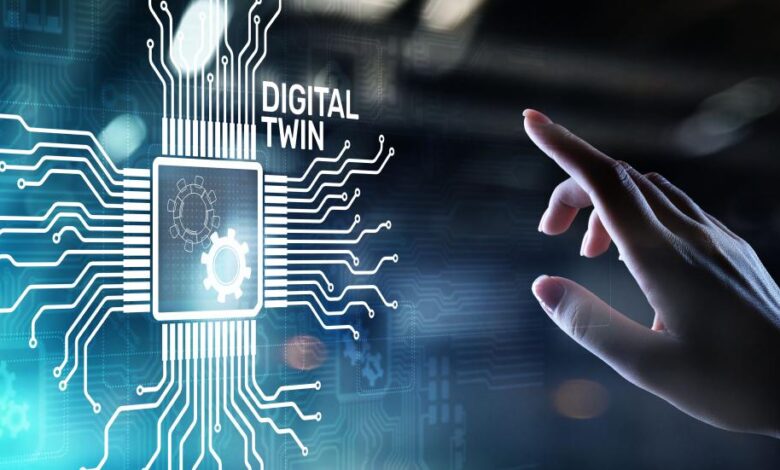
Digital Twins Are Enabling Scientific Innovation
Digital twins are enabling scientific innovation in ways we’re only beginning to understand. Imagine a perfect digital replica of a complex system – a human heart, a climate model, or even a new material – allowing scientists to run experiments, test hypotheses, and predict outcomes without the limitations of the physical world. This technology is revolutionizing fields from medicine to materials science, promising faster discoveries and more efficient solutions to some of humanity’s biggest challenges.
It’s a fascinating journey into the future of research, and one I’m excited to explore with you.
This post delves into the core concepts of digital twins, exploring their applications in various scientific disciplines. We’ll examine how they’re accelerating scientific discovery, tackling complex problems that were previously intractable. We’ll also discuss the crucial role of data management and visualization in making sense of the vast amounts of information generated by these digital counterparts. Finally, we’ll look ahead to the future, imagining the transformative potential of digital twins in shaping scientific research for years to come.
Data Integration and Management for Digital Twins in Science
Building effective scientific digital twins requires a robust strategy for data integration and management. The complexity and heterogeneity of scientific data present significant challenges, demanding careful consideration of data types, integration methods, and security protocols. This section explores these critical aspects, focusing on best practices for ensuring the accuracy, reliability, and longevity of scientific digital twin initiatives.
Types of Data Required for Scientific Digital Twins
Comprehensive scientific digital twins necessitate the integration of diverse data sources. These can broadly be categorized into experimental data, simulation data, and observational data. Experimental data encompasses results from laboratory experiments, field studies, and clinical trials, often including high-throughput data from genomics, proteomics, and other “omics” fields. Simulation data, generated from computational models and simulations, provides insights into complex systems and processes.
Observational data includes real-world measurements from sensors, satellites, and other monitoring devices, offering crucial context and validation for experimental and simulated data. The specific data types needed will vary depending on the scientific domain and the research questions being addressed; for example, a digital twin of a glacier might incorporate remote sensing data (satellite imagery, LiDAR), meteorological data (temperature, precipitation), and ice flow models, whereas a digital twin of a human heart might integrate ECG data, MRI scans, and patient medical history.
Methods for Integrating Heterogeneous Data Sources
Integrating the diverse data types mentioned above requires sophisticated techniques. Data standardization is a crucial first step, involving the conversion of data into a common format and structure. This often involves using ontologies and controlled vocabularies to ensure semantic interoperability. Data integration platforms, such as those based on graph databases or semantic web technologies, provide powerful tools for managing and querying interconnected data.
These platforms enable the establishment of relationships between different data sources, facilitating complex analyses and the generation of holistic insights. API-driven integration is another common approach, allowing seamless data exchange between different systems and applications. For example, a climate model might be linked to a sensor network via APIs, allowing real-time updates to the model based on observed environmental conditions.
Finally, data fusion techniques can combine data from multiple sources to create a more comprehensive and accurate representation of the system being modeled.
Data Management Strategy for Scientific Digital Twins
A robust data management strategy is essential for ensuring the accuracy, reliability, and security of data used in scientific digital twins. This includes implementing rigorous data quality control measures to detect and correct errors, establishing version control systems to track changes and maintain data provenance, and employing robust security protocols to protect sensitive data from unauthorized access or modification.
Data governance frameworks, which define roles, responsibilities, and processes for data management, are crucial for maintaining data integrity and compliance with relevant regulations. Regular data backups and disaster recovery plans are also vital to ensure data availability and prevent data loss. Furthermore, metadata management is crucial, as comprehensive metadata facilitates data discovery, reuse, and interoperability. The FAIR principles (Findable, Accessible, Interoperable, Reusable) provide a useful framework for guiding data management practices.
Comparison of Data Management Solutions
Solution Name | Data Types Supported | Scalability | Security Features |
---|---|---|---|
Cloud-based Data Lakes (e.g., AWS S3, Azure Data Lake Storage) | Various (structured, semi-structured, unstructured) | High | Encryption, access control lists, audit trails |
Relational Database Management Systems (e.g., PostgreSQL, MySQL) | Structured data | Moderate to High | Access control, encryption, data validation |
Graph Databases (e.g., Neo4j) | Relationships between data points | Moderate to High | Access control, encryption, data validation |
NoSQL Databases (e.g., MongoDB) | Semi-structured and unstructured data | High | Access control, encryption, data validation |
The Future of Digital Twins in Scientific Innovation: Digital Twins Are Enabling Scientific Innovation
The rapid advancement of digital twin technology is poised to revolutionize scientific research and development in ways we are only beginning to understand. Beyond the current applications in optimizing industrial processes and designing new products, the potential for scientific discovery using digital twins is vast, promising breakthroughs across numerous disciplines. This next phase will see a shift towards more complex, interconnected, and ethically considered digital twin systems.The convergence of several key technological trends will propel this evolution.
Increased computational power, coupled with the rise of artificial intelligence and machine learning, will allow for the creation of significantly more sophisticated and accurate digital twins capable of simulating increasingly complex systems. Furthermore, the expanding availability of high-fidelity data from diverse sources – including sensors, satellites, and high-throughput experiments – will provide the rich datasets necessary to fuel these advanced models.
Enhanced Simulation Capabilities
The future of scientific digital twins lies in their ability to perform highly realistic and predictive simulations. Current limitations in computational power and data availability restrict the complexity and accuracy of these simulations. However, future advancements in quantum computing and high-performance computing (HPC) will enable the creation of digital twins capable of modeling incredibly complex systems, such as the human brain or the global climate, with unprecedented levels of detail and accuracy.
This will allow scientists to explore a wider range of “what-if” scenarios, accelerating the pace of discovery and potentially leading to breakthroughs in areas like drug discovery, materials science, and climate modeling. For instance, a highly detailed digital twin of a human heart could simulate the effects of various medications with far greater precision than current methods, potentially reducing the time and cost of drug development.
AI-Driven Optimization and Analysis
The integration of artificial intelligence and machine learning will significantly enhance the capabilities of scientific digital twins. AI algorithms can be used to analyze vast datasets, identify patterns and anomalies, and optimize the design and operation of complex systems. This will enable scientists to extract valuable insights from their digital twins that would be impossible to discern through manual analysis.
For example, AI could be used to identify optimal parameters for a chemical reaction within a digital twin of a laboratory experiment, leading to more efficient and effective processes. Similarly, AI could help predict the spread of a disease using a digital twin of a population, enabling proactive intervention strategies.
Ethical Considerations and Societal Impacts, Digital twins are enabling scientific innovation
The widespread adoption of scientific digital twins raises important ethical considerations. Concerns around data privacy, algorithmic bias, and the potential misuse of this powerful technology need to be addressed proactively. Ensuring transparency and accountability in the development and deployment of scientific digital twins is crucial to build public trust and prevent unintended consequences. For instance, the use of digital twins in healthcare raises questions about patient data privacy and the potential for discriminatory outcomes if algorithms are not carefully designed and monitored.
Robust regulatory frameworks and ethical guidelines are necessary to guide the responsible development and use of this technology.
Potential Areas for Revolutionizing Scientific Research
The next decade will likely witness transformative applications of digital twins across various scientific disciplines. Here are some key areas:
- Personalized Medicine: Creating detailed digital twins of individual patients to personalize treatment plans and predict disease progression.
- Climate Change Modeling: Developing highly accurate digital twins of the Earth’s climate system to better understand and predict climate change impacts.
- Drug Discovery and Development: Utilizing digital twins to simulate drug interactions and optimize drug design, reducing development time and costs.
- Materials Science: Designing and testing new materials virtually using digital twins, accelerating the development of novel materials with enhanced properties.
- Environmental Monitoring and Management: Employing digital twins to model and manage ecosystems, predict environmental disasters, and optimize resource management.
The power of digital twins to accelerate scientific innovation is undeniable. From optimizing drug development to predicting climate change, these virtual counterparts are proving invaluable tools. While challenges remain in terms of data management and accuracy, the potential benefits are immense. As technology continues to evolve, we can expect even more sophisticated and impactful applications of digital twins, ushering in a new era of scientific discovery and progress.
It’s an exciting time to be witnessing this technological revolution unfold, and I’m eager to see what groundbreaking advancements lie ahead.
Digital twins are revolutionizing fields like medicine and engineering, allowing for unprecedented simulations and analysis. It’s amazing how technology is advancing, even as geopolitical tensions rise, like with Antony Blinken’s recent visit to a violent hotspot close to home , highlighting the need for innovative solutions to complex problems. Ultimately, the power of digital twins to accelerate scientific discovery remains a beacon of hope in a world grappling with numerous challenges.
Digital twins are revolutionizing fields like medicine and engineering, allowing for unprecedented simulations and testing. It’s a stark contrast to the real-world consequences of actions like those of a Philadelphia man, who, according to this news report, was charged with postal crimes and possession of stolen mail-in ballots , highlighting the importance of secure systems. The potential of digital twins to improve processes and prevent such breaches is a key area of ongoing development.
Digital twins are revolutionizing scientific fields, allowing for unprecedented simulations and analysis. This precision extends to healthcare, where the application of AI is rapidly expanding; for example, check out this fascinating article on how ai offers an intriguing new way to diagnose mental health conditions. The insights gained from such AI-driven diagnostics can then be fed back into refining digital twin models, further accelerating innovation in personalized medicine and beyond.