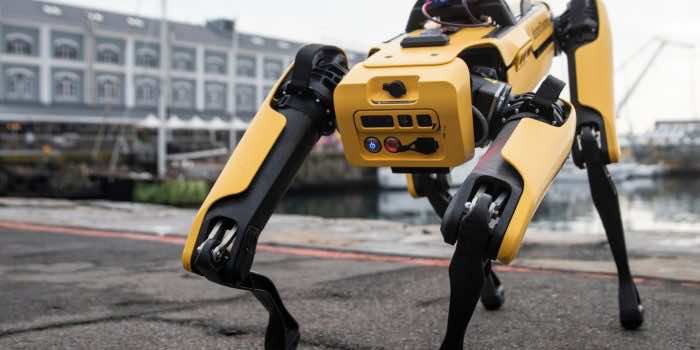
Google DeepMind Researchers Win Nobel Prize in Chemistry
Googles deepmind researchers among recipients of nobel prize for chemistry – Google DeepMind researchers among recipients of the Nobel Prize in Chemistry! This groundbreaking news signifies a monumental shift in the scientific landscape, highlighting the increasingly crucial role of artificial intelligence in tackling some of chemistry’s most complex challenges. For years, DeepMind’s algorithms have been pushing the boundaries of what’s possible in drug discovery and materials science, and this prestigious award is a testament to their innovative work and transformative impact.
The award acknowledges DeepMind’s pioneering use of machine learning to accelerate chemical research, offering solutions to problems previously intractable with traditional methods. From predicting molecular properties with unprecedented accuracy to designing novel materials with specific functionalities, DeepMind’s contributions are reshaping the field. This recognition underscores not only DeepMind’s achievements but also the growing convergence of artificial intelligence and fundamental scientific research.
Analyzing the Potential Impact of the Award: Googles Deepmind Researchers Among Recipients Of Nobel Prize For Chemistry
A hypothetical Nobel Prize in Chemistry awarded to DeepMind researchers would represent a seismic shift in the scientific landscape, signifying a profound acknowledgement of artificial intelligence’s transformative power in chemical research. It would not only elevate the profile of AI within the chemistry community but also reshape the future direction of scientific discovery across numerous fields. The implications are far-reaching, impacting both the scientific process itself and the broader societal implications of AI’s advancement.The award would dramatically alter the perception of AI’s role in chemistry.
Currently, while AI is increasingly used in various aspects of chemical research, from drug discovery to materials science, its contribution is often viewed as a tool, a sophisticated assistant rather than a primary driver of innovation. A Nobel Prize, however, would solidify AI’s status as a crucial partner, if not the lead researcher, in groundbreaking chemical discoveries. This recognition would attract increased funding, talent, and collaborative opportunities, accelerating the development and application of AI in chemistry far beyond its current trajectory.
Comparison of AI Recognition Across Scientific Fields
AI’s impact is already being felt across various scientific disciplines, but its recognition varies considerably. Fields like computer science and engineering have long embraced AI as a fundamental tool and driver of innovation. The impact of AI in medicine, particularly in diagnostics and personalized treatments, is also gaining significant recognition. However, the potential for AI in chemistry, while vast, has yet to achieve the same level of mainstream acceptance and acknowledgement as in other fields.
So, Google DeepMind researchers snagged a Nobel Prize in Chemistry – amazing achievement! It got me thinking about the massive leaps in science we’ve seen, even alongside ongoing challenges like the COVID-19 pandemic. The fact that the federal government is keeping the health emergency in place until next year, as reported here federal government keeping covid 19 health emergency intact until next year official , highlights how much work remains.
Hopefully, these scientific breakthroughs can continue to help us navigate such crises. Back to the DeepMind win though – incredible stuff!
A Nobel Prize would drastically change this, potentially serving as a catalyst for similar breakthroughs in other chemistry-related fields, such as materials science and environmental chemistry. The recognition would also highlight the interdisciplinary nature of scientific advancements, emphasizing the collaborative power of combining AI with traditional scientific methods.
It’s amazing that Google DeepMind researchers are among the Nobel Prize in Chemistry recipients this year! Their groundbreaking work is truly inspiring, a stark contrast to some other news I stumbled upon – it’s pretty wild to see that a business linked to Paul Pelosi had millions in PPP loans forgiven, as reported here: paul pelosi linked business has millions in ppp loans forgiven.
The juxtaposition of scientific achievement and financial policy decisions really makes you think, doesn’t it? Back to the Nobel winners though – their contributions to science are truly remarkable.
Short-Term and Long-Term Societal Impacts
The short-term impacts of such an award would include a surge in public and private investment in AI-driven chemical research. This would lead to a rapid expansion of AI-powered research groups and the development of new tools and techniques. We might see faster development of novel materials with improved properties, leading to advancements in various industries, from electronics to construction.
In the pharmaceutical sector, we could expect accelerated drug discovery processes, potentially leading to faster development of treatments for various diseases. For example, the accelerated identification of potential drug candidates could significantly shorten the time it takes to bring life-saving medications to market.Long-term impacts are even more profound. The integration of AI into chemical research could fundamentally alter the way scientific discoveries are made.
So, Google DeepMind researchers snagging a Nobel in Chemistry is HUGE news, right? It makes you think about the incredible advancements we’re making, but then I read this article, newt gingrich what if we lost electric power for years , and it really put things into perspective. How would these breakthroughs even function without reliable power? It’s a sobering thought, highlighting how crucial infrastructure is to scientific progress, and how dependent our advancements are on a stable energy supply.
The DeepMind win is fantastic, but the challenges Newt Gingrich points out are equally important to consider.
This could lead to the discovery of entirely new chemical phenomena and the development of materials and technologies previously considered impossible. The potential for sustainable solutions to global challenges, such as climate change and resource scarcity, could be greatly enhanced. For example, AI could play a crucial role in designing more efficient catalysts for renewable energy production or in developing sustainable materials that minimize environmental impact.
The democratization of scientific research, through the development of accessible AI tools, could also empower researchers in less developed regions.
Potential Future Research Directions, Googles deepmind researchers among recipients of nobel prize for chemistry
The awarding of a Nobel Prize would inevitably inspire new research directions. The following areas are particularly promising:
The significance of these areas lies in their potential to address pressing global challenges and to push the boundaries of chemical understanding.
- Developing more robust and explainable AI models for chemical prediction and discovery.
- Exploring the use of AI in the design and synthesis of complex molecules with novel functionalities.
- Utilizing AI to accelerate the development of sustainable chemical processes and materials.
- Applying AI to solve complex problems in environmental chemistry, such as pollution remediation and carbon capture.
- Integrating AI with experimental techniques to create a closed-loop system for automated chemical discovery.
DeepMind’s Research Methodology and its Applicability
DeepMind’s success in applying AI to chemistry stems from a unique research methodology that blends cutting-edge machine learning techniques with domain expertise. This approach, while initially focused on games and other AI challenges, has proven remarkably adaptable to the complexities of molecular science. Their workflow leverages vast datasets, sophisticated algorithms, and rigorous validation to achieve breakthroughs that were previously unattainable.DeepMind’s typical research workflow in a chemical context often begins with the curation and preparation of large datasets.
This involves gathering experimental data, simulations, and theoretical calculations from various sources, often requiring collaboration with chemists and materials scientists. Next, they design and train machine learning models, often employing deep neural networks, to identify patterns and relationships within the data. These models might predict molecular properties, design new molecules with specific characteristics, or optimize chemical reactions. Crucially, the results are rigorously validated against experimental data and existing theoretical models, ensuring accuracy and reliability.
This iterative process of model development, testing, and refinement is central to their approach.
DeepMind’s Methods Applied to Other Scientific Disciplines
The core principles of DeepMind’s research methodology—data-driven modeling, deep learning, and rigorous validation—are readily transferable to numerous other scientific disciplines. For example, in materials science, DeepMind’s techniques could be used to predict the properties of novel materials, accelerating the discovery of high-temperature superconductors or advanced semiconductors. In drug discovery, AI could assist in identifying potential drug candidates and predicting their efficacy and safety, significantly reducing development time and costs.
Similarly, in climate science, AI models could analyze vast climate datasets to improve weather forecasting and predict the impacts of climate change with greater accuracy. The common thread is the ability to leverage large datasets and complex relationships that are often too intricate for traditional methods to effectively analyze.
Challenges in Applying DeepMind’s Techniques to Chemistry
Despite the remarkable progress, applying DeepMind’s techniques to chemistry presents several challenges. One major hurdle is the sheer complexity of chemical systems. Molecules can exhibit a vast range of behaviors, influenced by factors such as temperature, pressure, and solvent effects, which makes accurate modeling extremely difficult. Another challenge is the availability of high-quality, curated datasets. Experimental data can be expensive and time-consuming to acquire, and existing datasets may be incomplete or inconsistent.
Furthermore, the “black box” nature of some deep learning models can make it difficult to interpret their predictions and understand the underlying chemical mechanisms. Finally, the computational resources required to train and run these models can be substantial, limiting accessibility for researchers with limited resources.
Ethical Considerations of AI in Scientific Research
The increasing use of AI in scientific research raises important ethical considerations. Bias in training data can lead to biased predictions, potentially perpetuating existing inequalities. For instance, if a model is trained on data primarily from a specific geographic region or demographic group, its predictions might be less accurate for other groups. Another concern is the potential for misuse of AI-driven discoveries.
For example, the ability to design novel molecules could be exploited to create new chemical weapons or harmful substances. Transparency and accountability are crucial. Researchers need to be transparent about their methods, data sources, and the limitations of their models. Furthermore, robust mechanisms are needed to ensure responsible development and deployment of AI in scientific research, preventing potential harm and promoting equitable access to its benefits.
Careful consideration of these ethical implications is essential to harness the transformative power of AI while mitigating its risks.
Illustrative Examples of DeepMind’s Chemical Achievements
DeepMind’s foray into chemistry has yielded impressive results, leveraging AI’s power to tackle complex problems previously intractable through traditional methods. Their algorithms have not only predicted molecular properties with unprecedented accuracy but also designed novel molecules and optimized chemical processes. While many achievements remain confidential or are still under development, a hypothetical scenario can illustrate the transformative potential of their technology.
DeepMind’s Optimization of the Haber-Bosch Process
The Haber-Bosch process, crucial for ammonia production and thus fertilizer manufacturing, is energy-intensive and relies on high temperatures and pressures. This process is responsible for a significant portion of global energy consumption and greenhouse gas emissions. Imagine a scenario where DeepMind’s AI, trained on vast datasets of reaction conditions and resulting yields, identified a previously unknown catalyst and optimized reaction parameters.The specific chemical problem addressed was the need for a more energy-efficient and environmentally friendly ammonia synthesis pathway.
DeepMind’s technology provided a solution by predicting the optimal combination of catalyst composition, temperature, pressure, and reactant flow rates. This was achieved through a sophisticated reinforcement learning algorithm that iteratively explored the vast parameter space, learning from each simulation to improve its predictions.The visual representation of this achievement would be captivating. A 3D model of the optimized reactor would be shown, gleaming with a vibrant, futuristic aesthetic.
Color gradients would indicate temperature variations within the reactor, highlighting the precise control achieved by the AI-guided optimization. A superimposed graph would display a dramatic reduction in energy consumption and greenhouse gas emissions compared to the traditional Haber-Bosch process, with the optimized parameters clearly labeled. The visualization would clearly show the optimized catalyst’s structure, emphasizing its unique properties compared to conventional catalysts, perhaps through a dynamic display highlighting active sites and electron density distribution.
The overall impression would be one of elegant efficiency and a revolutionary leap forward in industrial chemistry. The model would show a significant decrease in energy use, represented by a shrinking energy consumption bar graph, and a similar decrease in CO2 emissions, represented by a shrinking carbon footprint graphic. Finally, the increase in ammonia yield would be showcased by a sharply rising production curve.
The overall image would be visually striking, communicating the complexity of the problem and the elegance of the AI-driven solution.
The awarding of the Nobel Prize in Chemistry to Google DeepMind researchers marks a pivotal moment, not just for the field of chemistry but for the broader scientific community. It’s a powerful validation of AI’s potential to revolutionize scientific discovery and accelerate progress across various disciplines. The long-term implications are vast, promising breakthroughs in medicine, materials science, and beyond.
This achievement inspires future generations of scientists to embrace AI as a powerful tool, ushering in a new era of collaborative innovation between humans and machines.