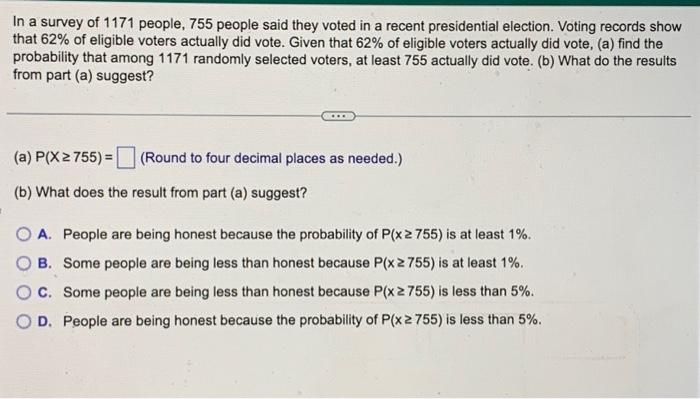
Polls Get Elections Wrong, So Use Google, Says Seth Stephens-Davidowitz
Polls get elections wrong so use google says seth stephens davidowitz – Polls Get Elections Wrong, So Use Google, Says Seth Stephens-Davidowitz – that’s a bold claim, right? And honestly, it got me thinking. We all know those pre-election polls, often wildly off the mark. Seth Stephens-Davidowitz, however, argues that we’ve been looking in the wrong place for predictive power. He suggests that Google search data offers a far more accurate picture of the electorate’s true intentions.
This isn’t just about some academic theory; it’s about understanding why polls consistently fail and what we can do to improve our election forecasting.
Stephens-Davidowitz supports his argument with compelling examples, showing how certain search trends accurately reflected election outcomes even when traditional polls missed the mark. He delves into the inherent biases and limitations of both traditional polling and Google search data, offering a nuanced perspective that acknowledges the strengths and weaknesses of each method. By comparing and contrasting these approaches, he challenges us to rethink how we predict election results and consider alternative data sources for a more comprehensive understanding of voter sentiment.
Seth Stephens-Davidowitz’s Argument
Seth Stephens-Davidowitz, a data scientist and author, argues that traditional polling methods consistently fail to accurately predict election outcomes. His critique centers on the limitations of relying solely on self-reported data and the inherent biases present in survey methodologies. He contends that these biases, coupled with a misunderstanding of how voters actually behave, lead to significant discrepancies between poll predictions and the final results.Stephens-Davidowitz supports his claim by examining several recent elections, highlighting instances where polls significantly misjudged the final outcome.
He points to the underestimation of support for Brexit in the 2016 referendum and Donald Trump’s victory in the 2016 US Presidential election as prime examples. He argues that these miscalculations stemmed from a failure to account for the “shy Trump voter” phenomenon – individuals who, for various social or political reasons, were hesitant to admit their support for Trump to pollsters.
He also emphasizes the limitations of random sampling, arguing that it doesn’t effectively capture the nuances of voter behavior in a complex and increasingly polarized society.
Comparison of Stephens-Davidowitz’s Perspective with Traditional Polling Methodologies
Traditional polling methodologies generally rely on random sampling techniques to create a representative sample of the population. They then use statistical methods to project the likely outcome of an election based on the responses gathered. Stephens-Davidowitz, however, argues that this approach overlooks crucial factors, such as the social desirability bias – the tendency for individuals to answer questions in a way they believe is socially acceptable, even if it’s not entirely truthful.
Seth Stephens-Davidowitz’s point about polls failing and Google’s data being more reliable really makes you think. It highlights how easily manipulated public opinion can be, which is further underscored by the revelations in this article: elon musk exposes twitters censorship of former president donald trump. The suppression of information clearly distorts the picture, making accurate polling even harder, and reinforcing the need for alternative data sources like Google trends to understand true public sentiment.
He advocates for incorporating alternative data sources, such as social media activity and search engine data, to gain a more comprehensive understanding of voter sentiment. This contrasts sharply with traditional methods which primarily rely on phone or in-person surveys.
Discrepancies Between Poll Predictions and Actual Election Results
The following table illustrates some notable discrepancies between poll predictions and actual election results in recent elections. Note that discrepancies can stem from various factors, not solely from limitations in polling methodologies. These are simply examples cited by Stephens-Davidowitz and other election analysts to highlight the challenges of accurate prediction.
Election Year | Poll Prediction | Actual Result | Discrepancy |
---|---|---|---|
2016 US Presidential Election | Hillary Clinton predicted to win comfortably | Donald Trump won | Significant underestimation of Trump support |
2016 UK EU Referendum | Remain predicted to win | Leave won | Significant underestimation of Leave support |
2020 US Presidential Election | Biden predicted to win, but margin of victory varied widely across polls | Biden won | Some polls overestimated Biden’s margin of victory; others underestimated it. |
The Role of Google Search Data
Google search data offers a fascinating, albeit imperfect, lens through which to view public opinion and, potentially, predict election outcomes. Unlike traditional polling, which relies on a sample of the population, Google search data provides a massive, real-time snapshot of what millions of people are actively searching for. This provides a unique opportunity to gauge public sentiment, albeit with important caveats.Google search data can be used to predict election outcomes by analyzing trends in search queries related to candidates, political issues, and election-related terms.
Increased searches for a specific candidate’s name, particularly in comparison to their opponents, might suggest growing public interest and potential support. Similarly, an upswing in searches for terms like “how to register to vote” or “polling place locations” in a specific region could indicate heightened voter engagement. Analyzing the geographical distribution of these searches can further refine predictions, revealing areas of strong support or opposition for particular candidates.
Specific Search Queries as Indicators of Voting Preferences
Searches related to candidates’ names, policy positions, and even negative news stories can serve as valuable indicators. For example, a sudden spike in searches for “[Candidate A] scandals” coupled with a simultaneous drop in searches for “[Candidate A] policy” could suggest a negative shift in public perception. Conversely, a surge in searches for “[Candidate B] platform” alongside positive news coverage could indicate growing support.
The relative frequency of searches comparing candidates (“[Candidate A] vs [Candidate B]”) can also provide insights into the public’s focus and potential decision-making processes. The geographical distribution of these searches allows for a granular analysis of regional preferences. For instance, a higher concentration of searches for “early voting locations” in a particular county might suggest a higher anticipated voter turnout in that area.
Seth Stephens-Davidowitz’s point about polls failing to predict elections accurately, and the need to leverage Google data instead, really hit home. It makes you wonder about the underlying motivations, like the cynical political maneuvering John Delaney highlights in his recent piece, john delaney suggests some dems are cheering on a recession to hurt trump , which further clouds accurate polling.
Ultimately, understanding voter sentiment requires more than just traditional polls, especially given such potentially manipulative factors.
Limitations and Biases in Using Google Search Data
While powerful, Google search data is not without limitations. One major concern is the potential for bias. The data reflects the searches conducted by those who use Google, a population that may not accurately represent the overall electorate. Furthermore, search behavior can be influenced by various factors beyond political preference, such as media coverage, social media trends, and even targeted advertising campaigns.
Seth Stephens-Davidowitz’s point about polls failing and Google’s data being more reliable really hit home for me. It makes you wonder how accurate any polling really is, especially considering what happened after Biden’s speech; check out this article about how it’s become gop voters virtually impossible to poll after Bidens maga republicans speech top pollster , making the whole polling process even more questionable.
So, yeah, maybe we should all be looking at Google Trends instead!
A surge in searches for a candidate might not reflect genuine support but rather the effect of a well-orchestrated publicity stunt. Another limitation is the inherent difficulty in directly translating search data into voting intentions. A high volume of searches for a candidate doesn’t automatically translate to votes cast for that candidate. People might search for information out of curiosity, concern, or even opposition.
Hypothetical Study Comparing Google Search Data and Traditional Polling Data
A hypothetical study could compare the accuracy of election predictions based on Google search data against those derived from traditional polling data. The study would involve selecting a set of past elections and collecting both Google search data (e.g., daily search volume for candidate names, policy-related terms) and corresponding polling data for the same period. A predictive model would be developed for each data source, and the accuracy of each model would be evaluated using metrics such as mean absolute error and root mean squared error in predicting the final election results.
The study could then compare the performance of the two models, potentially identifying strengths and weaknesses of each approach and exploring whether combining both data sources could lead to improved predictive accuracy. For example, the study could analyze the 2016 US Presidential election, comparing Google Trends data on searches for “Hillary Clinton” and “Donald Trump” with polling data from reputable sources like Gallup or Pew Research Center to assess the predictive accuracy of each method.
This comparison would allow for a quantitative evaluation of the relative merits of each approach.
Exploring Alternative Predictive Methods
Polling data, while traditionally a cornerstone of election forecasting, has shown its limitations in recent years. The increasing influence of social media and the complexities of modern political landscapes necessitate exploring alternative methods to improve the accuracy of election predictions. This exploration delves into data sources beyond polls and Google searches, examining their potential and limitations in forecasting election outcomes.
Beyond traditional polls and Google search data, several alternative data sources offer promising avenues for election forecasting. These sources, while not without their challenges, provide unique insights into voter sentiment and behavior, potentially offering a more comprehensive picture than traditional methods alone. The integration of these alternative data sources with established techniques can lead to more robust and accurate prediction models.
Social Media Sentiment Analysis
Social media platforms like Twitter and Facebook offer a vast repository of real-time public opinion. Sentiment analysis, a technique used to determine the emotional tone expressed in text, can be applied to social media posts to gauge public sentiment towards candidates and political issues. For example, analyzing the sentiment expressed in tweets mentioning specific candidates during a campaign can reveal shifts in public support.
This method can provide a rapid, dynamic picture of public opinion, offering insights unavailable through slower, more traditional polling methods. However, it’s crucial to account for biases in social media representation – not everyone uses social media, and the demographics of social media users may not perfectly reflect the general population. The use of sophisticated natural language processing (NLP) techniques is crucial to accurately interpret the nuances of language and filter out irrelevant information, noise, and bots.
A successful model would incorporate demographic weighting to compensate for the inherent biases of social media platforms. Furthermore, the sheer volume of data necessitates efficient processing and filtering algorithms to extract meaningful insights.
Economic Indicators and Election Outcomes
Economic indicators, such as unemployment rates, GDP growth, and consumer confidence, have historically shown a correlation with election outcomes. Periods of economic prosperity often favor incumbents, while economic downturns can lead to shifts in voter preferences. For instance, the high unemployment rate during the Great Depression significantly impacted the 1932 presidential election, leading to Franklin D. Roosevelt’s landslide victory.
However, the relationship between economic indicators and election outcomes is complex and not always straightforward. Other factors, such as social issues and candidate personalities, also play significant roles. A robust predictive model would incorporate economic data alongside other factors to provide a more holistic perspective. Sophisticated statistical models, such as regression analysis, can be used to quantify the influence of economic indicators on election outcomes, allowing for a more nuanced understanding of their predictive power.
Creating an Election Prediction Model Using Financial Market Data
Financial markets often reflect broader economic sentiment and anticipatory behavior. Changes in stock prices, volatility indices (like the VIX), and the performance of specific sectors can serve as indicators of public confidence and expectations. For example, a surge in market volatility leading up to an election could signal uncertainty and potential shifts in voter sentiment. A prediction model using this data would involve: (1) collecting relevant financial data, such as daily stock market indices and volatility measures, for a period leading up to previous elections; (2) correlating this data with the actual election outcomes; (3) developing a statistical model (e.g., time-series analysis or machine learning algorithms) to identify patterns and relationships; and (4) using the model to forecast future election outcomes based on current financial market conditions.
This approach necessitates careful consideration of confounding factors and potential biases in financial markets.
Comparison of Election Prediction Methods, Polls get elections wrong so use google says seth stephens davidowitz
Method | Advantages | Disadvantages | Accuracy |
---|---|---|---|
Traditional Polling | Established methodology, relatively straightforward | Sampling bias, response rates, question wording effects | Variable, often within a margin of error |
Google Search Data | Large scale, real-time data, reveals implicit preferences | Interpretation challenges, correlation vs. causation, privacy concerns | Improving, but still subject to limitations |
Social Media Sentiment Analysis | Real-time, dynamic data, potentially reveals nuanced opinions | Bias, noise, difficulty in interpreting sentiment accurately | Developing, accuracy depends on methodology and data quality |
Economic Indicators | Established correlation with election outcomes, readily available data | Complex relationships, other factors influence elections | Moderately predictive, but not a sole determinant |
Financial Market Data | Reflects broader economic sentiment and anticipatory behavior | Vulnerable to market manipulation, complex relationships | Emerging method, accuracy needs further evaluation |
Implications and Future Directions: Polls Get Elections Wrong So Use Google Says Seth Stephens Davidowitz
Stephens-Davidowitz’s work, while groundbreaking, presents a significant challenge to traditional election polling methods. His findings highlight the limitations of relying solely on surveys and suggest a need for a more nuanced and multi-faceted approach to political forecasting. The implications extend beyond simply improving accuracy; they also raise important ethical considerations and demand innovative solutions in data collection and analysis.The core implication is a shift away from the exclusive reliance on traditional polling methodologies.
Stephens-Davidowitz’s research demonstrates that seemingly disparate data sources, like Google search trends, can offer valuable insights into voter sentiment and behavior that traditional polls might miss. This necessitates a reevaluation of how we gather and interpret data for political forecasting, moving towards a more holistic approach that incorporates diverse data streams.
Improving Poll Accuracy
Addressing the limitations of traditional polling requires a multi-pronged strategy. First, integrating alternative data sources, such as Google search data, social media sentiment analysis, and even credit card transaction patterns (to infer economic anxieties), can enrich the predictive models. Second, improving sampling techniques to better represent the diversity of the electorate is crucial. Traditional polls have often struggled with accurately representing marginalized groups, leading to skewed results.
Finally, enhancing the statistical models used to analyze poll data is necessary to account for the complexities of voter behavior and the limitations of any single data source. For example, weighting survey responses based on demographic data correlated with Google search trends could help mitigate biases.
Ethical Considerations of Alternative Data
The use of alternative data sources for political prediction raises significant ethical considerations. Privacy is paramount. Using Google search data, for example, requires careful anonymization and adherence to strict data protection regulations. Transparency is equally vital; the methodologies used to analyze alternative data should be clearly documented and made available for scrutiny. Furthermore, there’s a risk of bias in the algorithms used to process this data.
If algorithms are not carefully designed and tested, they may inadvertently amplify existing societal biases, leading to inaccurate and potentially harmful predictions. The potential for misuse, such as targeted disinformation campaigns based on insights gleaned from alternative data, must also be addressed proactively.
Enhancing Data Collection and Analysis
The accuracy of future election predictions can be significantly enhanced through improved data collection and analysis techniques. This includes developing more sophisticated algorithms capable of integrating diverse data streams, from traditional polls to social media activity and economic indicators. Machine learning techniques, for instance, can be employed to identify patterns and correlations that might be missed by human analysts.
Furthermore, investing in research on the psychological and sociological factors influencing voting behavior will refine the predictive models. For example, understanding the influence of misinformation campaigns on search trends can help refine the interpretation of Google search data. Real-time data analysis, enabling the tracking and analysis of evolving public sentiment during the campaign, can also improve the accuracy of predictions.
The 2016 US Presidential election serves as a stark reminder of the need for such advancements; a more sophisticated approach to data analysis might have better predicted the outcome.
So, are polls completely useless? Not necessarily. But Stephens-Davidowitz’s work highlights a crucial need for innovation in political forecasting. Relying solely on traditional polls seems increasingly inadequate, given their consistent inaccuracies. The use of alternative data sources, like Google searches and social media sentiment, offers a promising avenue for improvement.
However, we must also acknowledge the ethical implications and potential biases involved in utilizing such data. The future of accurate election prediction likely lies in a multi-faceted approach, combining traditional methods with innovative data analysis techniques to paint a more complete picture of voter preferences. It’s a fascinating challenge, and one that warrants ongoing exploration.