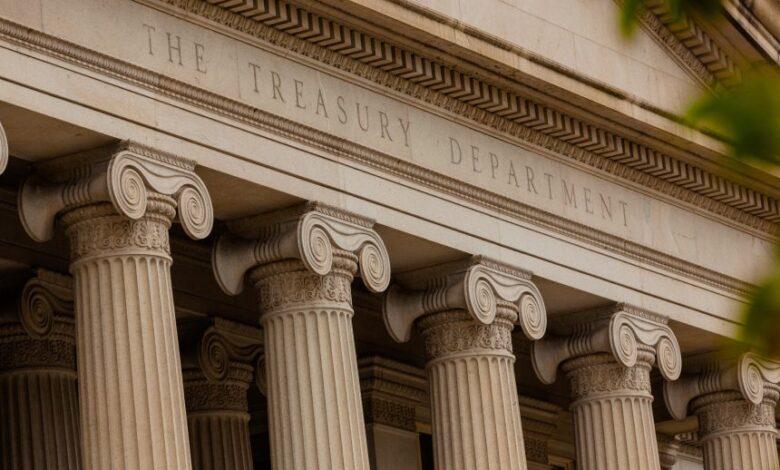
Regulators Prioritize Real AI Risks Good News?
Regulators are focusing on real ai risks over theoretical ones good – Regulators are focusing on real AI risks over theoretical ones – good news, or a potential oversight? The rapid advancement of artificial intelligence has brought with it a flurry of both exciting possibilities and serious concerns. While sci-fi scenarios of rogue AI dominate headlines, the reality is that AI is already impacting our lives in tangible, often problematic, ways.
This shift in regulatory focus towards demonstrable harm is a crucial development, demanding a careful examination of its implications.
This post dives into the complexities of this evolving landscape. We’ll explore the stark differences between “real” and “theoretical” AI risks, examining examples of each and the reasons behind the regulatory shift. We’ll also consider the potential downsides of prioritizing immediate threats over long-term possibilities, and speculate on the future of AI regulation in a world where the lines between reality and theory continue to blur.
Defining “Real” vs. “Theoretical” AI Risks
The line between theoretical and real AI risks is blurring rapidly as AI systems become more sophisticated and integrated into our lives. While hypothetical scenarios involving rogue AI dominate popular culture, a significant number of genuine risks are already manifesting themselves in our society, demanding immediate attention and mitigation strategies. This discussion will clarify the distinction between these two categories, focusing on concrete examples of each.
Real-World AI Risks Currently Impacting Society
Several tangible risks associated with AI are currently impacting various aspects of our society. These aren’t speculative dangers; they are demonstrable problems demanding immediate solutions.
We can categorize these real-world risks into three distinct areas: bias and discrimination, privacy violations, and job displacement.
- Bias and Discrimination: AI systems trained on biased data perpetuate and amplify existing societal inequalities. For example, facial recognition systems have been shown to be less accurate in identifying individuals with darker skin tones, leading to misidentification and potential discriminatory outcomes in law enforcement and security applications. Similarly, AI-powered loan applications may unfairly discriminate against certain demographic groups based on historical biases present in the training data.
- Privacy Violations: The increasing use of AI in surveillance technologies and data collection raises serious privacy concerns. Facial recognition in public spaces, coupled with data aggregation from various sources, can enable extensive tracking and profiling of individuals without their informed consent. This raises ethical and legal challenges regarding the balance between security and individual liberties.
- Job Displacement: Automation driven by AI is already impacting the job market, particularly in sectors reliant on repetitive tasks. While some argue that AI will create new jobs, the transition can be disruptive, leading to unemployment and economic inequality if not managed effectively. The trucking industry, for example, faces significant automation, potentially displacing millions of drivers.
Theoretical AI Risks Lacking Substantial Real-World Evidence
Conversely, several often-discussed AI risks remain largely theoretical, lacking sufficient real-world evidence to warrant immediate panic, although vigilance is still necessary.
Three examples of such theoretical risks include the development of superintelligent AI, the unintended consequences of autonomous weapons systems, and the potential for AI-driven societal collapse.
- Superintelligent AI: The hypothetical emergence of an AI surpassing human intelligence in all aspects remains a topic of much debate. While theoretically possible, there’s currently no clear pathway or evidence suggesting its imminent development. The complexity and unpredictable nature of achieving such a level of intelligence make this a long-term concern, not an immediate threat.
- Unintended Consequences of Autonomous Weapons Systems: The development of lethal autonomous weapons (LAWs) raises concerns about unintended escalation and lack of human control in warfare. While the technology is advancing, widespread deployment and demonstrably catastrophic consequences remain hypothetical at this stage. International regulations and ethical discussions are actively attempting to prevent such scenarios.
- AI-Driven Societal Collapse: Some scenarios envision AI leading to a societal collapse due to factors such as widespread job displacement, economic disruption, or the misuse of AI for malicious purposes. While these are valid concerns, they rely on a complex interplay of factors and currently lack concrete evidence of imminent collapse. Instead, they highlight the need for proactive measures to mitigate potential risks.
Comparison of Real and Theoretical AI Risks
Risk Type | Description | Evidence of Impact | Potential Mitigation Strategies |
---|---|---|---|
Bias and Discrimination in AI | AI systems trained on biased data perpetuate and amplify existing societal inequalities. | Numerous documented cases of biased outcomes in facial recognition, loan applications, and hiring processes. | Developing fairer algorithms, auditing datasets for bias, implementing diversity and inclusion measures in AI development teams. |
Privacy Violations from AI Surveillance | Increased use of AI in surveillance technologies and data collection leads to extensive tracking and profiling. | Widespread use of facial recognition, data tracking, and profiling through various digital platforms. Growing concerns about data breaches and misuse. | Strengthening privacy regulations, implementing data anonymization techniques, promoting transparency and user control over data. |
Job Displacement due to AI Automation | Automation driven by AI displaces workers in various sectors. | Observed job losses in manufacturing, transportation, and customer service due to automation. | Investing in retraining and upskilling programs, exploring policies like universal basic income, promoting human-AI collaboration. |
Superintelligent AI | Hypothetical emergence of an AI surpassing human intelligence in all aspects. | No current evidence of imminent development. | Research on AI safety and alignment, fostering international cooperation on AI governance. |
Unintended Consequences of Autonomous Weapons Systems | Concerns about unintended escalation and lack of human control in warfare. | Technological advancements in LAWS, but no widespread deployment or catastrophic consequences yet observed. | International regulations and treaties to limit or ban the development and deployment of LAWS. |
AI-Driven Societal Collapse | Hypothetical scenario where AI leads to societal collapse due to various factors. | No current evidence of imminent collapse. | Proactive risk management, robust societal safety nets, and responsible AI development practices. |
Regulator Focus and Priorities: Regulators Are Focusing On Real Ai Risks Over Theoretical Ones Good
Regulators worldwide are increasingly focusing their attention on the real-world risks posed by artificial intelligence, prioritizing immediate threats over hypothetical future scenarios. This shift reflects a growing understanding of the tangible harms AI can inflict and the need for practical, effective regulatory frameworks. The focus is on mitigating present dangers, rather than speculating on potential long-term, albeit potentially catastrophic, outcomes.This prioritization is driven by a confluence of factors, including the urgency of addressing existing harms, the feasibility of implementing regulations targeting these harms, and the pressure from public concern and economic considerations.
It’s refreshing to see regulators prioritizing tangible AI risks – like job displacement and bias – instead of getting bogged down in hypothetical doomsday scenarios. This focus on the real world is crucial, much like understanding the immediate geopolitical realities, as illustrated in the Israel-Iran standoff in maps , requires a clear-eyed assessment of the situation. Focusing on practical AI dangers, rather than far-fetched ones, is a more effective and responsible approach.
By focusing on concrete risks, regulators can achieve quicker results and build public trust in their ability to manage the development and deployment of AI.
It’s refreshing to see regulators prioritizing tangible AI risks instead of getting bogged down in hypotheticals. This focus on real-world impact reminds me of the recent article, a ports strike shows the stranglehold one union has on trade , highlighting how a single entity can severely disrupt global systems. Similarly, responsible AI regulation needs to address actual, present dangers, not just theoretical ones, to effectively mitigate potential harm.
Examples of Regulatory Actions Targeting Real AI Risks
Three notable examples illustrate the global focus on real AI risks. First, the European Union’s AI Act aims to classify AI systems based on their risk level, implementing stricter regulations for high-risk applications like those used in healthcare and law enforcement. This directly addresses risks such as algorithmic bias leading to unfair or discriminatory outcomes, and the potential for autonomous systems to cause physical harm.
Second, the UK’s approach focuses on promoting responsible innovation through a set of principles and guidance rather than prescriptive legislation. This targets risks related to data privacy, transparency, and accountability in AI systems, aiming to foster a culture of responsible AI development within the UK’s tech sector. Finally, China’s approach, while less focused on specific legislation like the EU, emphasizes the management of AI risks through a combination of ethical guidelines and industry self-regulation, focusing on issues like data security and the prevention of AI misuse in areas such as surveillance.
This reflects a concern with real-world applications of AI that could threaten national security or social stability.
Reasons for Prioritizing Real over Theoretical AI Risks
Regulators prioritize real AI risks due to several compelling reasons. Public safety is paramount; AI systems already deployed in critical infrastructure, transportation, and healthcare pose immediate risks of accidents, malfunctions, and harm to individuals. The economic impact of AI failures is also significant; faulty algorithms can lead to financial losses, damage to reputation, and disruptions to supply chains. Furthermore, regulating real risks is often more feasible.
Addressing tangible harms allows for the development of specific, measurable regulatory standards, whereas attempting to regulate hypothetical, far-future risks presents immense challenges in terms of prediction, measurement, and enforcement.
It’s refreshing to see regulators prioritizing tangible AI risks – like job displacement and algorithmic bias – instead of getting bogged down in hypothetical doomsday scenarios. Frankly, some of the political drama feels equally far-fetched; I mean, reading about claims like trump claims Biden’s leadership could drag America into World War III makes you appreciate the focus on concrete AI issues.
Getting a handle on real-world AI challenges seems far more urgent than worrying about robot uprisings right now.
Challenges in Addressing Theoretical AI Risks
The challenges in addressing theoretical AI risks are substantial.
- Uncertain Timeline and Probability: Predicting when and if theoretical risks, such as artificial general intelligence (AGI) surpassing human capabilities, will materialize is inherently difficult. The uncertainty makes it challenging to develop effective regulations.
- Defining and Measuring Risks: Many theoretical risks are poorly defined and lack quantifiable metrics. This makes it difficult to assess the severity of the risks and develop targeted regulatory measures.
- Rapid Technological Advancements: The rapid pace of AI development makes it difficult for regulations to keep up. What might seem like a distant theoretical risk today could become a reality much sooner than anticipated.
- International Coordination: Addressing global-scale theoretical risks requires significant international cooperation, which can be challenging to achieve due to differing regulatory priorities and approaches.
- Balancing Innovation and Safety: Overly restrictive regulations could stifle innovation, while insufficient regulation could lead to catastrophic outcomes. Finding the right balance is a major challenge.
Impact of Focusing on Real Risks
Regulators grappling with the burgeoning field of artificial intelligence face a crucial dilemma: how to effectively mitigate risks without stifling innovation. Focusing on demonstrable, real-world harms offers a pragmatic approach, but it also carries potential drawbacks. This section explores the potential benefits and downsides of prioritizing “real” AI risks over hypothetical future threats.Focusing on readily apparent risks allows for quicker, more targeted interventions.
This approach fosters a sense of urgency and allows regulators to address immediate societal harms, building public trust and demonstrating tangible results. By focusing on concrete problems, regulators can also develop more effective and nuanced regulations tailored to specific AI applications and their associated dangers. This targeted approach can also minimize the regulatory burden on businesses, allowing for innovation to continue while mitigating the most pressing dangers.
Potential Positive Consequences of Focusing on Demonstrated AI Risks, Regulators are focusing on real ai risks over theoretical ones good
Prioritizing demonstrable harms leads to faster regulatory action. For instance, biased algorithms used in loan applications resulting in discriminatory lending practices are a clear and present danger. Addressing this directly, through regulations requiring fairness audits or transparency measures, can provide immediate relief to affected communities. Similarly, focusing on the misuse of AI in autonomous vehicles, such as accidents caused by faulty software, allows for the rapid development of safety standards and testing protocols.
The success of these immediate interventions builds public confidence in regulatory bodies and strengthens the legitimacy of AI governance.
Potential Negative Consequences of Neglecting Potential Future Threats
While focusing on current risks is crucial, solely concentrating on immediate concerns can have serious long-term consequences. Neglecting potential future threats, such as the development of highly autonomous weapons systems or the potential for widespread job displacement due to advanced AI, risks allowing these dangers to escalate beyond manageable levels. A narrow focus can also lead to regulatory frameworks that are ill-equipped to adapt to the rapid pace of AI development.
This might involve creating regulations that become obsolete before they are even fully implemented, leading to a constant cycle of regulatory updates and potential loopholes. For example, current regulations might not adequately address the risks associated with advanced AI models capable of generating realistic deepfakes, which could be used for malicious purposes in the future.
Comparison of Regulatory Approaches: EU vs. US
The EU and US represent contrasting approaches to AI regulation. The EU, with its proposed AI Act, takes a more risk-based approach, categorizing AI systems based on their level of risk and imposing stricter requirements on high-risk systems. The US, on the other hand, has adopted a more fragmented approach, with various agencies focusing on specific aspects of AI governance.
This comparison highlights their differences:
Regulatory Body | Risk Focus | Regulatory Approach | Effectiveness (Preliminary Assessment) |
---|---|---|---|
European Union (EU) | Broad range of risks, including high-risk applications | Risk-based classification and regulation; comprehensive AI Act | Potential for high effectiveness due to comprehensive approach, but early stages of implementation. |
United States (US) | Focus on specific sectors and risks, often addressing harms after they occur. | Fragmented approach with various agencies addressing AI through existing laws and new initiatives. | Effectiveness varies depending on the agency and specific area, with potential for gaps in coverage. |
Future Regulatory Landscape
The future of AI regulation is a dynamic landscape shaped by the rapid pace of technological advancement and the evolving understanding of AI’s potential risks and benefits. While the current focus is rightly on mitigating immediate, real-world harms, the regulatory framework must also anticipate and prepare for the challenges posed by theoretical risks that may materialize in the future.
This requires a proactive and adaptable approach, capable of balancing innovation with safety and societal well-being.Predicting the future is inherently uncertain, but based on current trends, we can formulate plausible scenarios for the evolution of AI regulation.
Predictions for the Future of AI Regulation
The balance between addressing real and theoretical AI risks will likely shift over time. Initially, a strong emphasis on tangible harms, like algorithmic bias in loan applications or autonomous vehicle accidents, will remain crucial. However, as AI systems become more complex and autonomous, the focus will gradually broaden to encompass more abstract and potentially catastrophic risks. This will involve a greater emphasis on proactive risk assessment and mitigation strategies, rather than simply reacting to incidents after they occur.
We can expect a gradual shift from a primarily reactive to a more proactive regulatory model.Three specific predictions for the future of AI regulation are: (1) Increased international cooperation on AI standards and regulations, mirroring the collaborative efforts seen in other global challenges. This will be driven by the inherently transnational nature of AI development and deployment. (2) The emergence of specialized regulatory bodies focused solely on AI, reflecting the unique challenges and complexities of regulating this technology.
This is similar to how specialized agencies handle aviation or nuclear power. (3) A greater emphasis on explainability and transparency in AI systems, particularly those with significant societal impact. This will necessitate the development of new auditing and verification techniques to ensure that AI systems are functioning as intended and are not exhibiting unintended biases or behaviors. These predictions are supported by ongoing discussions at international forums like the OECD and the G7, as well as the increasing number of national-level AI strategies that prioritize safety and ethical considerations.
The Role of Technological Advancements
Technological advancements will profoundly shape the future regulatory landscape. The rapid evolution of AI capabilities, including advancements in machine learning, natural language processing, and robotics, will constantly challenge existing regulations. New technologies like generative AI, quantum computing, and neuromorphic computing will present unique regulatory challenges that require innovative approaches. For example, the ability of generative AI to create realistic fake videos and audio raises concerns about misinformation and deepfakes, requiring regulators to adapt their strategies to address these emerging threats.
The development of more powerful AI systems also raises questions about the potential for unintended consequences and the need for robust safety mechanisms. The development of auditing techniques to assess the safety and trustworthiness of these systems will become increasingly critical.
A Hypothetical Scenario: The Rise of Autonomous Warfare Systems
Consider a future scenario where highly autonomous weapons systems (AWS), capable of selecting and engaging targets without significant human intervention, become a reality. Currently, this is largely a theoretical risk, but rapid advancements in AI and robotics make it a plausible future challenge. The potential for accidental escalation, unintended consequences, and a loss of human control over lethal force poses significant ethical and security concerns.Regulators would likely respond in several ways.
First, there could be a complete ban on the development and deployment of fully autonomous weapons systems, similar to the existing international conventions on certain types of weapons. Second, international agreements and treaties could be established to regulate the development and use of less autonomous weapons systems, establishing strict criteria for human oversight and control. Third, significant investment in verification and validation techniques for AWS would be needed to ensure that these systems function reliably and safely.
This could involve the development of independent auditing mechanisms and international standards for testing and certification. The scenario highlights the need for proactive regulatory measures to address theoretical risks before they escalate into significant global security challenges.
The focus on real AI risks reflects a pragmatic approach to regulation – addressing immediate threats to public safety and economic stability. However, this focus shouldn’t come at the expense of long-term planning. Ignoring theoretical risks could leave us unprepared for unforeseen consequences. The future of AI regulation hinges on finding a balance: proactively mitigating present dangers while also developing frameworks to address the potential threats lurking on the horizon.
It’s a delicate balancing act, and one that will require constant vigilance and adaptation.