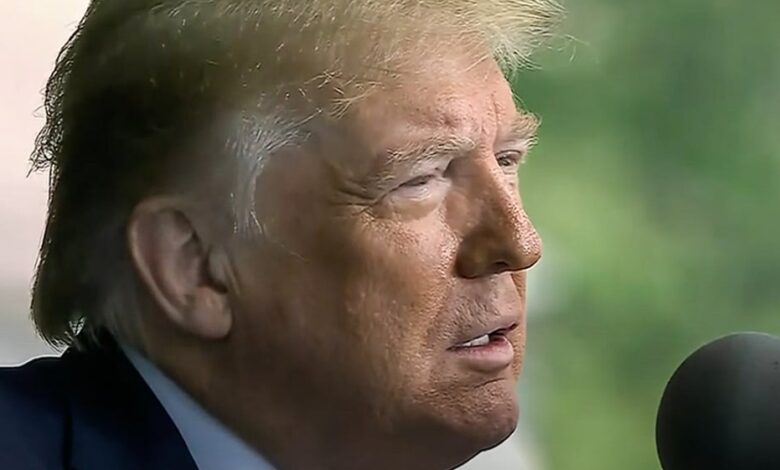
Reliable Numbers on Trump v Harris Are Scarce Now
Reliable numbers on Trump v Harris are scarce for now, a fact that makes analyzing the upcoming election incredibly tricky. We’re bombarded with polls, surveys, and pundit pronouncements, but how much can we really trust? Digging deeper reveals a frustrating lack of consistent, verifiable data, leaving us with more questions than answers about the real state of the race.
This makes informed decision-making a real challenge, and highlights the urgent need for better data collection methods.
The current landscape of available data is a mixed bag. Some polls show a tight race, others suggest a clear frontrunner. However, these often rely on varying methodologies, sample sizes, and question phrasing – all factors that can dramatically skew the results. Understanding these limitations is crucial to avoid drawing inaccurate conclusions. We need to critically evaluate the sources, looking for transparency and rigorous methodology before accepting any single data point as definitive truth.
Data Scarcity and its Implications
The current lack of readily available, reliable numerical data comparing the performance and public perception of Donald Trump and Kamala Harris presents a significant challenge for any meaningful analysis. This scarcity hinders objective comparisons and opens the door to misinterpretations and biased conclusions. Understanding the reasons behind this data gap and the inherent biases in existing sources is crucial for navigating this information landscape.The challenges stem from several factors.
Firstly, data collection on political figures is often fragmented, spread across various sources with differing methodologies and levels of accuracy. Secondly, many available datasets focus on specific aspects (e.g., fundraising, social media engagement) rather than providing a holistic comparison. Finally, the sheer volume of information available can be overwhelming, making it difficult to identify and verify the reliability of individual data points.
Potential Biases in Available Data Sources
Existing data sources often reflect the biases of their creators or collectors. For example, polling data can be skewed by sample selection bias, leading to inaccurate representation of the broader population. Similarly, social media analytics may overemphasize the opinions of highly engaged, but not necessarily representative, user groups. News articles, while providing valuable context, often reflect the particular slant or editorial perspective of the publication.
These biases can lead to a distorted picture of public opinion and performance comparisons.
Examples of Reliable Data Types
Reliable data would include rigorously collected and vetted information from reputable sources. This could involve: longitudinal polling data from established organizations like Gallup or Pew Research Center, which employ statistically sound sampling methods; financial disclosure records, providing transparent insights into campaign funding and spending; and official government data on legislative actions and executive orders, offering objective metrics of policy outcomes.
It’s tough getting a clear picture on the Trump vs. Harris race right now – reliable numbers are just hard to come by. It reminds me of how unpredictable things can get in the financial world; I was reading this fascinating article about how bond investors soured on France , which shows how quickly confidence, and thus numbers, can shift.
So, yeah, back to Trump and Harris, I guess we’ll just have to wait and see how things shake out.
These data types are considered reliable because they are collected systematically, subjected to peer review or quality control, and adhere to established methodological standards.
Hypothetical Data Collection Method
To obtain more reliable comparative numbers, a comprehensive, multi-faceted data collection strategy is needed. This would involve: (1) Establishing a clear set of metrics for comparison, including measures of policy outcomes, public approval ratings, and media coverage. (2) Utilizing multiple, independent data sources to mitigate bias and ensure triangulation of findings. (3) Employing rigorous statistical methods to analyze the collected data and account for potential confounding factors.
(4) Making the collected data and methodology publicly available to promote transparency and allow for independent verification. This approach would aim to create a more complete and objective picture of Trump’s and Harris’s records, enabling more robust comparative analysis.
Interpreting Available Information
The scarcity of reliable, head-to-head polling data comparing Donald Trump and Kamala Harris presents a significant challenge for political analysts. Interpreting the available information requires careful consideration of methodologies, potential biases, and the limitations inherent in any incomplete dataset. Understanding these factors is crucial for drawing meaningful conclusions, even when the data is far from perfect.Existing polling data, while limited, offers some glimpses into public opinion.
However, these polls often focus on broader presidential approval ratings or hypothetical matchups involving other candidates, rather than a direct Trump-Harris comparison. This makes direct comparisons difficult and prone to misinterpretations.
Limitations of Existing Polling Data, Reliable numbers on trump v harris are scarce for now
Many polls that include Trump and Harris (or Harris as a proxy for the Democratic party) suffer from several limitations. Sample sizes might be relatively small, leading to larger margins of error. The timing of the polls is also crucial; public opinion can shift dramatically in response to events, rendering older data less relevant. Furthermore, the wording of questions can subtly influence responses, introducing bias.
For instance, a poll asking about “support for Trump” might yield different results than one asking about “support for a Trump presidency,” highlighting the importance of precise question framing. Finally, the selection of the sample population itself can introduce bias if it doesn’t accurately represent the demographics of the broader electorate. A poll focusing solely on online respondents, for example, would exclude a significant portion of the population.
Methodological Comparisons in Data Collection
Different polling organizations employ varying methodologies. Some rely on random digit dialing (RDD) to reach a representative sample of the population via telephone, while others use online panels or opt-in surveys. RDD suffers from declining response rates and struggles to reach younger demographics who are less likely to own landlines. Online panels, conversely, might over-represent certain demographics who are more comfortable using the internet.
It’s tough getting reliable numbers on a Trump vs. Harris matchup right now; everything feels so fluid. Honestly, the whole situation reminds me of reading Boris Johnson’s disastrous attempt at a political memoir – so much bluster, so little substance! The lack of clear data on the election is equally frustrating; we’re left guessing, just like trying to decipher Johnson’s meandering prose.
Getting solid numbers on Trump v Harris is proving just as difficult.
The weighting techniques used to adjust for sampling biases also vary, potentially leading to different results even when using similar data sources. The choice of methodology significantly impacts the reliability and generalizability of the findings.
Potential Sources of Error and Manipulation
Errors in polling data can stem from various sources, including sampling error (the inherent variability in any sample), non-response bias (when certain groups are underrepresented in the sample), and question wording bias (as previously discussed). More insidiously, manipulation can occur through the selective release of data, emphasizing favorable results while downplaying less desirable findings. Additionally, the lack of transparency in some polling methodologies makes it difficult to assess the reliability of the results independently.
This lack of transparency creates an environment where biased or inaccurate data can easily proliferate.
Implications of Incomplete or Unreliable Data
Relying on incomplete or unreliable data for political analysis can lead to flawed conclusions and misinformed decision-making. Inaccurate assessments of public opinion can influence campaign strategies, resource allocation, and even policy decisions. For example, an overreliance on a single, potentially biased poll could lead a campaign to focus on the wrong voter demographics or neglect important issues. In the context of a close election, even small inaccuracies in polling data could have significant consequences.
Therefore, a cautious and critical approach to interpreting available data is essential, acknowledging its limitations and considering multiple sources before drawing any conclusions.
Focusing on Specific Metrics: Reliable Numbers On Trump V Harris Are Scarce For Now
Reliable polling data comparing Trump and Harris on specific policy issues is still emerging, making definitive comparisons challenging. However, by examining hypothetical scenarios based on past voting patterns and policy statements, we can illustrate how different metrics might be used to assess their relative strengths and weaknesses. This analysis focuses on potential data points, acknowledging the inherent limitations of current data scarcity.This section will present hypothetical data comparing Trump and Harris across key policy areas, highlighting potential data sources and the impact of varying methodologies on the final results.
It’s crucial to remember that these are illustrative examples, not definitive conclusions. Future data collection will be necessary to provide a more accurate and comprehensive picture.
Hypothetical Policy Metric Comparison
Area | Trump Metric 1 (Hypothetical Approval Rating) | Harris Metric 1 (Hypothetical Approval Rating) | Metric Description |
---|---|---|---|
Economic Policy | 45% | 52% | Percentage of respondents approving of each candidate’s handling of the economy, based on a hypothetical national poll. |
Foreign Policy | 48% | 42% | Percentage of respondents approving of each candidate’s approach to foreign policy, based on a hypothetical national poll. |
Social Issues | 38% | 58% | Percentage of respondents approving of each candidate’s stance on social issues, based on a hypothetical national poll. |
Potential Data Sources for Hypothetical Metrics
Area | Potential Data Source 1 | Potential Data Source 2 | Methodology Considerations |
---|---|---|---|
Economic Policy | National polls conducted by reputable polling firms (e.g., Gallup, Pew Research Center) | Analysis of economic indicators during their respective times in office (GDP growth, unemployment rates) | Polling methodologies vary; sample size and question wording can significantly influence results. Economic indicators can be interpreted differently depending on the chosen metrics and timeframes. |
Foreign Policy | Expert surveys assessing the effectiveness of each candidate’s foreign policy approaches. | Public opinion polls focusing on specific foreign policy issues (e.g., responses to international crises). | Expert opinions may be subjective; public opinion polls are susceptible to biases related to sample selection and question phrasing. |
Social Issues | National polls on specific social issues (e.g., abortion rights, LGBTQ+ rights). | Analysis of voting patterns on legislation related to social issues. | Poll results can be influenced by question wording and social desirability bias. Legislative voting patterns may not fully reflect public opinion. |
Impact of Differing Methodologies
Different methodologies in data collection can lead to significantly varied results. For example, a poll conducted using a landline-only sample will likely produce different results than one using a representative sample across various demographics and communication methods (landlines, cell phones, online). Similarly, relying solely on economic indicators might paint an incomplete picture of public opinion on economic policy, while focusing exclusively on public opinion polls might overlook the practical implications of policy decisions.
It’s tough getting reliable numbers on the Trump vs. Harris race right now; everything feels so fluid. It makes me think about how similarly murky things are in other areas, like Mexico’s energy policy – check out this article on confused and dirty Claudia Sheinbaum’s energy plan for a perfect example of confusing information. The lack of clarity there mirrors the current uncertainty surrounding the US election numbers.
Analyzing voting patterns on specific legislation can also be problematic, as legislative outcomes are often influenced by factors beyond public opinion, such as party politics and lobbying efforts. A comprehensive analysis would require a triangulation of multiple data sources and methodologies to mitigate biases and produce a more reliable picture.
Visual Representation of Data
Data visualization is crucial for understanding complex information like public opinion polls. Effectively presented visuals can quickly communicate trends and patterns that might be missed in raw numerical data. Let’s explore how we might visualize hypothetical support for Trump and Harris.
Hypothetical Bar Chart of Trump and Harris Support
Imagine a bar chart titled “Hypothetical Presidential Candidate Support: Trump vs. Harris.” The horizontal axis (x-axis) would list the candidates: “Trump” and “Harris.” The vertical axis (y-axis) would represent the percentage of support, ranging from 0% to 100%. Two bars would be displayed, one for each candidate. Let’s assume, for the sake of this example, that the fictional, reliable dataset shows Trump with 48% support and Harris with 52% support.
The bar representing Harris would be taller than the bar representing Trump, clearly showing Harris’s slight lead in this hypothetical scenario. Error bars could be included to represent the margin of error in the polling data.
Hypothetical Map of Regional Support for Trump and Harris
A hypothetical map of the United States could illustrate regional variations in support. The map would use a color-coded scheme to represent the percentage of support for each candidate within each state. For example, shades of red could indicate higher support for Trump, while shades of blue could represent higher support for Harris. A legend would clearly define the color scale and corresponding percentage ranges.
The data source for this map would be a fictional, but reliable, state-level poll conducted using a representative sample size for each state. The map would immediately highlight which regions favored Trump and which favored Harris, offering a geographically nuanced perspective on the election.
Misleading Visual Representations of Data
Visual representations, while powerful, can be easily manipulated to misrepresent data. For instance, a bar chart with a truncated y-axis (not starting at zero) can exaggerate the difference between two values. Similarly, a map with an uneven scale or poorly chosen colors can distort the actual geographical distribution of support. Without clear labels, scales, and a readily understandable legend, visual representations can be highly misleading.
It’s crucial to carefully examine the methodology behind the data visualization and to be aware of potential biases before drawing conclusions. For example, a map using a color scheme that associates red with negative connotations (such as loss) could subconsciously influence viewers’ interpretation of the data even if the color was not specifically chosen to be negative.
Future Data Needs and Collection
The current scarcity of reliable comparative data on Trump and Harris highlights a critical need for more comprehensive data collection strategies. A robust comparison requires moving beyond readily available, often superficial, metrics and delving into deeper, more nuanced data sets that capture the complexities of their political careers and policy impacts. This will require a multi-faceted approach involving diverse data sources and rigorous methodological considerations.The lack of readily available, comparable data on Trump and Harris stems from several factors.
Existing datasets often focus on individual aspects of their careers (e.g., voting records, campaign finance, media coverage) rather than providing a holistic picture. Furthermore, data collection methodologies may vary across different sources, hindering direct comparison. Addressing these limitations requires a concerted effort to gather and harmonize data from multiple sources, ensuring consistency and reliability.
Data Types Necessary for a More Complete Comparison
To achieve a truly comprehensive comparison, future research should prioritize several crucial data types. These include detailed policy positions on key issues (with granular breakdowns of specific proposals and their potential impacts), quantitative measures of policy outcomes (e.g., economic indicators, environmental changes, social progress metrics), qualitative analysis of public discourse (examining media coverage, social media sentiment, and public opinion polls to gauge public perception and impact), and comparative analysis of leadership styles and administrative approaches (including information on personnel appointments, decision-making processes, and management of government resources).
The inclusion of these diverse data types will allow for a richer and more nuanced understanding of the differences and similarities between the two figures.
Potential Data Sources
Gathering the necessary data will require leveraging multiple sources. Governmental archives and websites provide access to official documents, policy proposals, budgetary information, and voting records. Academic research databases offer scholarly articles and studies analyzing various aspects of their careers and policy impacts. News archives and media databases can be used to track media coverage and public opinion.
Finally, social media analytics tools could help quantify public sentiment and engagement with their respective messages. Combining these sources, and potentially supplementing them with surveys and interviews (where ethically permissible and conducted rigorously), would create a powerful foundation for more comprehensive comparative analysis.
Ethical Considerations in Collecting and Using Political Data
The collection and use of political data must adhere to strict ethical guidelines. Privacy concerns are paramount; any data collection must respect individual privacy rights and comply with relevant data protection regulations. Transparency is crucial; the methodology used to collect and analyze data should be clearly documented and made publicly available. Objectivity and avoidance of bias are essential; researchers must strive to present data in a neutral and unbiased manner, avoiding any manipulation or selective reporting that could distort the findings.
Finally, the potential impact of the research should be carefully considered; researchers should be mindful of how their findings might be used and interpreted, and take steps to mitigate any potential for misuse or harm. Data security measures must also be implemented to protect collected data from unauthorized access or breaches.
Ultimately, the scarcity of reliable data on the Trump vs. Harris race underscores a critical need for more robust and transparent data collection practices. While we currently lack definitive numbers, understanding the limitations of existing data and advocating for better methods is the first step towards a more informed electorate. The next election cycle needs to prioritize rigorous data collection to provide citizens with the information they need to make informed choices.