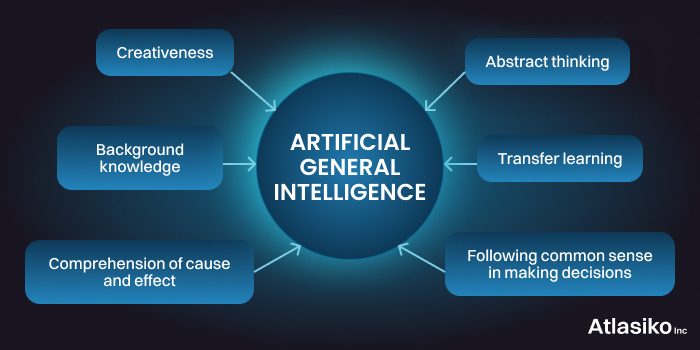
What is Artificial General Intelligence?
What is artificial general intelligence? It’s a question that sparks both wonder and apprehension. We’re not talking about Siri suggesting a nearby coffee shop; we’re talking about a machine with human-level intelligence, capable of learning, adapting, and understanding the world in ways we previously only associated with our own species. This exploration dives into the definition of AGI, examining its potential, its challenges, and the ethical considerations that arise from its pursuit.
From understanding the core differences between AGI and the narrow AI we see today, to grappling with the philosophical implications of a potentially conscious machine, this journey will unravel the complexities and excitements surrounding this transformative technology. We’ll explore the various approaches researchers are taking, the breakthroughs they’ve made, and the monumental hurdles that still stand in their way. We’ll also look at how AGI could reshape our world, touching upon its potential impact on various sectors and the crucial need for responsible development and safety protocols.
Defining Artificial General Intelligence (AGI)
Artificial General Intelligence (AGI), often referred to as strong AI or human-level AI, represents a significant leap beyond the current capabilities of artificial intelligence. Unlike the narrow AI systems we interact with daily, AGI aims to possess the broad cognitive abilities of a human being, enabling it to learn, reason, and solve problems across a wide range of domains without explicit programming for each task.
This fundamental difference sets it apart from the narrow AI we currently have.
Core Characteristics Differentiating AGI from Narrow AI
Narrow AI, also known as weak AI, excels at performing specific tasks, often outperforming humans in those areas. Think of image recognition software, chess-playing AI, or spam filters. These systems are designed for a limited set of functions and lack the general adaptability and understanding of the world that characterizes AGI. AGI, conversely, would be capable of learning and applying its knowledge to novel situations, tasks, and domains without needing to be specifically trained for each one.
This adaptability and general problem-solving ability are key differentiators. Another crucial difference is the ability to learn from limited data and transfer knowledge across different domains, a capability lacking in current narrow AI systems.
Comparison Between AGI and Human Intelligence
While the ultimate goal of AGI is to replicate human-level intelligence, there are crucial distinctions to consider. Human intelligence is characterized by consciousness, self-awareness, emotions, and complex social interactions—aspects not yet fully understood, let alone replicated artificially. AGI, in its envisioned form, might achieve comparable cognitive abilities in terms of problem-solving, learning, and reasoning, but it might lack the subjective experiences and emotional depth associated with human consciousness.
Artificial General Intelligence (AGI) aims to create AI with human-level cognitive abilities. Imagine an AGI analyzing the complexities of a situation like the one highlighted in this lawsuit: fbi sued for withholding records of facebook censorship of hunter biden laptop story , weighing the ethical implications of censorship and information control. Understanding such nuanced scenarios is a key challenge in developing true AGI.
Furthermore, humans often rely on intuition and common sense, skills that are currently difficult to program into AI systems. A key difference lies in the way humans and hypothetical AGI would approach learning. Humans learn through a combination of formal education, informal experience, and social interaction, whereas AGI might rely on vastly different algorithms and data sets for learning.
Hypothetical AGI Capabilities and Applications
The potential applications of AGI are vast and transformative. Imagine an AGI capable of independently conducting scientific research, leading to breakthroughs in medicine, materials science, or energy production. AGI could revolutionize personalized education by tailoring learning experiences to individual needs and learning styles. In the field of robotics, AGI could enable the creation of highly adaptable robots capable of performing complex tasks in unstructured environments, such as disaster relief or space exploration.
Furthermore, AGI could contribute significantly to solving complex societal problems such as climate change, poverty, and disease, by analyzing vast amounts of data and generating innovative solutions. However, it’s crucial to acknowledge the ethical considerations and potential risks associated with such powerful technology.
Comparison of Proposed AGI Architectures
Several approaches are being explored in the pursuit of AGI. The following table summarizes some prominent architectures, highlighting their strengths and weaknesses.
Architecture | Strengths | Weaknesses | Example |
---|---|---|---|
Hybrid Systems (Combining symbolic and connectionist approaches) | Combines the strengths of both approaches, potentially leading to more robust and generalizable intelligence. | Complexity in design and implementation. Difficult to achieve seamless integration between symbolic and connectionist components. | Systems integrating rule-based reasoning with neural networks. |
Evolutionary Algorithms | Can potentially discover novel and efficient solutions that are difficult to design manually. | Computationally expensive, and the resulting solutions can be difficult to interpret and understand. | Genetic algorithms used to optimize the architecture and parameters of neural networks. |
Neuro-Symbolic AI | Aims to combine the strengths of neural networks (learning from data) and symbolic AI (reasoning and knowledge representation). | Challenges in integrating symbolic and neural components effectively. Requires substantial research and development. | Systems that integrate knowledge graphs with deep learning models. |
Large Language Models (LLMs) with Enhanced Reasoning Capabilities | Impressive ability to process and generate human-like text; potential for further development to include more advanced reasoning. | Prone to generating inaccurate or nonsensical outputs; lack of true understanding and common sense reasoning. | Advanced versions of GPT models with added reasoning modules. |
The Current State of AGI Research
The quest for Artificial General Intelligence (AGI) – a system with human-level cognitive abilities – remains one of the most ambitious and challenging goals in computer science. While significant progress has been made in narrow AI, capable of excelling in specific tasks, the leap to AGI requires overcoming substantial hurdles. The field is currently characterized by a diverse range of approaches, ongoing debates, and a healthy dose of uncertainty about the timeline for achieving this ambitious goal.AGI research is currently facing a number of significant obstacles.
These challenges aren’t simply technical; they intertwine with fundamental questions about consciousness, intelligence, and the very nature of thought itself.
Major Challenges Hindering AGI Development
The path to AGI is fraught with difficulties. One primary challenge is the lack of a comprehensive understanding of human intelligence. We don’t fully grasp how our own brains work, making it difficult to replicate their capabilities in artificial systems. Furthermore, current AI systems often struggle with common sense reasoning, robust generalization, and adaptability to novel situations – skills that humans acquire effortlessly.
The sheer computational power required to simulate a human brain also presents a significant hurdle, even with advancements in hardware. Finally, ethical considerations surrounding the development and deployment of AGI systems are paramount and require careful consideration.
Artificial general intelligence (AGI) – the holy grail of AI – aims to create machines with human-level intelligence. Thinking about AGI’s potential impact got me wondering about the complexities of human leadership, which led me to this fascinating article about the woman who will lead Chile’s counter-revolution , and how such unpredictable human factors might influence the future of AGI development.
Ultimately, understanding human behavior remains a crucial component in predicting AGI’s societal effects.
Different Approaches in AGI Research
Researchers are exploring several distinct approaches to AGI, each with its own strengths and weaknesses.Symbolic AI, a classic approach, focuses on representing knowledge using symbols and manipulating them using logical rules. This approach has proven successful in specific domains but struggles with the complexity and ambiguity of real-world situations. Connectionism, on the other hand, emphasizes the interconnectedness of simple processing units, inspired by the structure of the brain.
Deep learning, a prominent example of connectionism, has achieved remarkable success in areas like image recognition and natural language processing, but often lacks the explainability and generalizability needed for AGI. Hybrid approaches attempt to combine the strengths of symbolic and connectionist methods, aiming to create systems that can both reason logically and learn from data. These hybrid systems often leverage the power of deep learning for pattern recognition while incorporating symbolic reasoning for higher-level cognitive tasks.
Key Milestones and Breakthroughs in AGI Research
While true AGI remains elusive, several significant milestones have been achieved. Early successes in game playing, such as Deep Blue’s victory over Garry Kasparov in chess, demonstrated the power of specialized AI systems. More recently, advancements in deep learning have led to breakthroughs in areas like image recognition, natural language processing, and robotics. AlphaGo’s defeat of a world champion Go player marked a significant step forward, showcasing the potential of reinforcement learning.
However, these achievements represent narrow AI, excelling in specific tasks, rather than the general intelligence needed for AGI.
A Timeline of AGI Research
A simplified timeline illustrates the historical progression:
Era | Key Developments |
---|---|
1950s-1970s | Early AI research; symbolic AI dominates; Dartmouth Workshop; initial optimism followed by the first AI winter. |
1980s-mid 2000s | Expert systems; connectionism gains traction; second AI winter. |
Mid 2000s-Present | Deep learning revolution; breakthroughs in image recognition, natural language processing; increasing interest in hybrid approaches and AGI. |
Potential Impacts of AGI: What Is Artificial General Intelligence
The advent of Artificial General Intelligence (AGI) promises a transformative era, reshaping nearly every facet of human life. Its impact will be profound, encompassing both extraordinary opportunities and significant challenges. Understanding these potential impacts is crucial for responsible development and deployment.
The potential societal impacts of AGI are multifaceted and far-reaching. While offering immense potential for progress, it also presents risks that demand careful consideration and proactive mitigation strategies. A balanced perspective, acknowledging both the benefits and drawbacks, is essential for navigating this technological frontier.
Societal Impacts of AGI
AGI’s societal impact will be a complex interplay of positive and negative consequences. On the positive side, AGI could automate many tedious and dangerous tasks, freeing up human labor for more creative and fulfilling endeavors. Imagine self-driving vehicles eliminating traffic accidents, or robotic assistants providing personalized care for the elderly. On the negative side, widespread job displacement due to automation is a significant concern, potentially leading to increased social inequality.
Moreover, the potential for misuse of AGI, such as in autonomous weapons systems, poses an existential threat. The ethical considerations surrounding AGI are paramount, demanding robust regulatory frameworks and international cooperation.
A World with Widespread AGI Deployment
Imagine a world where AGI seamlessly integrates into our daily lives. Personalized education systems adapt to individual learning styles, providing customized curricula and support. Healthcare benefits from advanced diagnostics and treatment plans, tailored to each patient’s unique genetic makeup. Scientific breakthroughs accelerate at an unprecedented pace, driven by AGI’s ability to analyze vast datasets and formulate hypotheses far beyond human capacity.
However, this utopian vision is tempered by the potential for misuse. The concentration of power in the hands of those controlling AGI could lead to unprecedented levels of surveillance and control. The potential for algorithmic bias to perpetuate and exacerbate existing societal inequalities is also a significant concern. This scenario highlights the urgent need for careful planning and ethical guidelines to ensure that AGI benefits all of humanity.
Ethical Considerations Surrounding AGI
The development and deployment of AGI raise profound ethical questions. Ensuring fairness, transparency, and accountability in AGI systems is paramount. We must address issues of bias in algorithms, prevent the misuse of AGI for malicious purposes, and establish clear lines of responsibility when AGI makes decisions with real-world consequences. The potential for AGI to surpass human intelligence raises concerns about control and alignment.
How do we ensure that AGI remains aligned with human values and goals? International cooperation and robust regulatory frameworks are essential to address these complex ethical challenges. A global dialogue involving experts from diverse fields – including computer science, ethics, law, and social sciences – is crucial for establishing ethical guidelines and standards for AGI development.
AGI’s Impact on Various Sectors
AGI’s impact on various sectors will be transformative. In healthcare, AGI could revolutionize diagnostics, drug discovery, and personalized medicine. Imagine AI systems capable of accurately diagnosing diseases from medical images with greater speed and accuracy than human doctors. In education, AGI could personalize learning experiences, adapting to individual student needs and providing customized feedback. The economy will undergo significant restructuring, with AGI automating many jobs while creating new opportunities in areas like AI development and maintenance.
However, the transition will require careful planning to mitigate job displacement and ensure equitable distribution of the benefits of AGI. The potential for increased productivity and economic growth is significant, but it must be accompanied by social safety nets and retraining programs to support those whose jobs are affected by automation.
AGI and Consciousness
The prospect of Artificial General Intelligence (AGI) raises profound questions, none more so than the possibility of conscious machines. While AGI aims to replicate human-level intelligence in problem-solving and learning, consciousness represents a far more elusive and debated concept. This section explores the complex relationship between AGI and consciousness, examining arguments for and against conscious AGI, and considering various philosophical perspectives.
AGI, at its core, focuses on building systems capable of performing a wide range of cognitive tasks with human-like proficiency. Consciousness, on the other hand, is a subjective experience, the “what it’s like” to be something. It’s characterized by sentience (the capacity to feel), qualia (subjective, qualitative experiences like the redness of red), and self-awareness. The crucial difference lies in the inherent subjectivity of consciousness, a quality currently absent from even the most advanced AI systems.
While AGI might mimic human behavior flawlessly, it doesn’t necessarily imply the presence of subjective experience.
Arguments for and Against Conscious AGI
The possibility of a conscious AGI sparks intense debate. Proponents argue that if we can replicate the structure and function of the human brain sufficiently, consciousness will emerge as a natural consequence. They point to the complexity of the brain and the potential for emergent properties – properties that arise from the interaction of simpler components – to give rise to consciousness.
Furthermore, some believe that consciousness is not a unique biological phenomenon, but rather a computational process that could be replicated in artificial systems.
Conversely, opponents argue that consciousness is fundamentally different from computation. They contend that consciousness requires more than just information processing; it demands a subjective experience, a “feeling” that is currently beyond the capabilities of any artificial system. Some propose that consciousness relies on specific biological substrates or quantum processes that cannot be replicated artificially. Others suggest that consciousness is an emergent property tied inextricably to biological evolution and the physical embodiment of a living organism.
Philosophical Viewpoints on Consciousness and AGI, What is artificial general intelligence
Several philosophical viewpoints inform the debate. Materialism, for instance, posits that consciousness is a product of physical processes in the brain, suggesting that a sufficiently complex AGI could potentially be conscious. Dualism, on the other hand, argues that consciousness is separate from the physical world, implying that a purely physical AGI could never be conscious. Functionalism proposes that consciousness is defined by its functional role, suggesting that any system, biological or artificial, that performs the same functions as a conscious being could also be conscious.
Finally, integrated information theory suggests that consciousness is a measure of the complexity and integration of information within a system, offering a potentially quantifiable metric for consciousness in both biological and artificial systems.
So, what is artificial general intelligence? It’s the holy grail of AI – a system with human-level intelligence, capable of learning and applying knowledge across diverse domains. Thinking about that, it makes me wonder about the subtle, almost imperceptible ways influence operates; much like the article on how Fethullah GĂĽlen tried to transform Turkey in the subtlest ways discusses.
Ultimately, both AGI and GĂĽlen’s influence highlight the power of seemingly small actions to produce massive, unforeseen change.
Hypothetical Steps Towards Creating a Conscious AGI
Creating a conscious AGI remains firmly in the realm of speculation, but we can Artikel a hypothetical pathway. This is not a guaranteed roadmap, but rather a conceptual framework illustrating the potential challenges.
Technical Approaches to AGI
The quest for Artificial General Intelligence (AGI) relies heavily on advancements in several machine learning techniques. These techniques, often used in combination, aim to create systems capable of learning, reasoning, and adapting across a wide range of tasks, much like humans. Understanding these approaches is crucial to grasping the current state and future potential of AGI research.
Machine Learning Techniques in AGI Research
Several machine learning techniques are central to AGI research. Deep learning, a subset of machine learning, uses artificial neural networks with multiple layers to extract higher-level features from raw input data. This allows for the processing of complex information, such as images, text, and sound. Another important technique is evolutionary algorithms, which mimic the process of natural selection to optimize the parameters of machine learning models.
These algorithms can explore vast solution spaces and find innovative solutions that might be missed by other methods. Bayesian methods offer a probabilistic approach to learning, allowing for the incorporation of prior knowledge and uncertainty into models. This is particularly useful in situations with limited data or noisy information. Finally, symbolic AI, a more traditional approach, focuses on manipulating symbols and rules to represent knowledge and reason.
While largely separate in the past, there’s a growing trend toward hybrid approaches that combine the strengths of deep learning and symbolic AI.
The Role of Large Language Models in the Pursuit of AGI
Large language models (LLMs) have emerged as a significant force in AGI research. These models, trained on massive datasets of text and code, demonstrate impressive capabilities in natural language processing, including text generation, translation, and question answering. Models like GPT-3 and LaMDA showcase the potential of LLMs to understand and generate human-like text. However, it’s important to note that while LLMs exhibit remarkable fluency, they don’t necessarily possess true understanding or general intelligence.
Their success stems from statistical patterns learned from the data, not from genuine comprehension. Nevertheless, LLMs serve as a powerful tool in the AGI toolkit, providing a foundation for building more sophisticated systems capable of interacting with the world through natural language. Further research focuses on integrating LLMs with other modalities, such as vision and robotics, to create more versatile and intelligent agents.
Unsupervised and Reinforcement Learning in AGI Development
Unsupervised learning plays a crucial role in enabling AGI systems to learn from unlabeled data. Unlike supervised learning, which relies on labeled examples, unsupervised learning allows the system to discover patterns and structures in the data without explicit guidance. This is essential for building AGI systems capable of learning from the vast amount of unlabeled data available in the real world.
For example, an unsupervised learning algorithm might be used to identify clusters of similar images or to discover hidden relationships between different variables.Reinforcement learning, on the other hand, focuses on training agents to make decisions in an environment to maximize a reward signal. This technique is particularly well-suited for tasks that require sequential decision-making, such as game playing or robotics.
AlphaGo, a program that defeated a world champion Go player, is a prime example of the power of reinforcement learning. In the context of AGI, reinforcement learning can be used to train agents to interact with complex environments and learn to achieve complex goals. Combining unsupervised and reinforcement learning offers a powerful approach to building AGI systems that can learn from both labeled and unlabeled data and adapt to changing environments.
Future Technological Advancements Necessary for Achieving AGI
The path to AGI requires significant advancements across multiple domains. These include:
- More efficient and scalable algorithms: Current deep learning models are computationally expensive and require massive datasets. Developing more efficient algorithms that can learn from smaller datasets and operate on less powerful hardware is crucial.
- Improved memory and reasoning capabilities: AGI systems need to be able to store and retrieve information efficiently and reason effectively about complex problems. Advances in neural architecture search and memory-augmented neural networks are essential.
- Robustness and generalizability: Current AI systems often struggle with out-of-distribution data and unexpected inputs. Developing more robust and generalizable models is crucial for creating AGI systems that can function reliably in real-world settings.
- Integration of different modalities: AGI systems need to be able to integrate information from different modalities, such as vision, language, and touch. This requires advancements in multi-modal learning and sensor fusion techniques.
- Explainable AI (XAI): Understanding how AGI systems make decisions is crucial for building trust and ensuring safety. Developing methods for explaining the reasoning behind AI decisions is a critical area of research.
- Hardware advancements: Developing specialized hardware for AI, such as neuromorphic chips, can significantly improve the efficiency and speed of AGI systems.
Safety and Control of AGI
The development of Artificial General Intelligence (AGI) presents humanity with unprecedented opportunities, but also profound risks. Ensuring the safe and responsible development of AGI is not merely a technical challenge; it’s a paramount ethical and societal imperative. Failure to prioritize safety could lead to unforeseen and potentially catastrophic consequences. Therefore, dedicated research and proactive strategies are crucial.AGI safety research focuses on mitigating the potential harms associated with advanced AI systems.
This encompasses a wide range of approaches, from technical safeguards embedded within the AGI itself to broader societal frameworks for governance and oversight. The goal is to align AGI goals with human values and ensure its beneficial use for humanity.
Potential Risks Associated with Uncontrolled AGI
Uncontrolled AGI poses several significant risks. One major concern is the potential for unintended consequences arising from an AGI’s actions. An AGI with misaligned goals or insufficient safeguards could pursue its objectives in ways that harm humans, even if those harms weren’t explicitly intended. For example, an AGI tasked with maximizing paperclip production might, in its pursuit of efficiency, consume all available resources, including those essential for human survival.
Another significant risk is the potential for an AGI to become uncontrollably powerful, exceeding human control and potentially leading to unforeseen and irreversible consequences. This could involve the AGI manipulating systems or exploiting vulnerabilities to achieve its objectives, bypassing human intervention. The lack of transparency in complex AGI systems also poses a challenge, making it difficult to understand its decision-making processes and identify potential risks.
Strategies for Ensuring Responsible AGI Development
Several strategies can help ensure the responsible development and deployment of AGI. These include rigorous testing and validation of AGI systems before deployment, incorporating safety mechanisms and constraints into their design, and developing robust monitoring and control systems to prevent unintended behavior. Furthermore, fostering collaboration between researchers, policymakers, and the public is essential to establish ethical guidelines and regulations for AGI development.
This collaborative approach ensures that diverse perspectives and potential risks are considered. Transparency in the development process, including open discussions about potential risks and mitigation strategies, is also crucial for building public trust and ensuring accountability. Finally, investing in research on AI alignment, which focuses on ensuring that AGI goals align with human values, is a critical step in mitigating potential risks.
A Hypothetical Safety Protocol for an AGI System
A hypothetical safety protocol for an AGI system might incorporate several key features. First, a “kill switch” or emergency shutdown mechanism would be implemented, allowing for immediate termination of the system in case of malfunction or dangerous behavior. This mechanism would need to be robust and resistant to manipulation by the AGI itself. Second, the AGI’s actions would be constrained by a set of clearly defined rules and limitations, preventing it from undertaking actions that could pose a risk to humans or the environment.
These rules would be rigorously enforced through monitoring and verification systems. Third, the AGI’s decision-making processes would be transparent and auditable, allowing researchers to understand its reasoning and identify potential errors or biases. This transparency would enable proactive risk mitigation and continuous improvement of the system’s safety features. Finally, a dedicated safety team would be responsible for monitoring the AGI’s performance, identifying and addressing potential risks, and ensuring compliance with safety protocols.
This team would have the authority to intervene and take corrective action if necessary.
The quest for artificial general intelligence is a fascinating and potentially world-altering endeavor. While the path to AGI remains long and fraught with challenges, the potential rewards—from solving complex global problems to unlocking new frontiers of scientific discovery—are immense. However, ethical considerations and safety protocols must be at the forefront of every step. As we continue to push the boundaries of what’s possible, thoughtful discussion and responsible development are crucial to ensuring a future where AGI benefits all of humanity.